Exploring sequential characteristics in speaker bottleneck feature for text-dependent speaker verification
2018 IEEE INTERNATIONAL CONFERENCE ON ACOUSTICS, SPEECH AND SIGNAL PROCESSING (ICASSP)(2018)
摘要
In this paper, given the speaker bottleneck feature vectors extracted with speaker discriminant neural networks, we focus on using the sequential speaker characteristics for text-dependent speaker verification. In each evaluation trial, speaker supervectors are used as the representations of the sequential speaker characteristics rendered in the compared speech utterances. To this end, dynamic time warping is used to warp the variable-length speaker feature vector sequences of the utterances to the same length. Thereafter for every utterance, a speaker supervector can be obtained as the concatenation of its speaker feature vectors. We use Euclidean distance and support vector machine (SVM) to compute the decision score on the speaker supervectors. Our experiments on a Microsoft internal keyword-spotting database showed the effectiveness of the proposed speaker supervector for text-dependent speaker verification. Moreover, when SVM backend was used in scoring, the speaker supervector achieved the best EER performance 1.627%, better than the combination of i-vector and probabilistic linear discriminant analysis.
更多查看译文
关键词
Text-dependent speaker verification,sequential speaker characteristics,speaker supervector,dynamic time warping
AI 理解论文
溯源树
样例
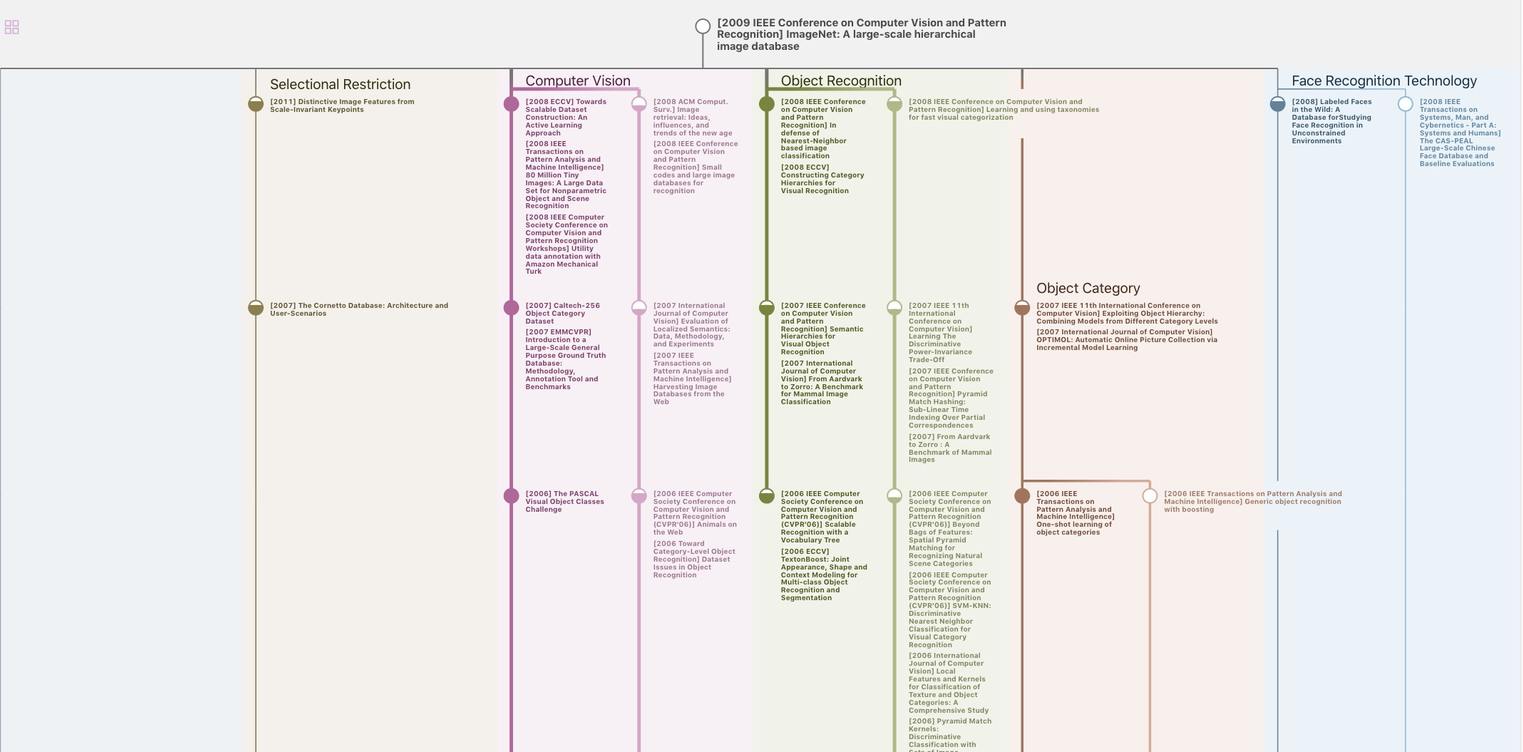
生成溯源树,研究论文发展脉络
Chat Paper
正在生成论文摘要