Modeling Alzheimer’s disease progression using deep recurrent neural networks
2018 International Workshop on Pattern Recognition in Neuroimaging (PRNI)(2018)
摘要
Multi-step prediction, predicting values multiple steps ahead, is a difficult problem because of the accumulation of error after successive predictions. Recurrent neural networks (RNN) have been applied successfully to multi-step prediction problems such as language modeling where training data are abundant and regular. However, applying RNN to problems in healthcare is still challenging as RNN requires large, feature complete training data in which intervals between time points are fixed which are not reflective of healthcare data. To overcome these problems, we propose strategies to train RNN using missing data which involve using the RNN to fill in the incomplete or missing time points online. The strategies enables the efficient use of training data as well as the adoption of RNN in problems where the variables in a multivariate time series are recorded at irregular intervals or at different frequencies, where there are a lot of missing data. We train RNN using the Alzheimer's Disease Neuroimaging Initiative (ADNI) database to model the monthly progression of Alzheimer's disease for seven years into the future which is a multi-step prediction problem. We show that RNN outperforms baseline models.
更多查看译文
关键词
disease progression modeling,multi-step prediction,recurrent neural networks,missing data imputation
AI 理解论文
溯源树
样例
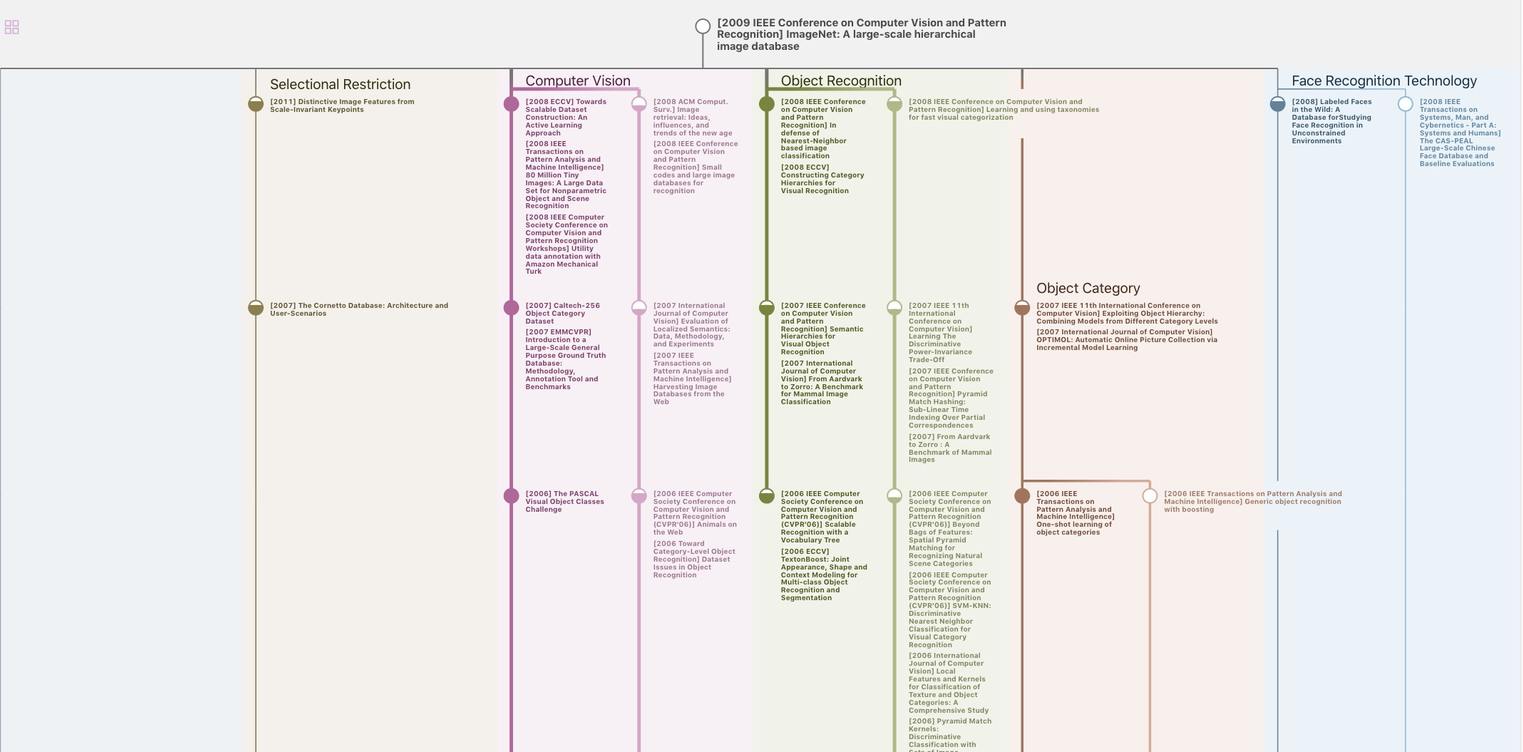
生成溯源树,研究论文发展脉络
Chat Paper
正在生成论文摘要