Domain Adaptation Using Factorized Hidden Layer For Robust Automatic Speech Recognition
19TH ANNUAL CONFERENCE OF THE INTERNATIONAL SPEECH COMMUNICATION ASSOCIATION (INTERSPEECH 2018), VOLS 1-6: SPEECH RESEARCH FOR EMERGING MARKETS IN MULTILINGUAL SOCIETIES(2018)
Abstract
Domain robustness is a challenging problem for automatic speech recognition (ASR). In this paper, we consider speech data collected for different applications as separate domains and investigate the robustness of acoustic models trained on multi domain data on unseen domains. Specifically, we use Factorized Hidden Layer (FHL) as a compact low-rank representation to adapt a multi-domain ASR system to unseen domains. Experimental results on two unseen domains show that FHL is a more effective adaptation method compared to selectively fine-tuning part of the network, without dramatically increasing the model parameters. Furthermore, we found that using singular value decomposition to initialize the low-rank bases of an FHL model leads to a faster convergence and improved performance.
MoreTranslated text
Key words
speech recognition, domain adaptation, factorized hidden layer
AI Read Science
Must-Reading Tree
Example
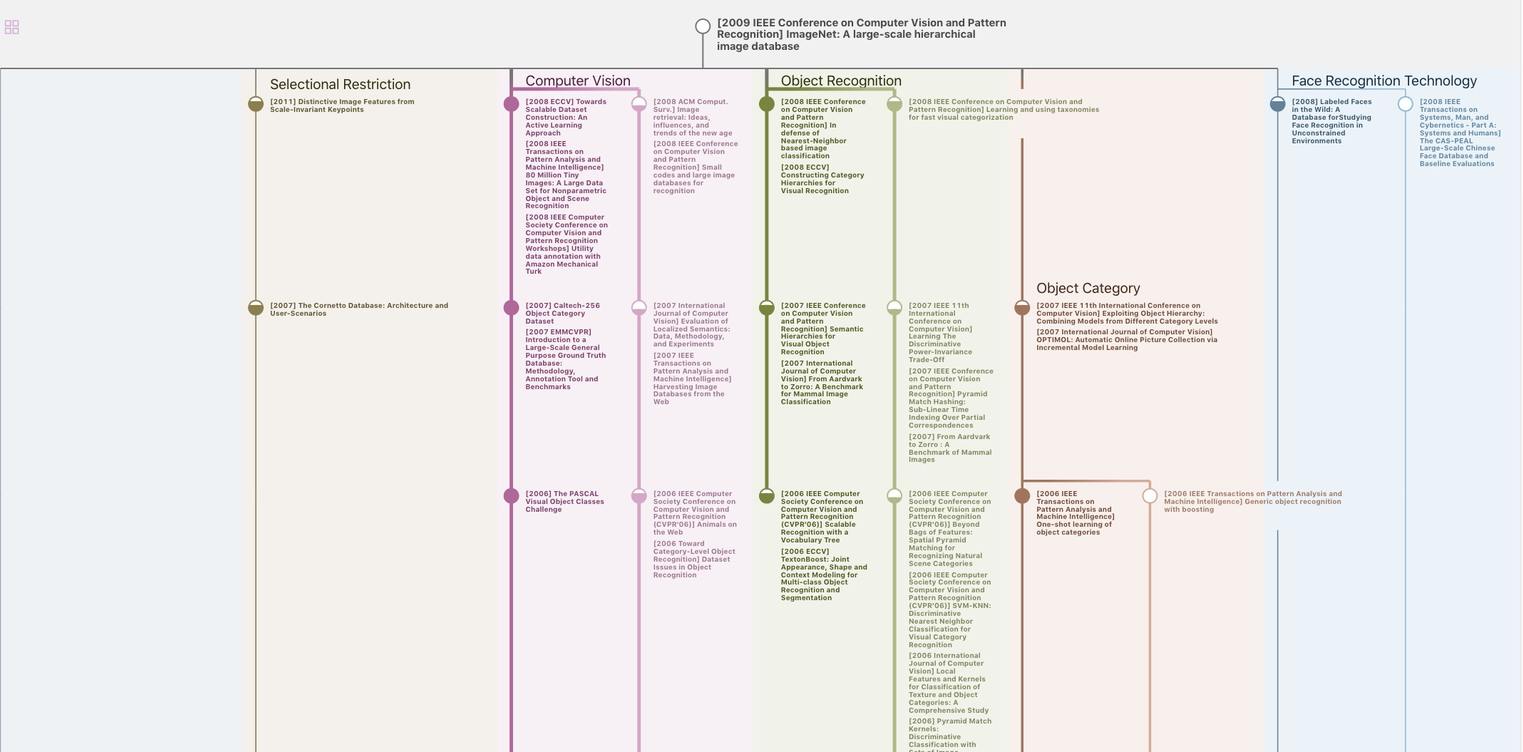
Generate MRT to find the research sequence of this paper
Chat Paper
Summary is being generated by the instructions you defined