Retinal Blood Vessel Segmentation Using a Fully Convolutional Network – Transfer Learning from Patch- to Image-Level
MACHINE LEARNING IN MEDICAL IMAGING: 9TH INTERNATIONAL WORKSHOP, MLMI 2018(2018)
Abstract
Fully convolutional networks (FCNs) are well known to provide state-of-the-art results in various medical image segmentation tasks. However, these models usually need a tremendous number of training samples to achieve good performances. Unfortunately, this requirement is often difficult to satisfy in the medical imaging field, due to the scarcity of labeled images. As a consequence, the common tricks for FCNs’ training go from data augmentation and transfer learning to patch-based segmentation. In the latter, the segmentation of an image involves patch extraction, patch segmentation, then patch aggregation. This paper presents a framework that takes advantage of all these tricks by starting with a patch-level segmentation which is then extended to the image level by transfer learning. The proposed framework follows two main steps. Given a image database \(\mathcal {D}\), a first network \(\mathcal {N}_P\) is designed and trained using patches extracted from \(\mathcal {D}\). Then, \(\mathcal {N}_P\) is used to pre-train a FCN \(\mathcal {N}_I\) to be trained on the full sized images of \(\mathcal {D}\). Experimental results are presented on the task of retinal blood vessel segmentation using the well known publicly available DRIVE database.
MoreTranslated text
Key words
Retinal blood vessel segmentation,Fully convolutional neural networks,Transfer learning
AI Read Science
Must-Reading Tree
Example
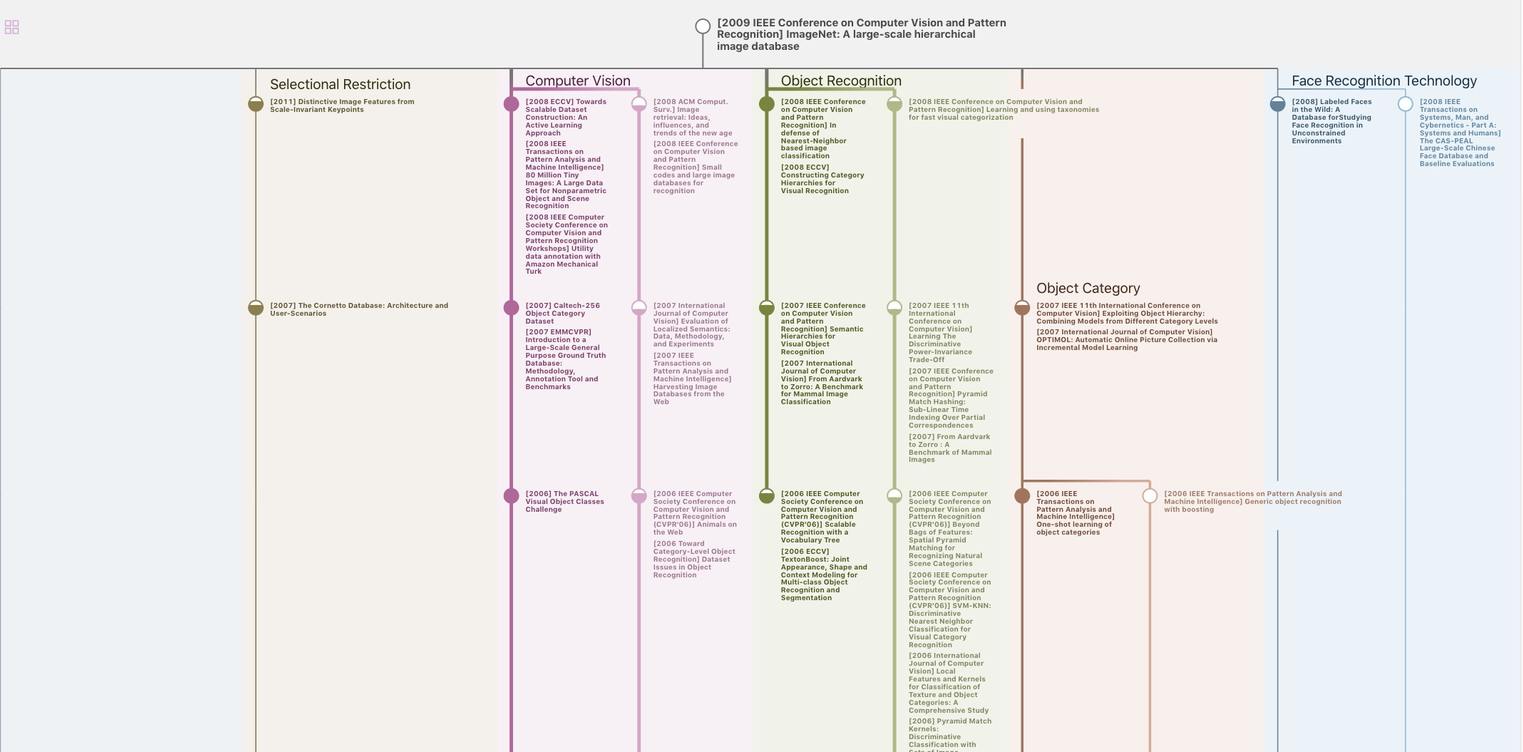
Generate MRT to find the research sequence of this paper
Chat Paper
Summary is being generated by the instructions you defined