A Dynamic Programming-Based Real-Time Predictive Optimal Gear Shift Strategy For Conventional Heavy-Duty Vehicles
2018 ANNUAL AMERICAN CONTROL CONFERENCE (ACC)(2018)
摘要
This paper examines the problem of utilizing upcoming terrain and vehicle speed predictions for gear shift trajectory optimization in conventional heavy-duty vehicles. The paper is motivated by the fuel savings potential of such optimization, especially in connected and automated heavy-duty trucks. A key goal of this work is to develop a computationally tractable online shifting algorithm with a fuel saving potential approaching that of existing offline global optimization methods from the literature. We consider two optimization objectives, namely, fuel consumption and gear shift frequency. We use dynamic programming to navigate the Pareto tradeoff between these objectives offline, for known vehicle duty cycles. The resulting gear shift trajectories collapse to an instantaneous shift map in the Pareto limit where fuel consumption minimization is the sole objective. We construct a neural network that anticipates the upcoming Pareto-optimal gear shift decision, given a sequence of gear shifts deemed ideal by the simple, instantaneous Pareto-limit shift map. We train this neural network using mix of urban, suburban, and highway drive cycles. The neural network reduces fuel consumption by 0.43%-4.16% in simulation, compared to a benchmark rule-based gear shift strategy.
更多查看译文
关键词
vehicle speed predictions,neural network,dynamic programming,heavy-duty vehicles,fuel savings,global optimization methods,optimal gear shift strategy,vehicle duty cycles,Pareto-optimal gear shift decision
AI 理解论文
溯源树
样例
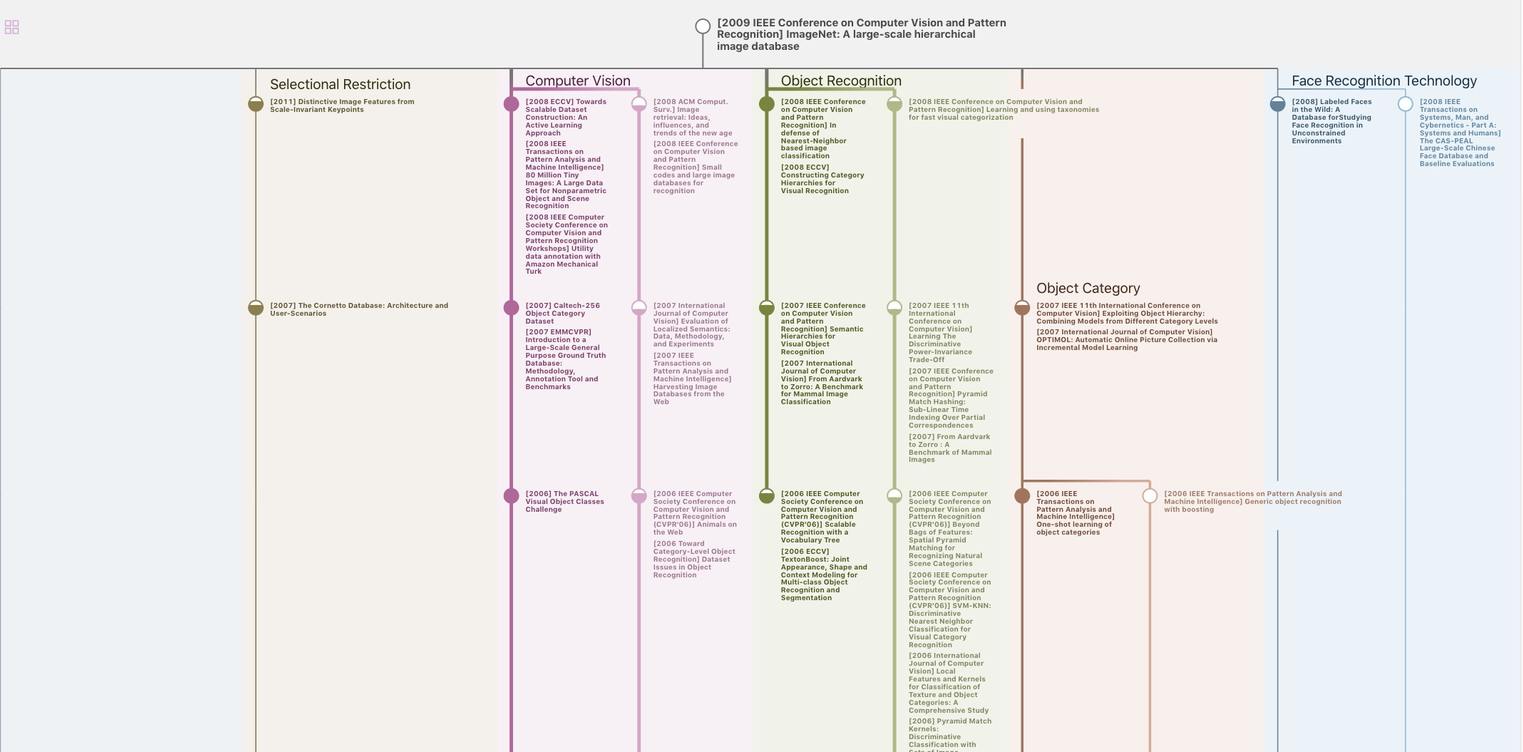
生成溯源树,研究论文发展脉络
Chat Paper
正在生成论文摘要