Conditional Compatibility Branch And Bound For Feature Cloud Matching
2018 IEEE INTERNATIONAL CONFERENCE ON ROBOTICS AND AUTOMATION (ICRA)(2018)
摘要
In this paper, we consider the problem of data association in feature cloud matching. While Joint Compatibility (JC) test is a widely adopted technique for searching the global optimal data association, it becomes less restrictive as more features are well matched. The early well-matched features contribute little to total matching cost while the gating threshold increases in the chi-square test, which allows the acceptance of bad feature pairings in the last step. In this paper, we propose the Conditional Compatibility (CC) test, which is not only more restrictive than JC test, but also probabilistically sound. The proposed test of a new feature pairing is based on the conditional probability distribution of feature locations given the early pairings. CC test can be added into any JC test based search algorithm, such as Joint Compatibility Branch and Bound (JCBB), Incremental Posterior Joint Compatibility (IPJC) and FastJCBB, without increasing much computational complexity. The more restrictive criterion of accepting a feature pairing, not only helps to reject bad associations, but also bounds the search space, which substantially improves the search efficiency. The real matching experiments justify that our algorithm produces better feature cloud matching results in a more efficient manner.
更多查看译文
关键词
Feature Cloud Matching, Scan Matching, Data Association, Conditional Compatibility Test
AI 理解论文
溯源树
样例
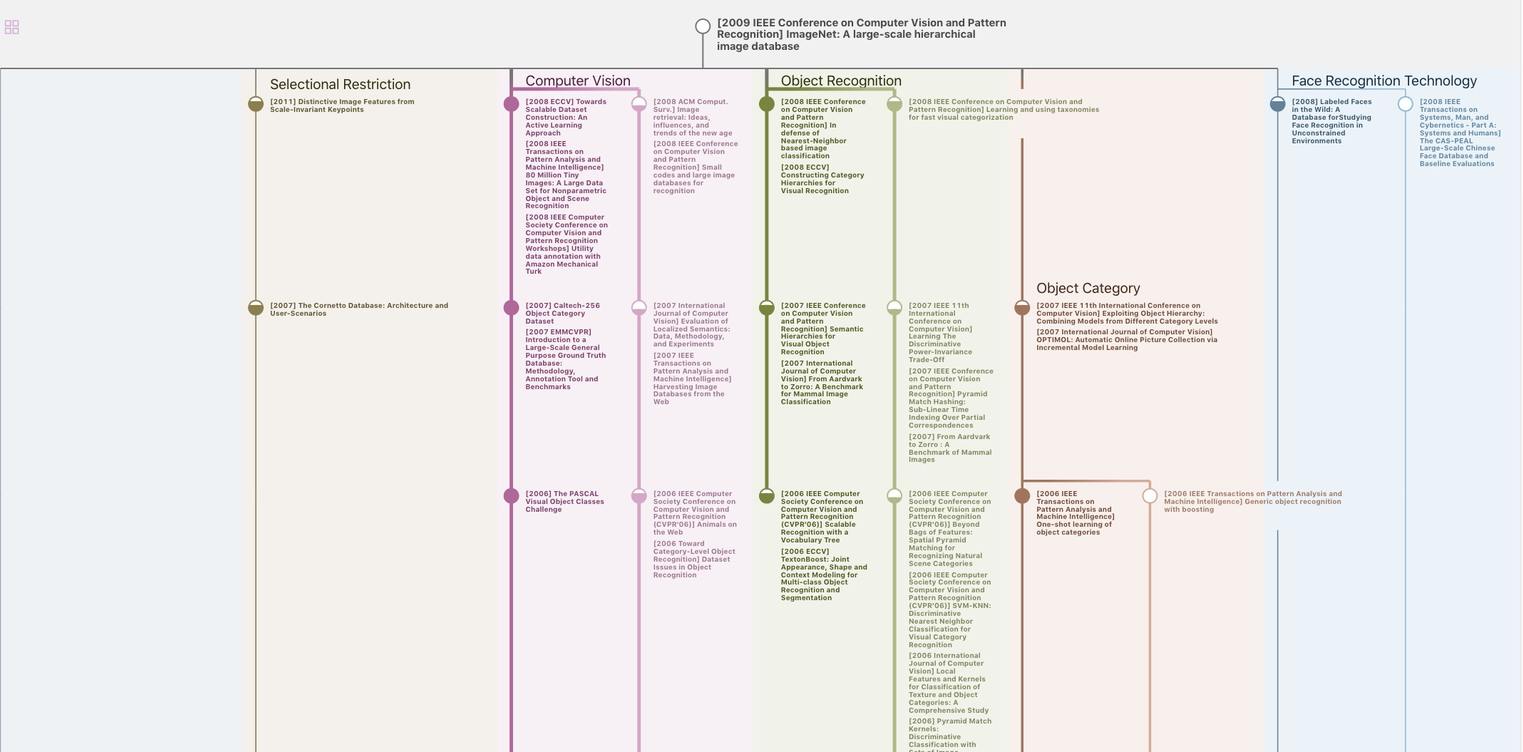
生成溯源树,研究论文发展脉络
Chat Paper
正在生成论文摘要