Workload-aware Parameter Selection and Performance Prediction for In-memory Databases.
SBBD(2018)
摘要
In-memory databases, just as hard drive ones, may offer hundreds of customizable settings, making the task of system tuning overwhelming for a database administrator. Even worse, the number of parameters continues to grow over the years and they can affect performance in a not intuitive manner. Models that capture their behavior can assist automatic tuning mechanisms to obtain optimal performance. In this work, we propose a learning-based approach to select the most meaningful parameters and generate a performance model based on both the workload and the database configurations. Experimental results confirm that our approach can create accurate performance models using only a reduced set of selected parameters.
更多查看译文
AI 理解论文
溯源树
样例
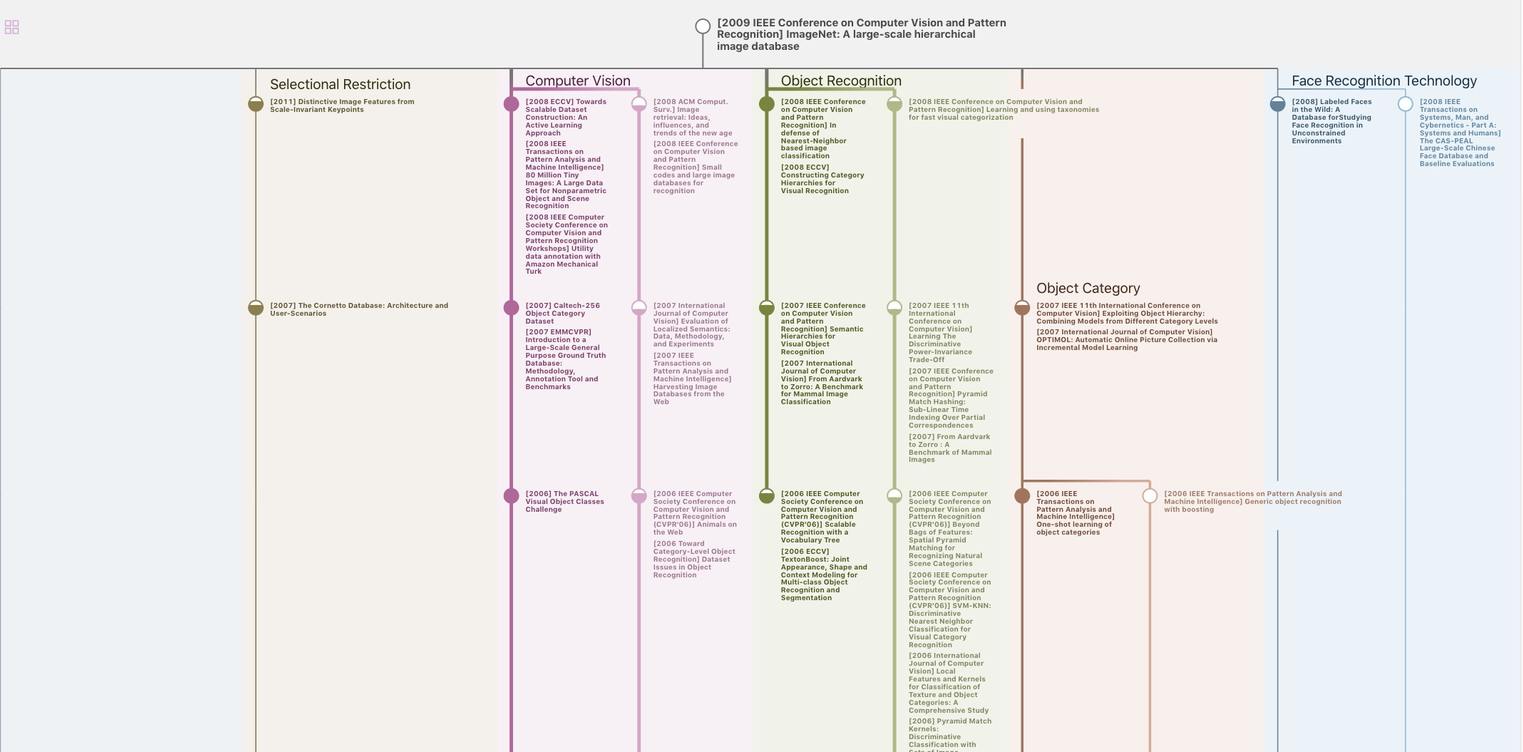
生成溯源树,研究论文发展脉络
Chat Paper
正在生成论文摘要