Oboe: auto-tuning video ABR algorithms to network conditions.
SIGCOMM(2018)
摘要
Most content providers are interested in providing good video delivery QoE for all users, not just on average. State-of-the-art ABR algorithms like BOLA and MPC rely on parameters that are sensitive to network conditions, so may perform poorly for some users and/or videos. In this paper, we propose a technique called Oboe to auto-tune these parameters to different network conditions. Oboe pre-computes, for a given ABR algorithm, the best possible parameters for different network conditions, then dynamically adapts the parameters at run-time for the current network conditions. Using testbed experiments, we show that Oboe significantly improves BOLA, MPC, and a commercially deployed ABR. Oboe also betters a recently proposed reinforcement learning based ABR, Pensieve, by 24% on average on a composite QoE metric, in part because it is able to better specialize ABR behavior across different network states.
更多查看译文
关键词
Video delivery, Adaptive bitrate algorithms
AI 理解论文
溯源树
样例
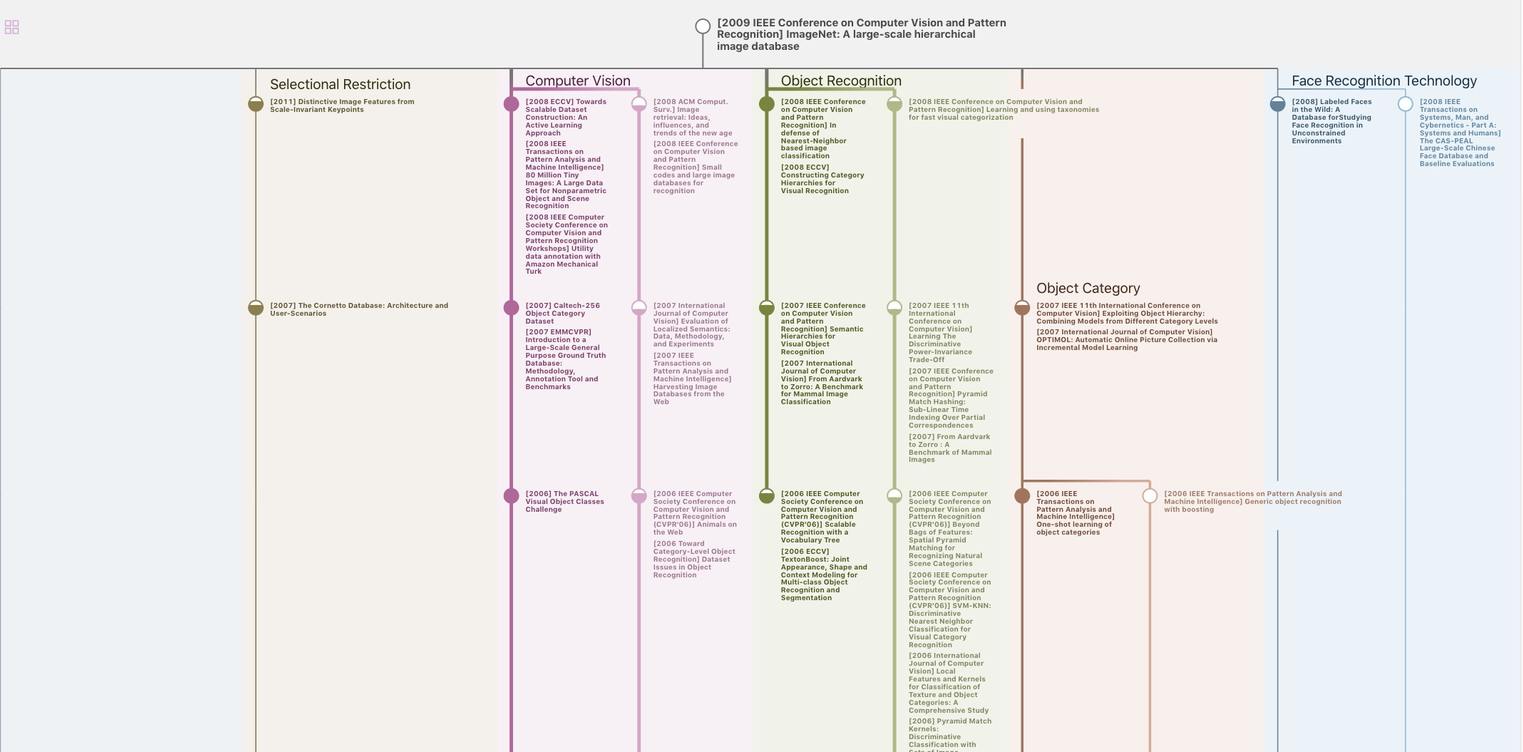
生成溯源树,研究论文发展脉络
Chat Paper
正在生成论文摘要