Ranking-Based Affect Estimation Of Motion Capture Data In The Valence-Arousal Space
PROCEEDINGS OF THE 5TH INTERNATIONAL CONFERENCE ON MOVEMENT AND COMPUTING (MOCO'18)(2018)
摘要
Affect estimation consists of building a predictive model of the perceived affect given stimuli. In this study, we are looking at the perceived affect in full-body motion capture data of various movements. There are two parts to this study. In the first part, we conduct groundtruthing on affective labels of motion capture sequences by hosting a survey on a crowdsourcing platform where participants from all over the world ranked the relative valence and arousal of one motion capture sequences to another. In the second part, we present our experiments with training a machine learning model for pairwise ranking of motion capture data using RankNet. Our analysis shows a reasonable strength in the inter-rater agreement between the participants. The evaluation of the RankNet demonstrates that it can learn to rank the motion capture data, with higher confidence in the arousal dimension compared to the valence dimension.
更多查看译文
关键词
Affective computing, motion capture, machine learning
AI 理解论文
溯源树
样例
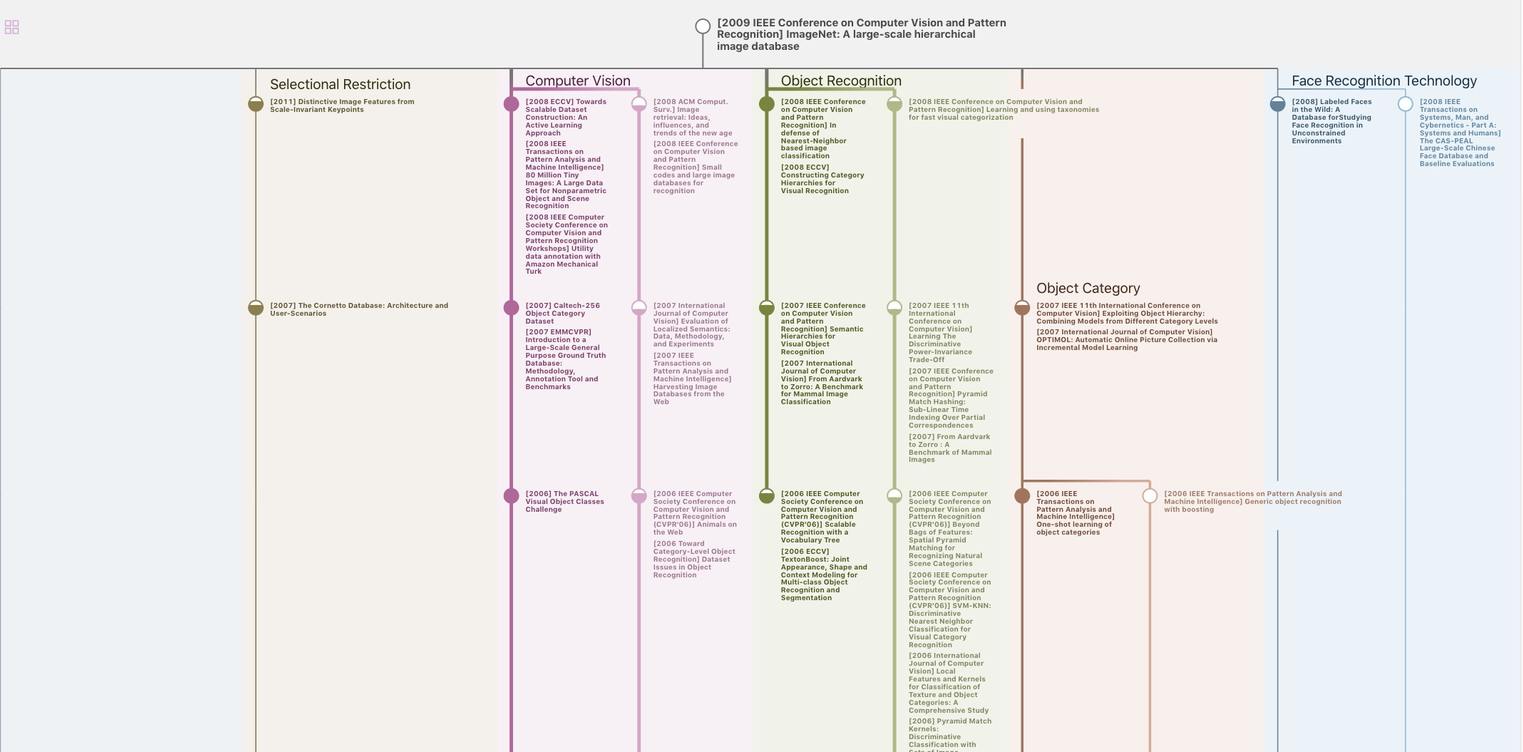
生成溯源树,研究论文发展脉络
Chat Paper
正在生成论文摘要