Encoder Transfer For Attention-Based Acoustic-To-Word Speech Recognition
19TH ANNUAL CONFERENCE OF THE INTERNATIONAL SPEECH COMMUNICATION ASSOCIATION (INTERSPEECH 2018), VOLS 1-6: SPEECH RESEARCH FOR EMERGING MARKETS IN MULTILINGUAL SOCIETIES(2018)
摘要
Acoustic-to-word speech recognition based on attention-based encoder-decoder models achieves better accuracies with much lower latency than the conventional speech recognition systems. However, acoustic-to-word models require a very large amount of training data and it is difficult to prepare one for a new domain such as elderly speech. To address the problem, we propose domain adaptation based on transfer learning with layer freezing. Layer freezing first pre-trains a network with the source domain data, and then a part of parameters is re-trained for the target domain while the rest is fixed. In the attention based acoustic-to-word model, the encoder part is frozen to maintain the generality, and only the decoder part is re-trained to adapt to the target domain. This substantially allows for adaptation of the latent linguistic capability of the decoder to the target domain. Using a large-scale Japanese spontaneous speech corpus as source, the proposed method is applied to three target domains: a call center task and two voice search tasks by adults and by elderly. The models trained with the proposed method achieved better accuracy than the baseline models, which are trained from scratch or entirely re-trained with the target domain.
更多查看译文
关键词
End-to-end speech recognition, Attention-based encoder-decoder model, Adaptation, Transfer learning
AI 理解论文
溯源树
样例
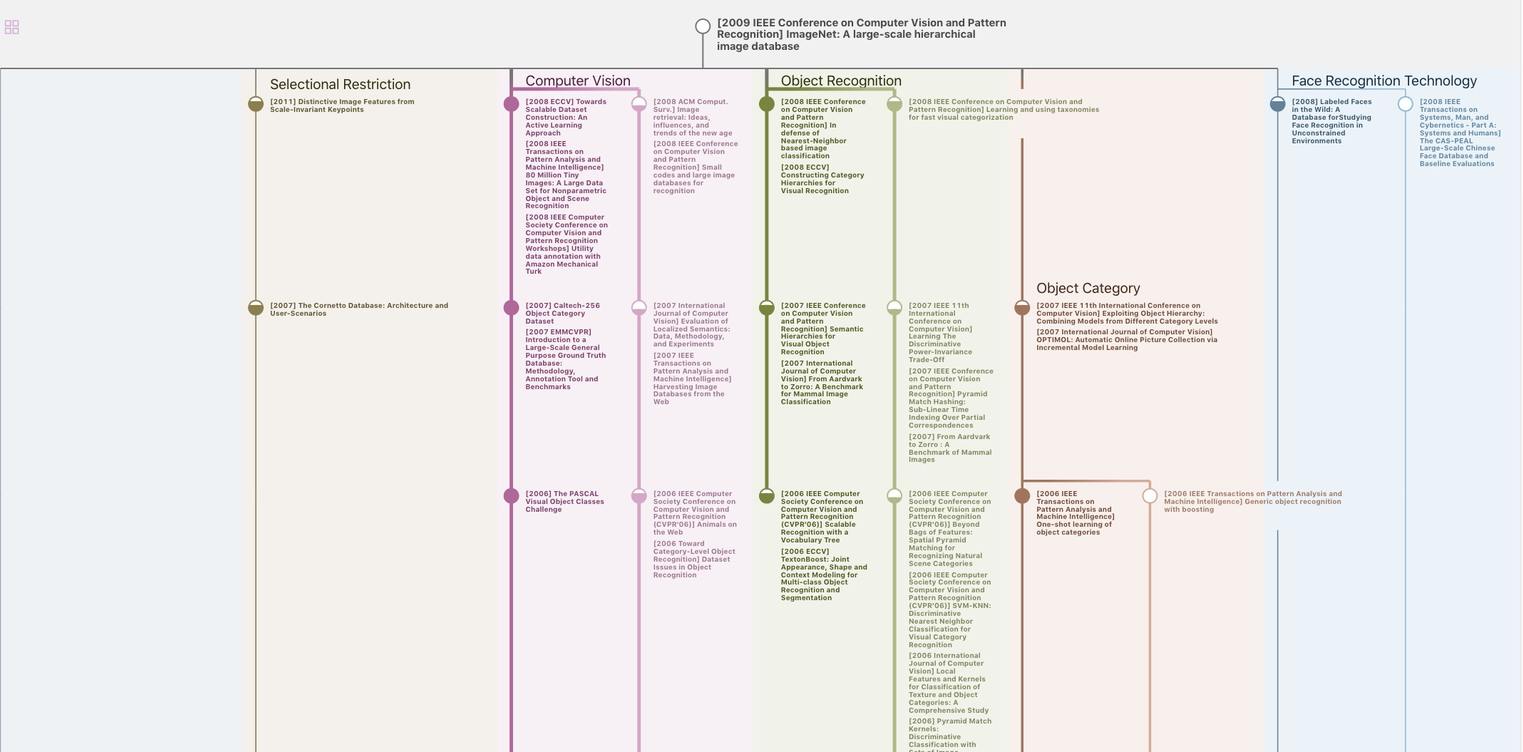
生成溯源树,研究论文发展脉络
Chat Paper
正在生成论文摘要