Low-Rank And Joint-Sparse Signal Recovery For Spatially And Temporally Correlated Data Using Sparse Bayesian Learning
2018 IEEE INTERNATIONAL CONFERENCE ON ACOUSTICS, SPEECH AND SIGNAL PROCESSING (ICASSP)(2018)
摘要
In order to meet the demands of data-intensive continuous monitoring in wireless body area network, we address a structured sparse signal recovery method to exploit both spatial and temporal correlations in data using compressive sensing (CS). Using a simultaneously low-rank and jointsparse (L&S) signal model, we employ a Bayesian learning treatment by incorporating an L&S-inducing prior over the data and the appropriate hyperpriors over all hyperparameters, resulting in effective reconstruction of the L&S data. Simulation results suggest that the proposed L&S-bSBL is superior to the state-of-the-art recovery methods in terms of computation burden and runtime cost.
更多查看译文
关键词
Wireless Body Area Network, Bayesian Learning, Compressive Sensing, Low-rank and Joint-sparse
AI 理解论文
溯源树
样例
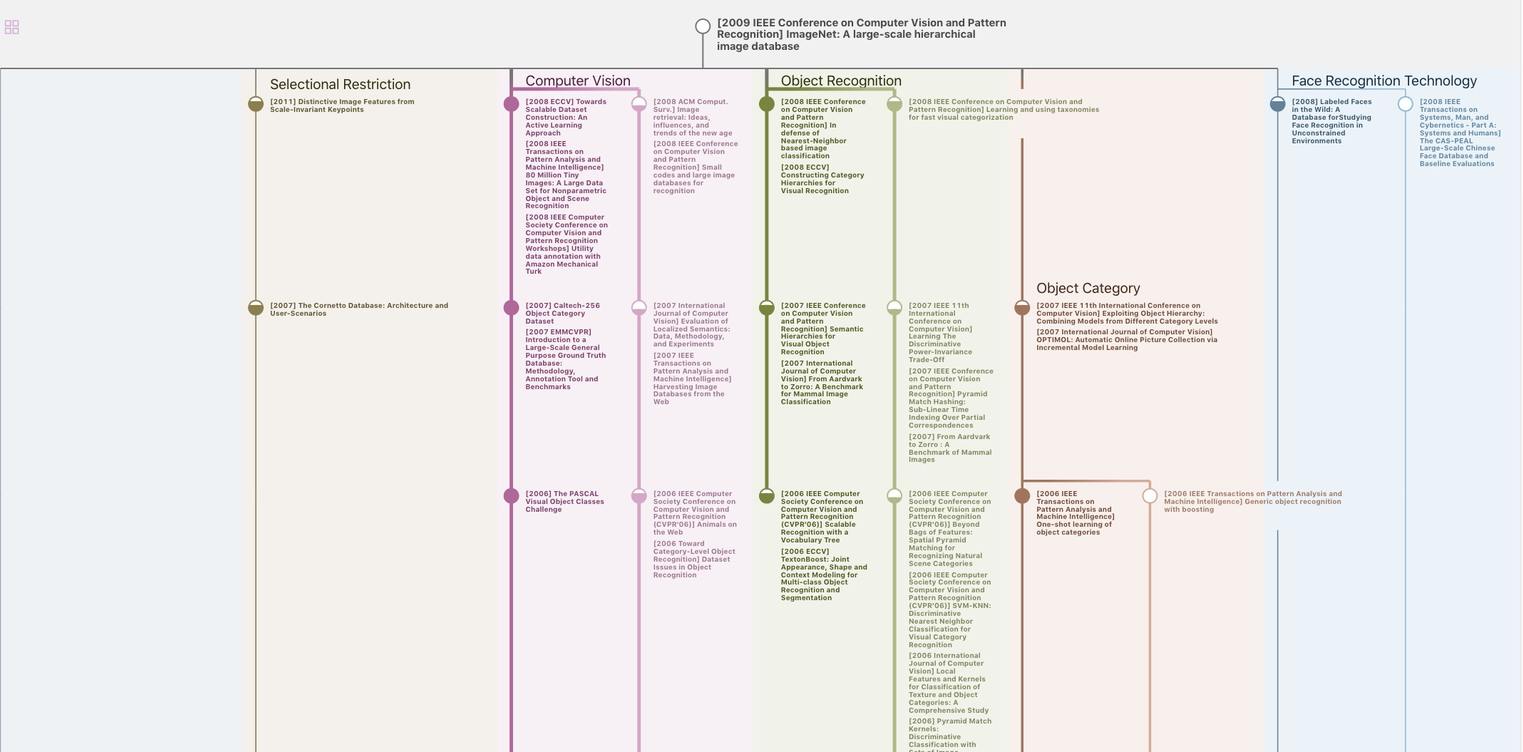
生成溯源树,研究论文发展脉络
Chat Paper
正在生成论文摘要