Multi-Scale Residual Network With Two Channels Of Raw Ct Image And Its Differential Excitation Component For Emphysema Classification
DEEP LEARNING IN MEDICAL IMAGE ANALYSIS AND MULTIMODAL LEARNING FOR CLINICAL DECISION SUPPORT, DLMIA 2018(2018)
摘要
Automated tissue classification is an essential step for quantitative analysis and treatment of emphysema. Although many studies have been conducted in this area, there still remain two major challenges. First, different emphysematous tissue appears in different scales, which we call "inter-class variations". Second, the intensities of CT images acquired from different patients, scanners or scanning protocols may vary, which we call "intra-class variations". In this paper, we present a novel multi-scale residual network with two channels of raw CT image and its differential excitation component. We incorporate multi-scale information into our networks to address the challenge of inter-class variations. In addition to the conventional raw CT image, we use its differential excitation component as a pair of inputs to handle intra-class variations. Experimental results show that our approach has superior performance over the state-of-the-art methods, achieving a classification accuracy of 93.74% on our original emphysema database.
更多查看译文
关键词
Emphysema classification, Multi-scale, Differential excitation component
AI 理解论文
溯源树
样例
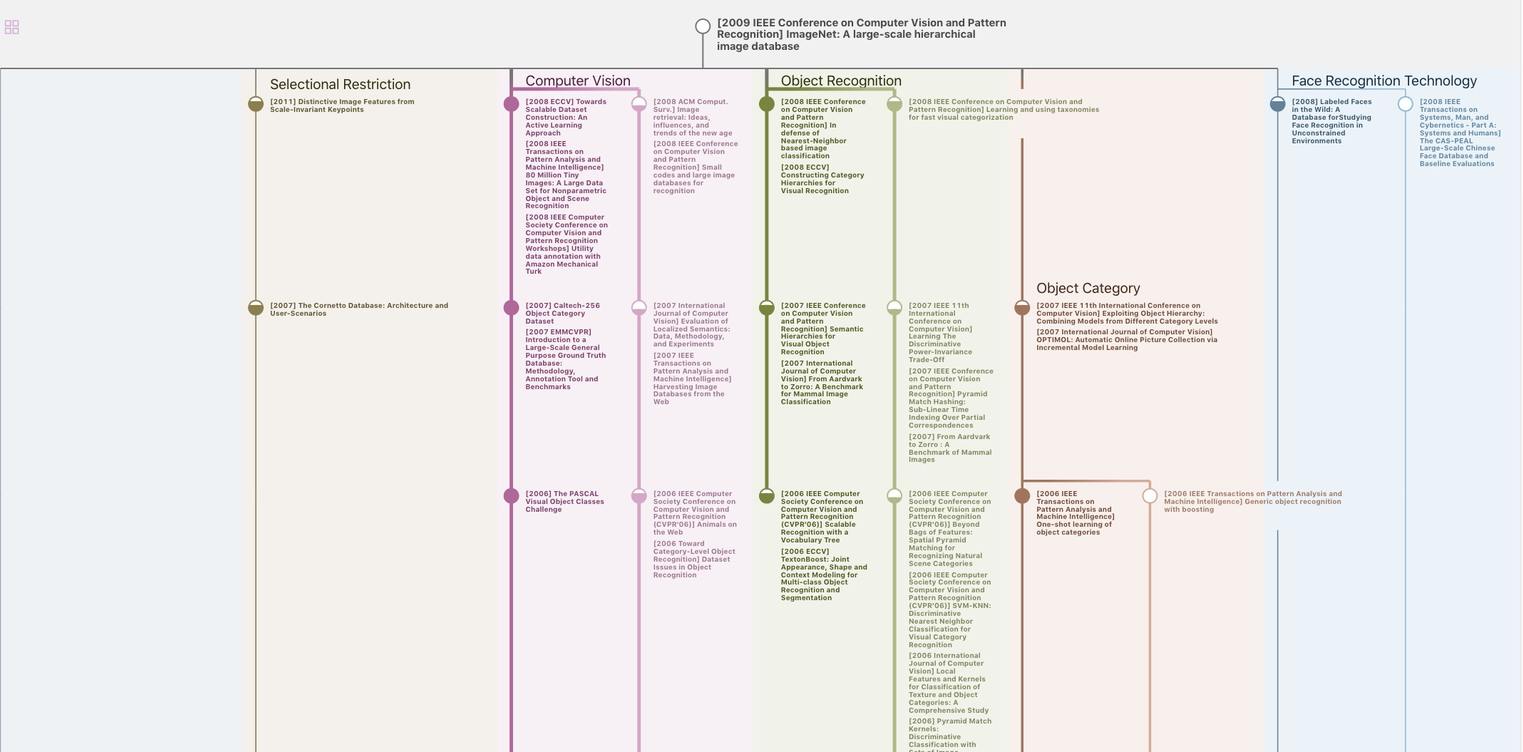
生成溯源树,研究论文发展脉络
Chat Paper
正在生成论文摘要