Deep Feature Embedding Learning For Person Re-Identification Using Lifted Structured Loss
2018 IEEE INTERNATIONAL CONFERENCE ON ACOUSTICS, SPEECH AND SIGNAL PROCESSING (ICASSP)(2018)
摘要
In this paper, we propose deep feature embedding learning for person re-identification (re-id) using lifted structured loss. Although triplet loss has been commonly used in deep neural networks for person re-id, the triplet loss-based framework is not effective in fully using the batch information. Thus, it needs to choose hard negative samples manually that is very time-consuming. To address this problem, we adopt lifted structured loss for deep neural networks that makes the network learn better feature embedding by minimizing intra-class variation and maximizing inter-class variation. Extensive experiments on CUHK03, CUHK01 and VIPeR datasets demonstrate the superior performance of the proposed method over state-of-the-arts in terms of the cumulative match curve (CMC) metric.
更多查看译文
关键词
Person re-identification, convolutional neural networks, deep learning, lifted structured loss, triplet loss
AI 理解论文
溯源树
样例
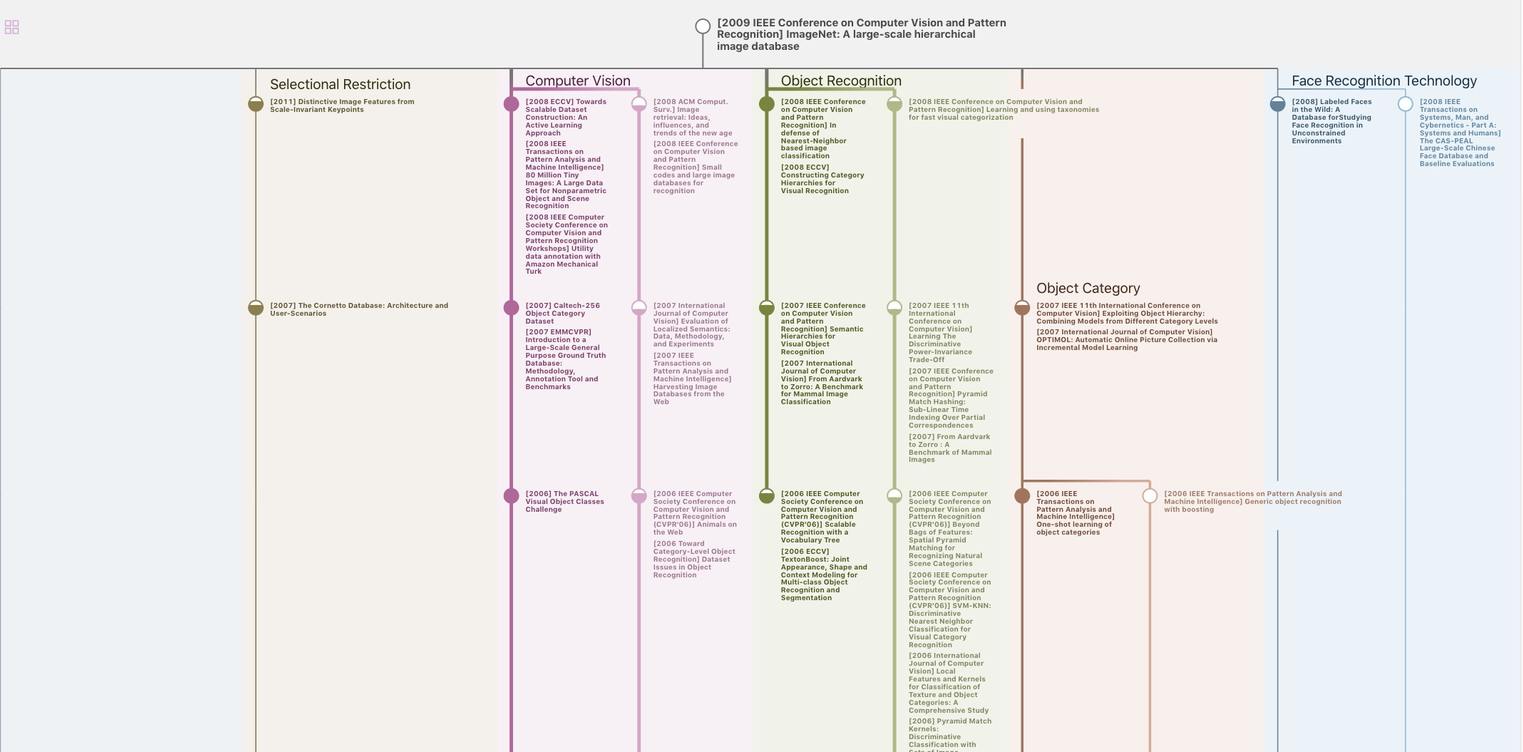
生成溯源树,研究论文发展脉络
Chat Paper
正在生成论文摘要