A Comparative Assessment Of Economic Model Predictive Control Strategies For Fuel Economy Optimization Of Heavy-Duty Trucks
2018 ANNUAL AMERICAN CONTROL CONFERENCE (ACC)(2018)
摘要
This paper provides a comparative assessment of three control strategies that fuse a global, offline dynamic programming (DP) optimization with online model predictive control (MPC) in an effort to minimize fuel consumption for a heavy-duty truck. The online MPC optimization, which is local in nature, makes refinements to a coarsely (but globally, subject to grid resolution) optimized target velocity profile from the DP optimization. Three candidate economic MPC formulations are evaluated: a time-based formulation that directly penalizes predicted fuel consumption, a simplified time-based formulation that penalizes braking effort in place of fuel consumption, and a distance-based convex formulation that maintains a tradeoff between energy expenditure and tracking of the coarsely optimized velocity based on DP. The performance of each approach is presented for three representative route profiles, using a medium-fidelity proprietary Volvo model of the heavy-duty truck's longitudinal dynamics. Results demonstrate 47% fuel economy improvement across all three formulations, when compared to a baseline strategy. Furthermore, we present a detailed analysis of energy usage by "type" (aerodynamic losses, braking losses, and comparison of brake-specific fuel consumption), under each candidate control approach.
更多查看译文
关键词
brake-specific fuel consumption,economic model predictive control strategies,online model predictive control,online MPC optimization,velocity profile,DP optimization,candidate economic MPC formulations,simplified time-based formulation,distance-based convex formulation,coarsely optimized velocity,representative route profiles,medium-fidelity proprietary Volvo model,fuel economy improvement,global-offline dynamic programming optimization,heavy-duty truck fuel economy optimization
AI 理解论文
溯源树
样例
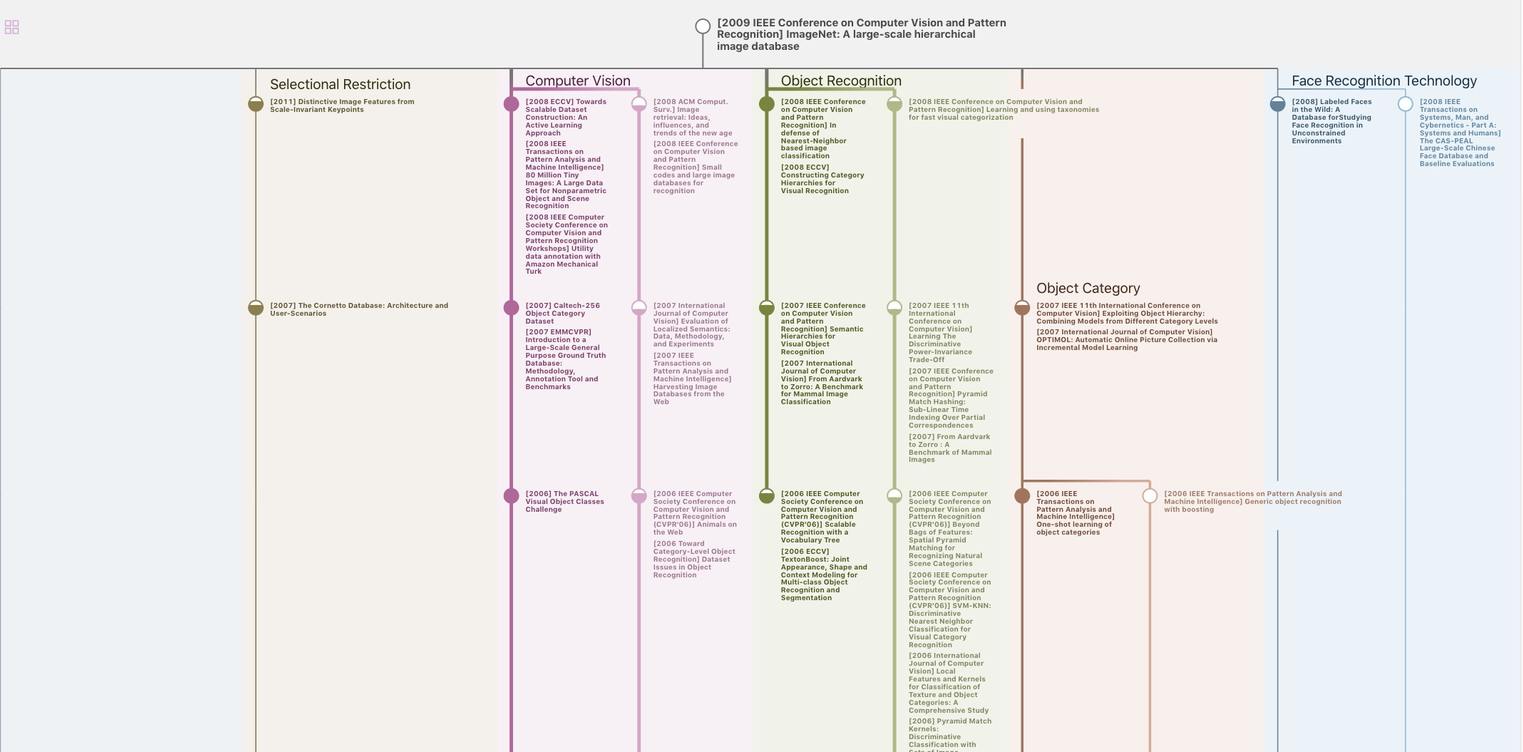
生成溯源树,研究论文发展脉络
Chat Paper
正在生成论文摘要