Parallax: Automatic Data-Parallel Training of Deep Neural Networks.
arXiv: Distributed, Parallel, and Cluster Computing(2018)
Abstract
The employment of high-performance servers and GPU accelerators for training deep neural network models have greatly accelerated recent advances in machine learning (ML). ML frameworks, such as TensorFlow, MXNet, and Caffe2, have emerged to assist ML researchers to train their models in a distributed fashion. However, correctly and efficiently utilizing multiple machines and GPUs is still not a straightforward task for framework users due to the non-trivial correctness and performance challenges that arise in the distribution process. This paper introduces Parallax, a tool for automatic parallelization of deep learning training in distributed environments. Parallax not only handles the subtle correctness issues, but also leverages various optimizations to minimize the communication overhead caused by scaling out. Experiments show that Parallax built atop TensorFlow achieves scalable training throughput on multiple CNN and RNN models, while requiring little effort from its users.
MoreTranslated text
AI Read Science
Must-Reading Tree
Example
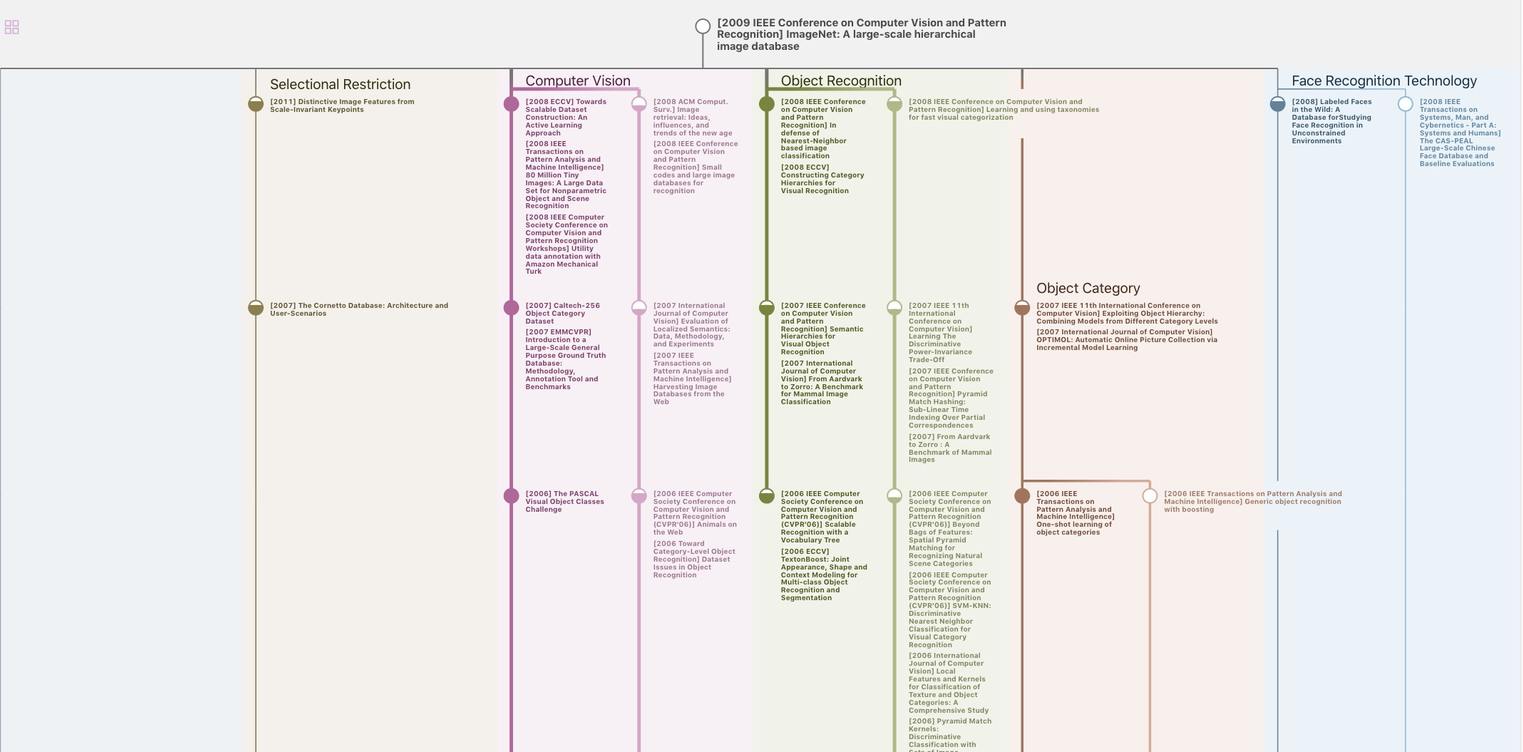
Generate MRT to find the research sequence of this paper
Chat Paper
Summary is being generated by the instructions you defined