Reconstruction Of Cross-Correlations With Constant Number Of Deterministic Samples
2018 21ST INTERNATIONAL CONFERENCE ON INFORMATION FUSION (FUSION)(2018)
摘要
Optimal fusion of estimates that are computed in a distributed fashion is a challenging task. In general, the sensor nodes cannot keep track of the cross-correlations required to fuse estimates optimally. In this paper, a novel technique is presented that provides the means to reconstruct the required correlation structure. For this purpose, each node computes a set of deterministic samples that provides all the information required to reassemble the cross-covariance matrix for each pair of estimates. As the number of samples is increasing over time, a method to reduce the size of the sample set is presented and studied. In doing so, communication expenses can be reduced significantly, but approximation errors are possibly introduced by neglecting past correlation terms. In order to keep approximation errors at a minimum, an appropriate set size can be determined and a trade-off between communication expenses and estimation quality can be found.
更多查看译文
关键词
deterministic samples,cross-correlations
AI 理解论文
溯源树
样例
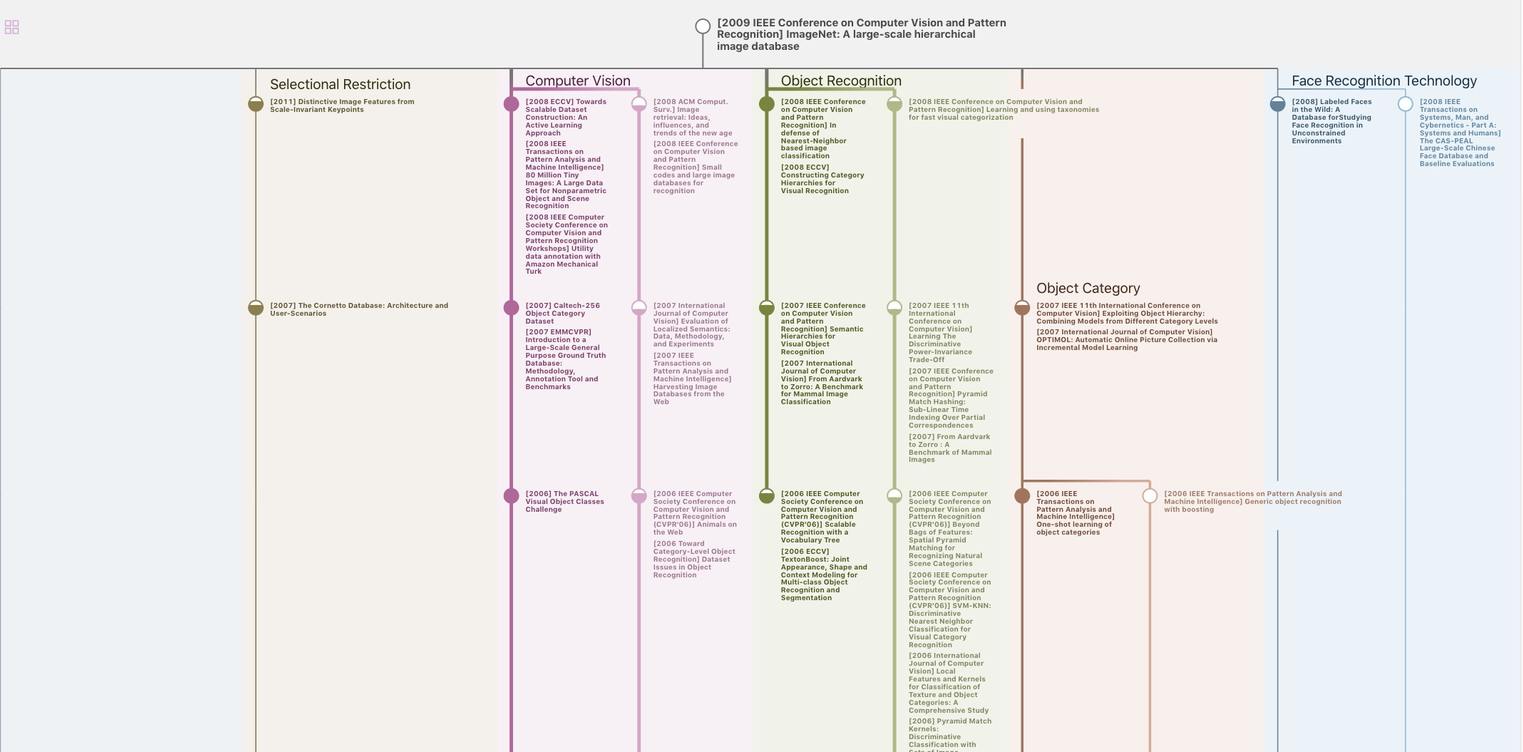
生成溯源树,研究论文发展脉络
Chat Paper
正在生成论文摘要