The National Sleep Research Resource: Towards a Sleep Data Commons.
BCB(2018)
摘要
Objective: The gold standard for diagnosing sleep disorders is polysomnography, which generates extensive data about biophysical changes occurring during sleep. We developed the National Sleep Research Resource (NSRR), a comprehensive system for sharing sleep data. The NSRR embodies elements of a data commons aimed at accelerating research to address critical questions about the impact of sleep disorders on important health outcomes. Approach: We used a metadata-guided approach, with a set of common sleep-specific terms enforcing uniform semantic interpretation of data elements across three main components: (1) annotated datasets; (2) user interfaces for accessing data; and (3) computational tools for the analysis of polysomnography recordings. We incorporated the process for managing dataset-specific data use agreements, evidence of Institutional Review Board review, and the corresponding access control in the NSRR web portal. The metadata-guided approach facilitates structural and semantic interoperability, ultimately leading to enhanced data reusability and scientific rigor. Results: The authors curated and deposited retrospective data from 10 large, NIH-funded sleep cohort studies, including several from the Trans-Omics for Precision Medicine (TOPMed) program, into the NSRR. The NSRR currently contains data on 26,808 subjects and 31,166 signal files in European Data Format. Launched in April 2014, over 3000 registered users have downloaded over 130 terabytes of data. Conclusions: The NSRR offers a use case and an example for creating a full-fledged data commons. It provides a single point of access to analysis-ready physiological signals from polysomnography obtained from multiple sources, and a wide variety of clinical data to facilitate sleep research. The NIH Data Commons (or Commons) is an ambitious vision for a shared virtual space to allow digital objects to be stored and computed upon by the scientific community. The Commons would allow investigators to find, manage, share, use and reuse data, software, metadata and workflows. It imagines an ecosystem that makes digital objects Findable, Accessible, Interoperable and Reusable (FAIR). Four components are considered integral parts of the Commons: a computing resource for accessing and processing of digital objects; a "digital object compliance model" that describes the properties of digital objects that enable them to be FAIR; datasets that adhere to the digital object compliance model; and software and services to facilitate access to and use of data. This paper describes the contributions of NSRR along several aspects of the Commons vision: metadata for sleep research digital objects; a collection of annotated sleep data sets; and interfaces and tools for accessing and analyzing such data. More importantly, the NSRR provides the design of a functional architecture for implementing a Sleep Data Commons. The NSRR also reveals complexities and challenges involved in making clinical sleep data conform to the FAIR principles. Future directions: Shared resources offered by emerging resources such as cloud instances provide promising platforms for the Data Commons. However, simply expanding storage or adding compute power may not allow us to cope with the rapidly expanding volume and increasing complexity of biomedical data. Concurrent efforts must be spent to address digital object organization challenges. To make our approach future-proof, we need to continue advancing research in data representation and interfaces for human-data interaction. A possible next phase of NSRR is the creation of a universal self-descriptive sequential data format. The idea is to break large, unstructured, sequential data files into minimal, semantically meaningful, fragments. Such fragments can be indexed, assembled, retrieved, rendered, or repackaged on-the-fly, for multitudes of application scenarios. Data points in such a fragment will be locally embedded with relevant metadata labels, governed by terminology and ontology. Potential benefits of such an approach may include precise levels of data access, increased analysis readiness with on-the-fly data conversion, multi-level data discovery and support for effective web-based visualization of contents in large sequential files.
更多查看译文
关键词
data commons,data integration,data sharing,common data element,data provenance,sleep research,polysomnography,data quality,European Data Format
AI 理解论文
溯源树
样例
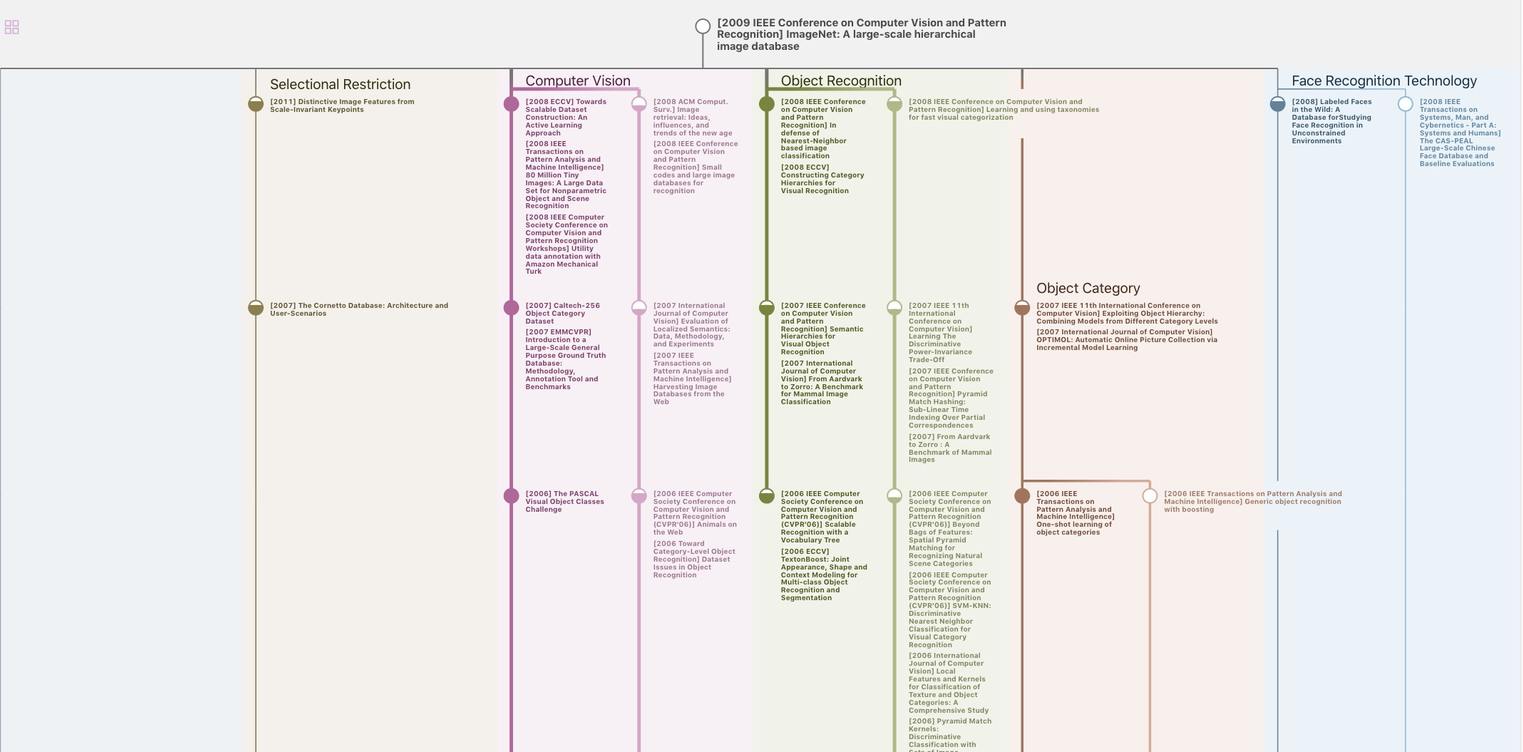
生成溯源树,研究论文发展脉络
Chat Paper
正在生成论文摘要