L1 penalized Cox regression to characterize cardiovascular events in hypertensive patients
2017 COMPUTING IN CARDIOLOGY (CINC)(2017)
摘要
Reliable cardiovascular risk stratification of hypertensive patients is essential to provide appropriate clinical management. However, traditional statistical approaches may ignore important information from datasets and overlook possible interactions among covariates when dealing with high-dimensional data. Regularization techniques, such as least absolute shrinkage and selection operator (LASSO), may improve the prediction accuracy and inter-pretability of regression models. Our aim is to identify the most relevant features to predict cardiovascular (CV) events in hypertensive patients by using the L
1
-penalized Cox regression. We use clinical records of 1664 patients of the Mostoles University Hospital whose CV status was determined by clinical variables and biomarkers including body index mass, blood pressure, cholesterol, albumin/creatinine ratio, and kidney function. By monotoni-cally tuning its regularization parameter, the LASSO approach put forth is able to identify the most predictive features, with "cystanin C-based glomerular filtration" being the single most relevant predictor for CV events.
更多查看译文
关键词
biomarkers,least absolute shrinkage and selection operator,LASSO,regression model interpretability,Mostoles University Hospital,body index mass,blood pressure,cholesterol,albumin-creatinine ratio,kidney function,monotonic regularization parameter tuning,cystanin C-based glomerular filtration,predictive features,LASSO approach,clinical variables,clinical records,prediction accuracy,regularization techniques,high-dimensional data,appropriate clinical management,reliable cardiovascular risk stratification,hypertensive patients,cardiovascular events,L1 penalized Cox regression
AI 理解论文
溯源树
样例
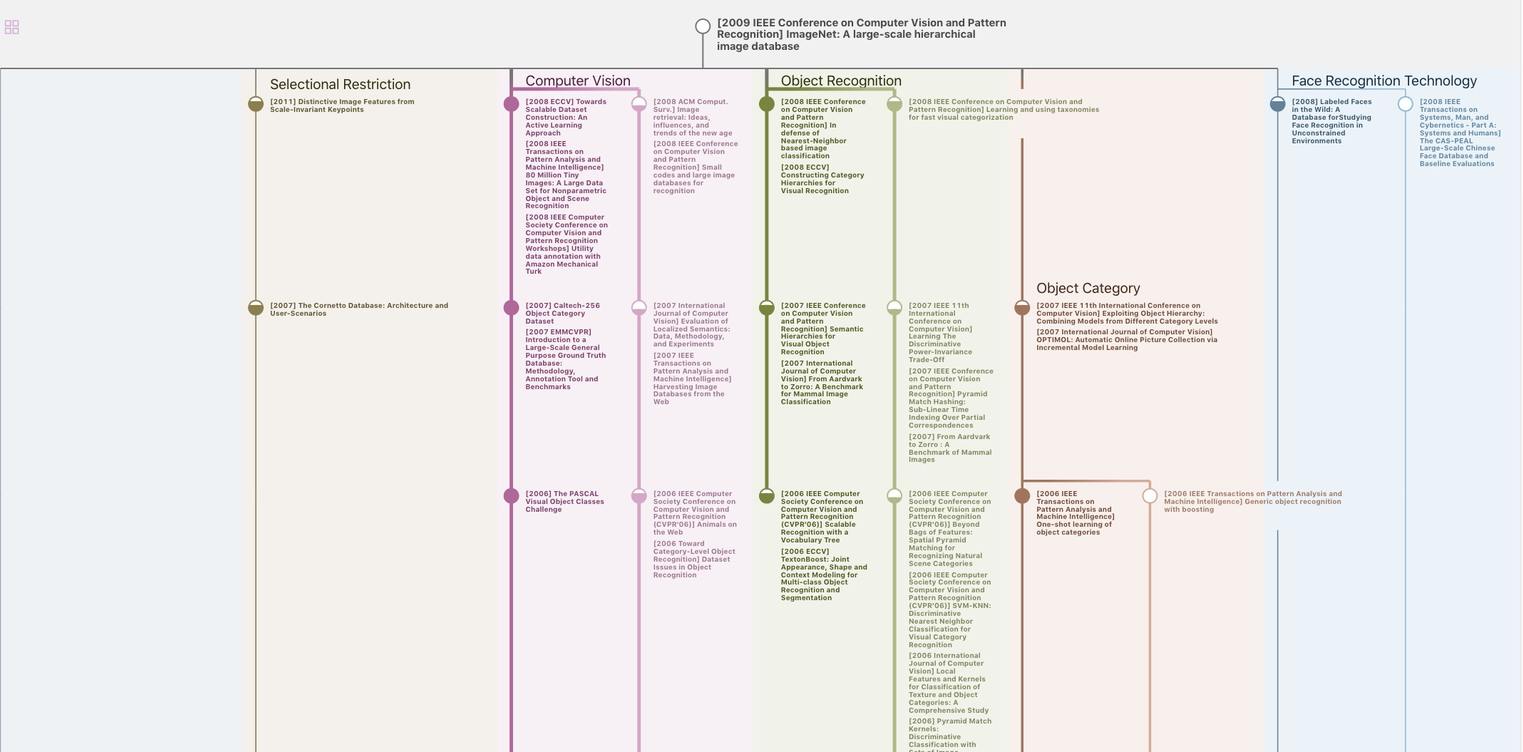
生成溯源树,研究论文发展脉络
Chat Paper
正在生成论文摘要