Unsupervised Morphology Learning with Statistical Paradigms.
COLING(2018)
摘要
This paper describes an unsupervised model for morphological segmentation that exploits the notion of paradigms, which are sets of morphological categories (e.g., suffixes) that can be applied to a homogeneous set of words (e.g., nouns or verbs). Our algorithm identifies statistically reliable paradigms from the morphological segmentation result of a probabilistic model, and chooses reliable suffixes from them. The new suffixes can be fed back iteratively to improve the accuracy of the probabilistic model. Finally, the unreliable paradigms are subjected to pruning to eliminate unreliable morphological relations between words. The paradigm-based algorithm significantly improves segmentation accuracy. Our method achieves start-of-the-art results on experiments using the Morpho-Challenge data, including English, Turkish, and Finnish.
更多查看译文
关键词
morphology,statistical paradigms,learning
AI 理解论文
溯源树
样例
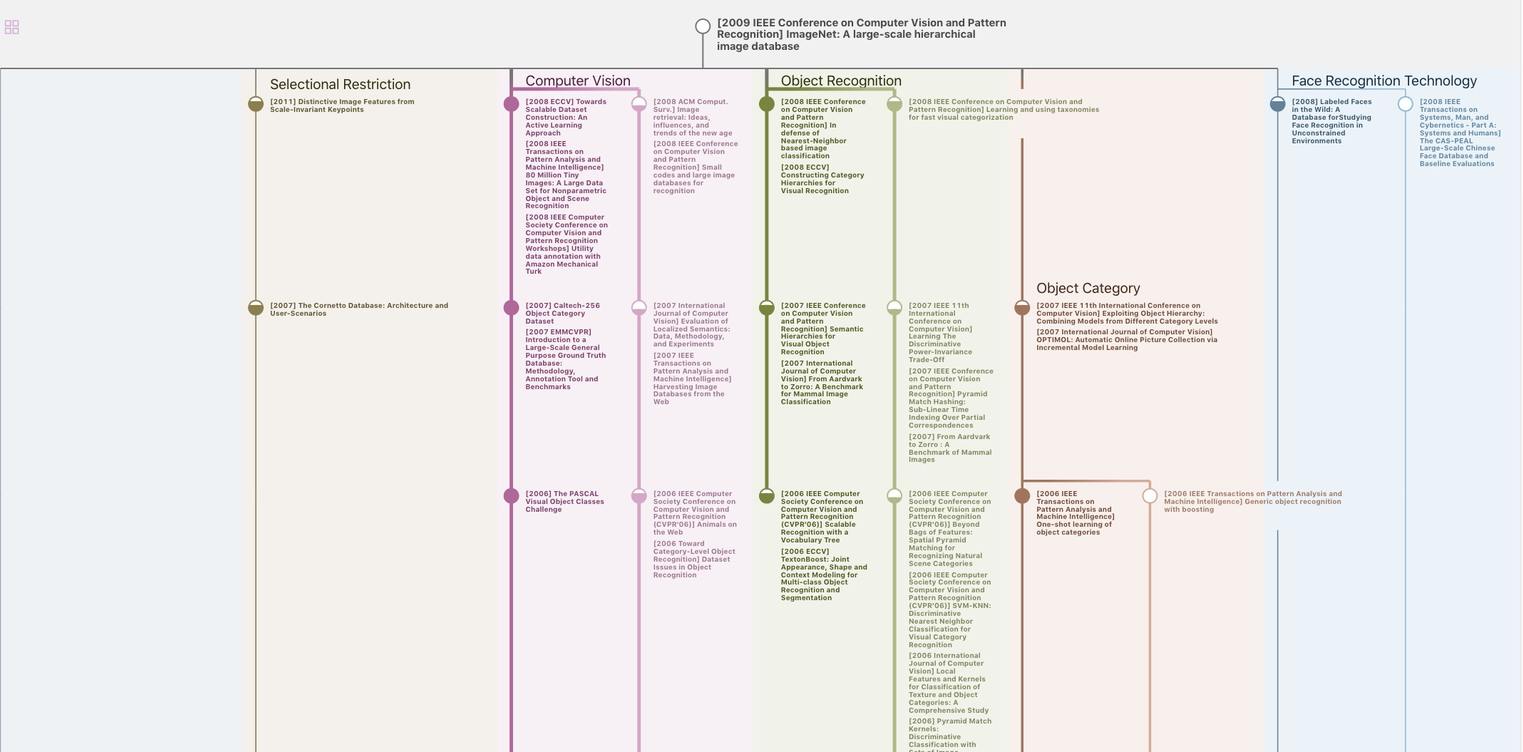
生成溯源树,研究论文发展脉络
Chat Paper
正在生成论文摘要