Machine Learning For Placement-Insensitive Inertial Motion Capture
2018 IEEE INTERNATIONAL CONFERENCE ON ROBOTICS AND AUTOMATION (ICRA)(2018)
摘要
Although existing inertial motion-capture systems work reasonably well (<= 10 degrees error in Euler angles), their accuracy suffers when sensor positions change relative to the associated body segments (+/- 60 degrees mean error and 120 degrees standard deviation). We attribute this performance degradation to undermined calibration values, sensor movement latency and displacement offsets. The latter specifically leads to incongruent rotation matrices in kinematic algorithms that rely on rotational transformations. To overcome these limitations, we propose to employ machine-learning techniques. In particular, we use multi-layer perceptrons to learn sensor-displacement patterns based on 3 hours of motion data collected from 12 test subjects in the lab over 215 trials. Furthermore, to compensate for calibration and latency errors, we directly process sensor data with deep neural networks and estimate the joint angles. Based on these approaches, we demonstrate up to 69% reduction in tracking errors.
更多查看译文
关键词
sensor positions,body segments,standard deviation,calibration values,rotation matrices,inertial motion-capture systems,latency errors,motion data,sensor-displacement patterns,multilayer perceptrons,rotational transformations,kinematic algorithms,sensor movement,performance degradation,Euler angles,placement-insensitive inertial motion capture,machine learning,joint angles,sensor data,time 3.0 hour
AI 理解论文
溯源树
样例
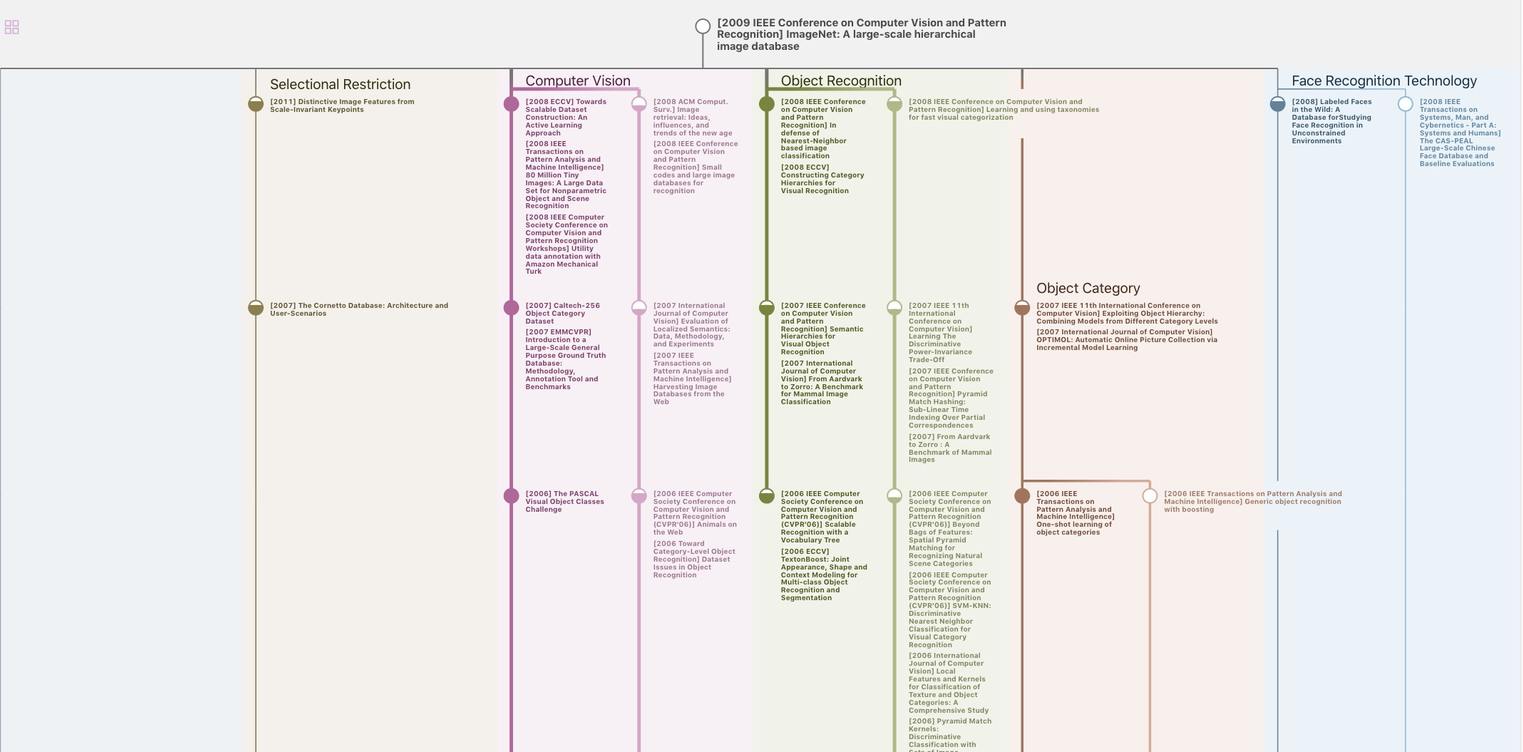
生成溯源树,研究论文发展脉络
Chat Paper
正在生成论文摘要