Analyzing Genomic Data Using Tensor-based Orthogonal Polynomials
BCB(2018)
摘要
Rapid increases in the availability of genomic data for diverse organisms has spurred the search for better mathematical and computational methods to investigate the underlying patterns that connect genotypic and phenotypic data. Large genomic datasets make it possible to search for higher order epistatic interactions, but also highlight the need for new mathematical tools that can simultaneously represent sequences and phenotypes. We propose a multivariate tensor-based orthogonal polynomial approach to characterize nucleotides or amino acids in a DNA/RNA or protein sequence. Given phenotype data and corresponding sequences, we can construct orthogonal polynomials using sequence information and subsequently map phenotypes on to the space of the polynomials. This approach provides information about higher order associations between different parts of a sequence, and allows us to identify both linear and nonlinear relationships between phenotype and genomic or proteomic sequence data. We use this method to assess the relationship between sequences and transcription activity levels in a large raw mammalian enhancer dataset downloaded from NCBI. We provide insights into the bioinformatics and computational pipeline necessary to curate and translate large-scale genomic data to extract and quantify complex genome-phenotype interactions.
更多查看译文
关键词
Epistasis, Tensor-based Multivariate Orthogonal Polynomials, Bioinformatics Pipeline, Mammalian Enhancers, Transcription
AI 理解论文
溯源树
样例
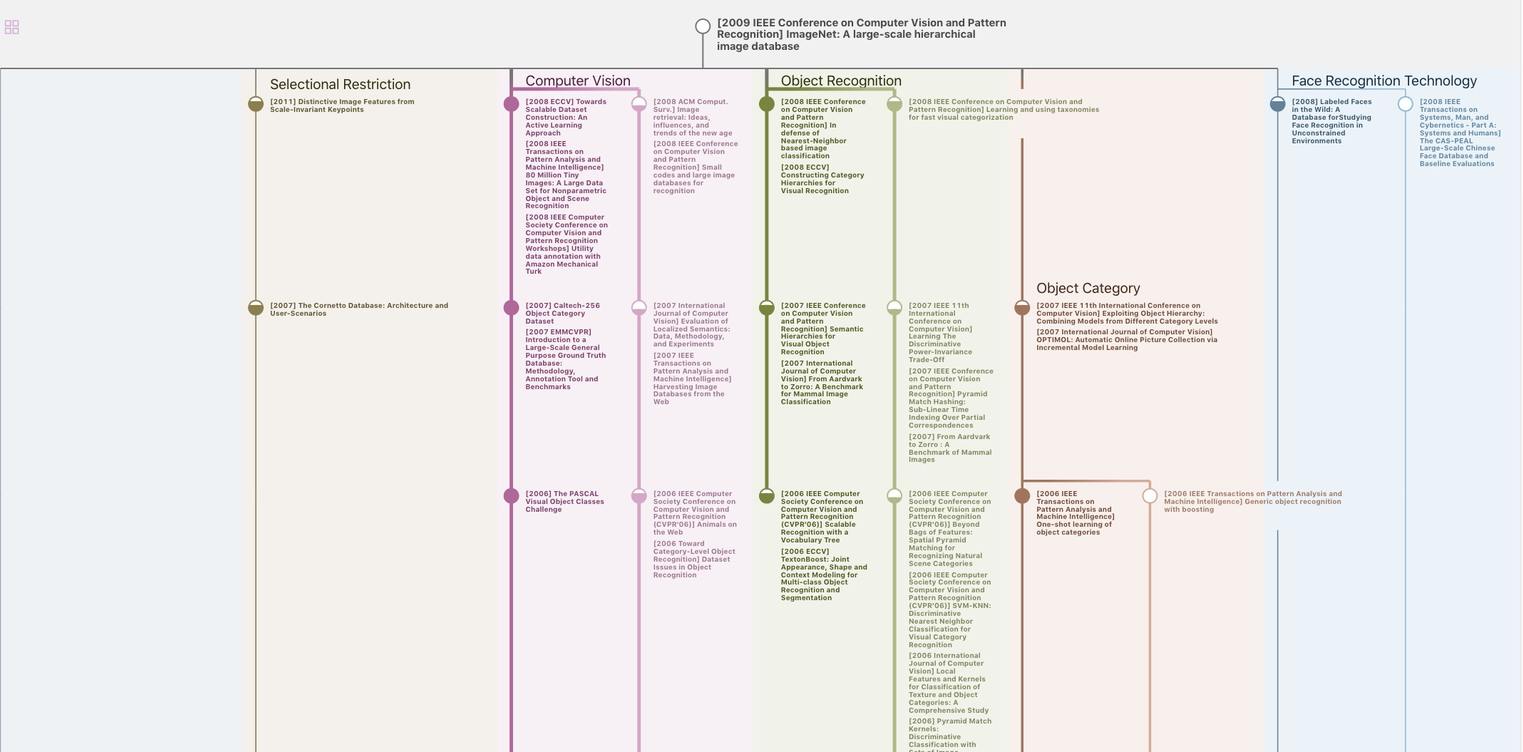
生成溯源树,研究论文发展脉络
Chat Paper
正在生成论文摘要