Early Termination of Failed HPC Jobs Through Machine and Deep Learning.
Euro-Par(2018)
摘要
Failed jobs in a supercomputer cause not only waste in CPU time or energy consumption but also decrease work efficiency of users. Mining data collected during the operation of data centers helps to find patterns explaining failures and can be used to predict them. Automating system reactions, e.g., early termination of jobs, when software failures are predicted does not only increase availability and reduce operating cost, but it also frees administrators’ and users’ time. In this paper, we explore a unique dataset containing the topology, operation metrics, and job scheduler history from the petascale Mistral supercomputer. We extract the most relevant system features deciding on the final state of a job through decision trees. Then, we successfully train a neural net to predict job evolution based on power time series of nodes. Finally, we evaluate the effect on CPU time saving for static and dynamic job termination policies.
更多查看译文
关键词
HPC, Slurm, Failure prediction, Failure prevention, Deep learning, Data center
AI 理解论文
溯源树
样例
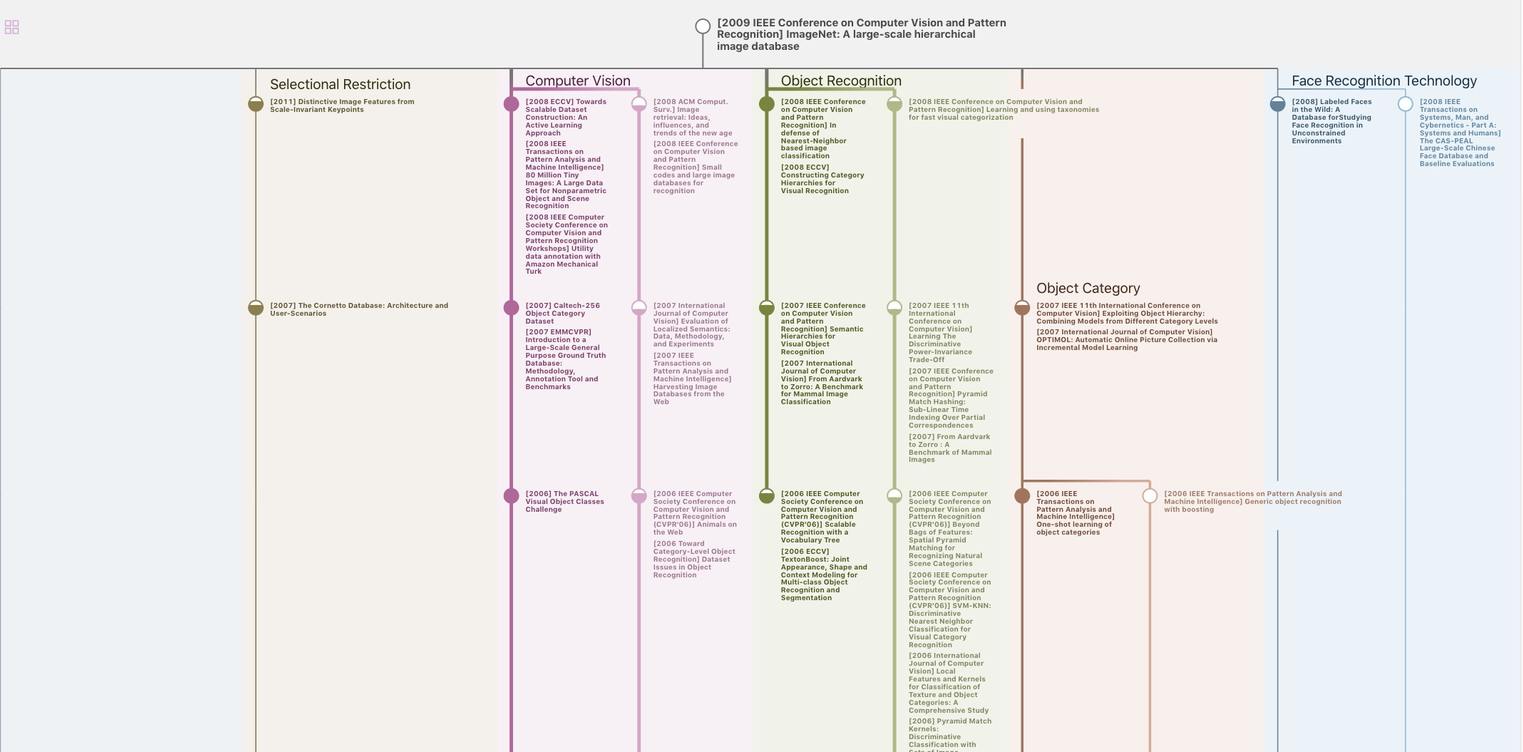
生成溯源树,研究论文发展脉络
Chat Paper
正在生成论文摘要