A Knowledge Driven Structural Segmentation Approach For Play-Talk Classification During Autism Assessment
19TH ANNUAL CONFERENCE OF THE INTERNATIONAL SPEECH COMMUNICATION ASSOCIATION (INTERSPEECH 2018), VOLS 1-6: SPEECH RESEARCH FOR EMERGING MARKETS IN MULTILINGUAL SOCIETIES(2018)
摘要
Automatically segmenting conversational audio into semantically relevant components has both computational and analytical significance. In this paper, we segment play activities and conversational portions interspersed during clinically administered interactions between a psychologist and a child with autism spectrum disorder (ASD). We show that various acoustic-prosodic and turn-taking features commonly used in the literature differ between these segments, and hence can possibly influence further inference tasks. We adopt a two-step approach for the segmentation problem by taking advantage of the structural relation between the two segments. First, we use a supervised machine learning algorithm to estimate class posteriors at frame-level. Next, we use an explicit-duration hidden Markov model (EDHIVIM) to align the states using the posteriors from the previous step. The durational distributions for both play and talk regions are learnt from training data and modeled using the EDHMM. Our results show that speech features can be used to successfully discriminate between play and talk activities, each providing important insights into the child's condition.
更多查看译文
关键词
Autism spectrum disorder, audio segmentation, explicit-duration hidden markov models
AI 理解论文
溯源树
样例
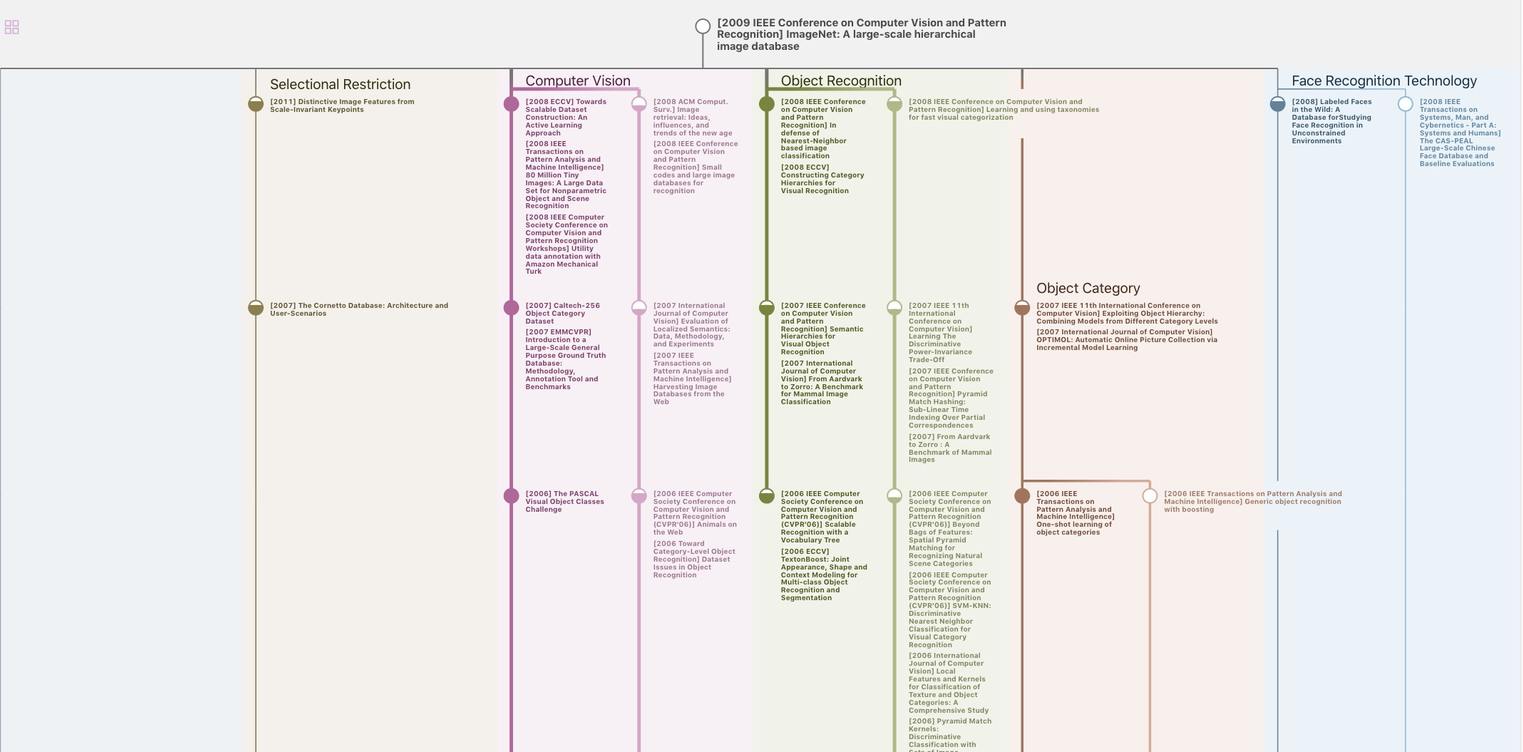
生成溯源树,研究论文发展脉络
Chat Paper
正在生成论文摘要