Learning The Parameters Of Spatially-Referring Natural Language Likelihoods In Binary Models
2018 21ST INTERNATIONAL CONFERENCE ON INFORMATION FUSION (FUSION)(2018)
摘要
Despite imprecision and possible ambiguity expressed by spatially referring natural language statements, they are potentially useful "measurements" (also known as soft data) for target localisation and tracking. The likelihood functions of such measurements typically include the parameters that model the inherent uncertainty in soft data. Adopting a binary model for spatially referring statements involving the word "near", the paper derives the theoretical posterior Cramer-Rao bound for the estimation (learning) of the parameter which features in the likelihood function. A numerical analysis of the bound is presented with an example demonstrating estimation/learning in practice.
更多查看译文
关键词
spatially-referring natural language likelihoods,binary model,natural language statements,soft data,target localisation,likelihood function,target tracking,parameter learning,theoretical posterior Cramér-Rao bound,numerical analysis
AI 理解论文
溯源树
样例
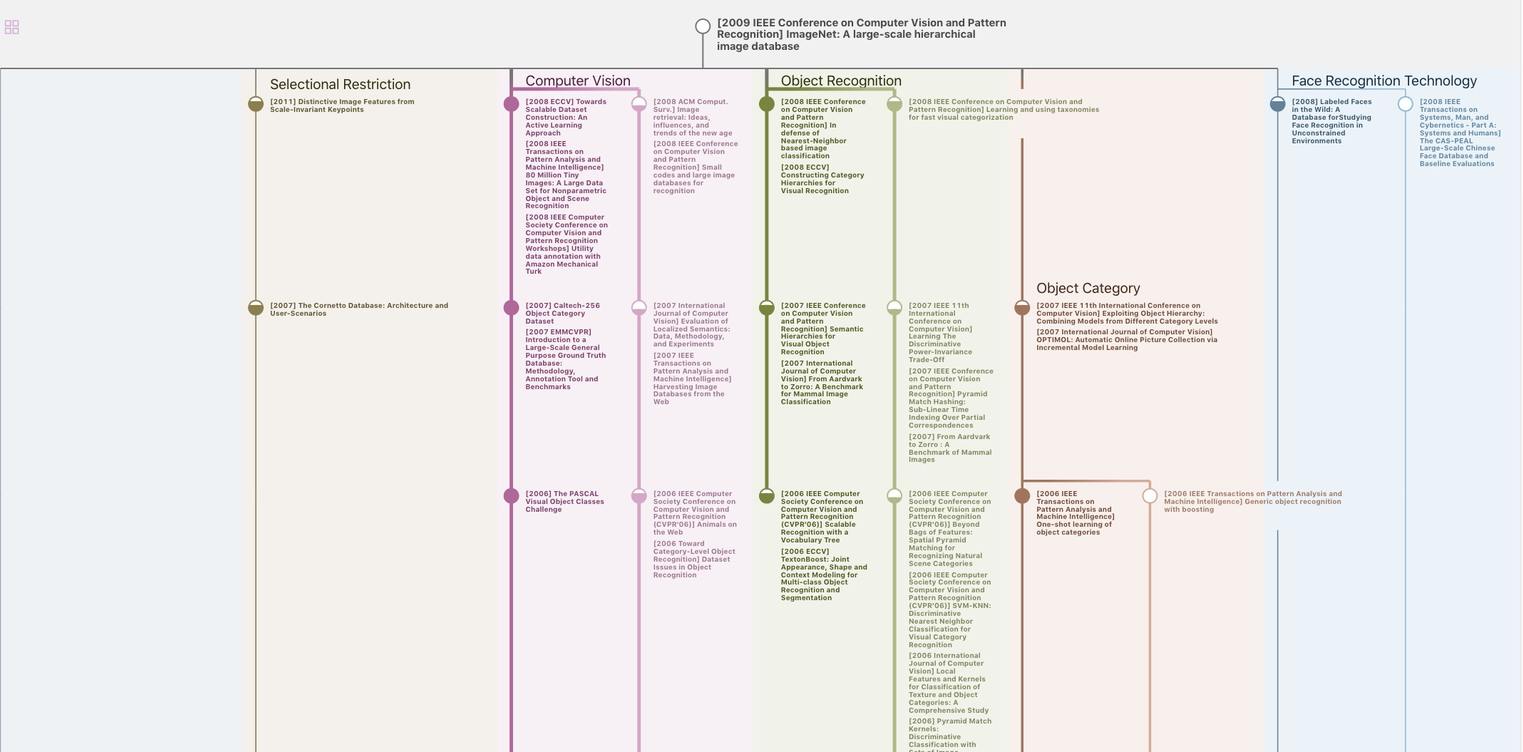
生成溯源树,研究论文发展脉络
Chat Paper
正在生成论文摘要