Nobody Cares if You Liked Star Wars: KNN Graph Construction on the Cheap.
Euro-Par(2018)
摘要
K-Nearest-Neighbors (KNN) graphs play a key role in a large range of applications. A KNN graph typically connects entities characterized by a set of features so that each entity becomes linked to its k most similar counterparts according to some similarity function. As datasets grow, KNN graphs are unfortunately becoming increasingly costly to construct, and the general approach, which consists in reducing the number of comparisons between entities, seems to have reached its full potential. In this paper we propose to overcome this limit with a simple yet powerful strategy that samples the set of features of each entity and only keeps the least popular features. We show that this strategy outperforms other more straightforward policies on a range of four representative datasets: for instance, keeping the 25 least popular items reduces computational time by up to 63%, while producing a KNN graph close to the ideal one.
更多查看译文
关键词
Popular Items, Sampling Policy, MovieLens 1M, Complementary Cumulative Distribution Functions (CCDF), Jaccard Similarity
AI 理解论文
溯源树
样例
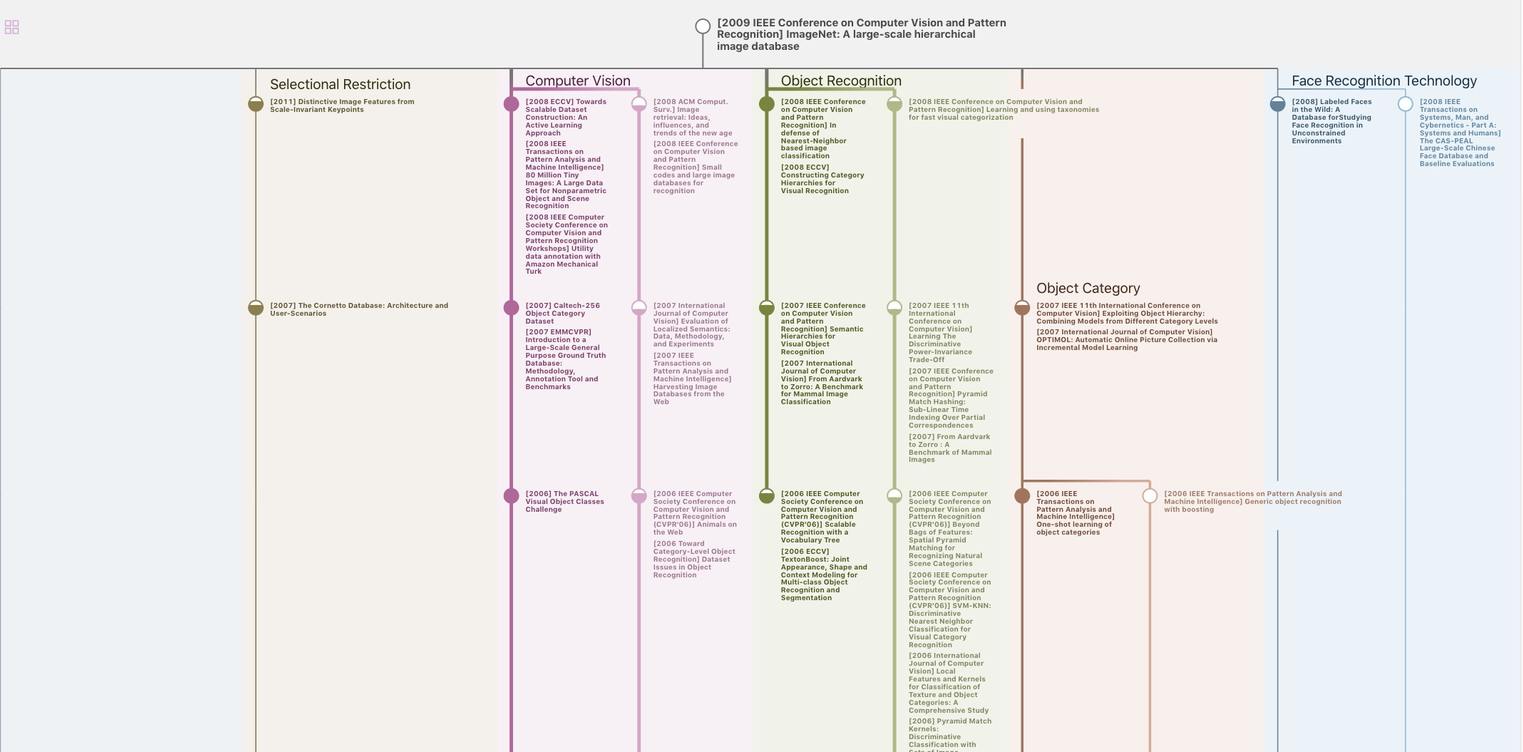
生成溯源树,研究论文发展脉络
Chat Paper
正在生成论文摘要