Siamese Recurrent Auto-Encoder Representation For Query-By-Example Spoken Term Detection
19TH ANNUAL CONFERENCE OF THE INTERNATIONAL SPEECH COMMUNICATION ASSOCIATION (INTERSPEECH 2018), VOLS 1-6: SPEECH RESEARCH FOR EMERGING MARKETS IN MULTILINGUAL SOCIETIES(2018)
摘要
With the explosive development of human-computer speech interaction, spoken term detection is widely required and has attracted increasing interest. In this paper, we propose a weak supervised approach using Siamese recurrent auto-encoder (RAE) to represent speech segments for query-by-example spoken term detection (QbyE-STD). The proposed approach exploits word pairs that contain different instances of the same/different word content as input to train the Siamese RAE. The encoder last hidden state vector of Siamese RAE is used as the feature for QbyE-STD, which is a fixed dimensional embedding feature containing mostly semantic content related information. The advantages of the proposed approach are: 1) extracting more compact feature with fixed dimension while keeping the semantic information for STD; 2) the extracted feature can describe the sequential phonetic structure of similar sounds to degree, which can be applied for zero-resource QbyESTD. Evaluations on real scene Chinese speech interaction data and TIMIT confirm the effectiveness and efficiency of the proposed approach compared to the conventional ones.
更多查看译文
关键词
Siamese recurrent auto-encoder, query-by example spoken term detection, zero-resource
AI 理解论文
溯源树
样例
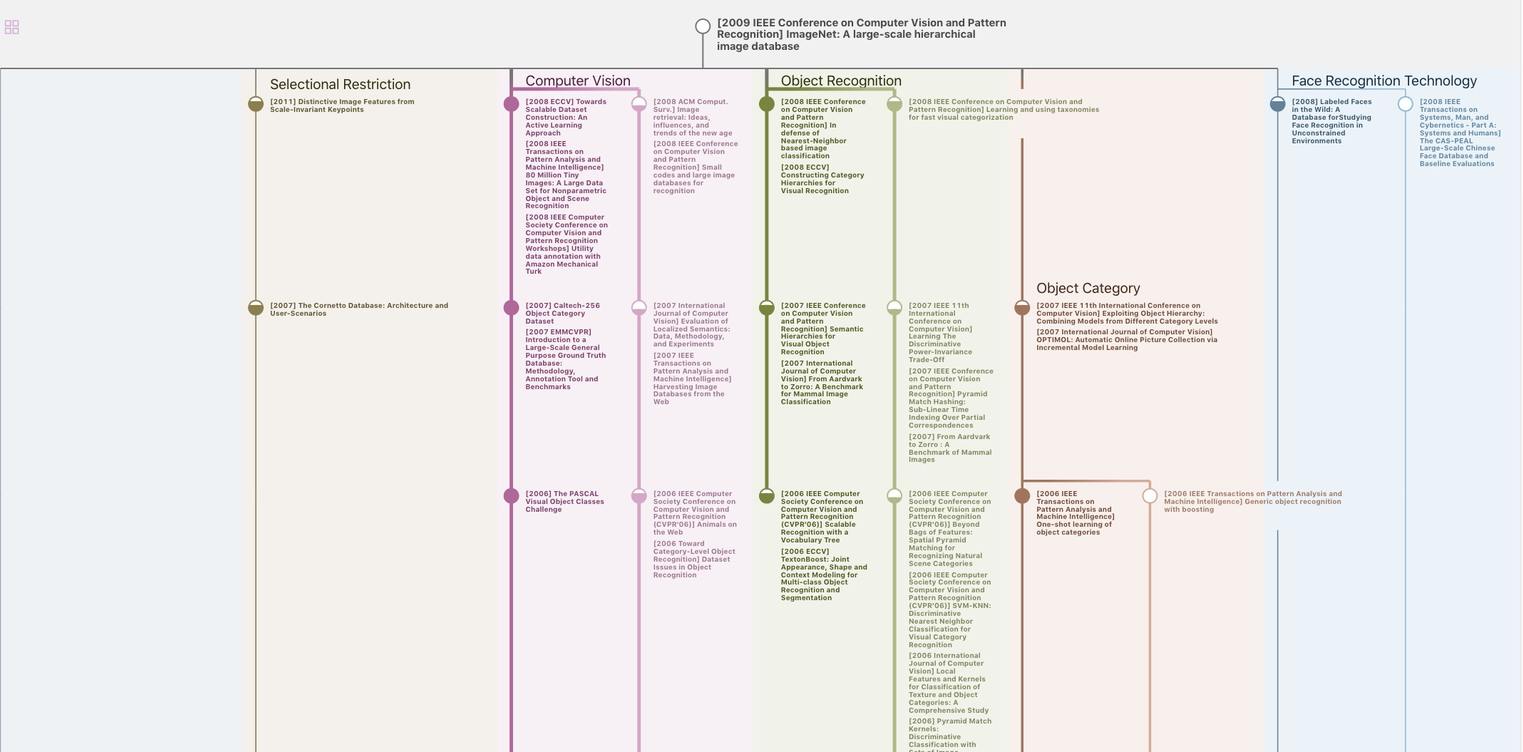
生成溯源树,研究论文发展脉络
Chat Paper
正在生成论文摘要