A Comparative Study of Classifying Legal Documents with Neural Networks.
Federated Conference on Computer Science and Information Systems(2018)
摘要
In recent years, deep learning has shown promising results when used in the field of natural language processing (NLP). Neural networks (NNs) such as convolutional neural networks (CNNs) and recurrent neural networks (RNNs) have been used for various NLP tasks including sentiment analysis, information retrieval, and document classification. In this paper, we the present the Supreme Court Classifier (SCC), a system that applies these methods to the problem of document classification of legal court opinions. We compare methods using traditional machine learning with recent NN-based methods. We also present a CNN used with pre-trained word vectors which shows improvements over the state-of-the-art applied to our dataset. We train and evaluate our system using the Washington University School of Law Supreme Court Database (SCDB). Our best system (word2vec + CNN) achieves 72.4% accuracy when classifying the court decisions into 15 broad SCDB categories and 31.9% accuracy when classifying among 279 liner-grained SCDB categories.
更多查看译文
关键词
pre-trained word vectors,court decisions,deep learning,natural language processing,convolutional neural networks,recurrent neural networks,information retrieval,document classification,legal court opinions,traditional machine learning,recent NN-based methods,NLP tasks,legal documents classification,CNN,word2vec,Supreme Court classifier,Law Supreme Court database,sentiment analysis
AI 理解论文
溯源树
样例
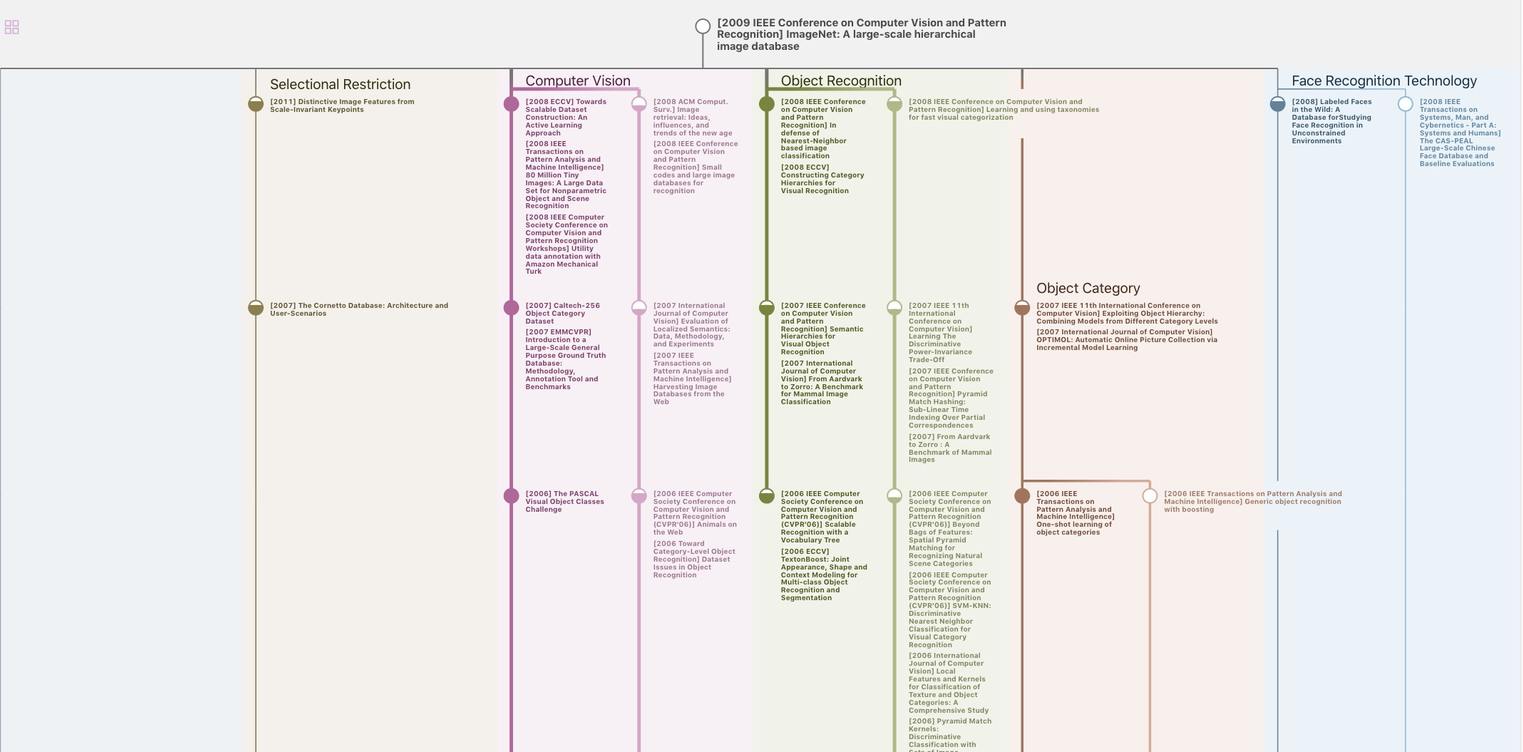
生成溯源树,研究论文发展脉络
Chat Paper
正在生成论文摘要