Differentiable Learning-to-Normalize via Switchable Normalization
international conference on learning representations(2018)
摘要
We address a learning-to-normalize problem by proposing Switchable Normalization (SN), which learns to select different normalizers for different normalization layers of a deep neural network. SN employs three distinct scopes to compute statistics (means and variances) including a channel, a layer, and a minibatch. SN switches between them by learning their importance weights in an end-to-end manner. It has several good properties. First, it adapts to various network architectures and tasks (see Fig.1). Second, it is robust to a wide range of batch sizes, maintaining high performance even when small minibatch is presented (e.g. 2 images/GPU). Third, SN does not have sensitive hyper-parameter, unlike group normalization that searches the number of groups as a hyper-parameter. Without bells and whistles, SN outperforms its counterparts on various challenging benchmarks, such as ImageNet, COCO, CityScapes, ADE20K, and Kinetics. Analyses of SN are also presented. We hope SN will help ease the usage and understand the normalization techniques in deep learning. The code of SN has been made available in https://github.com/switchablenorms/.
更多查看译文
关键词
complex configuration,isolated nucleosomes,current method,novel method,coverage profile,model organism,nucleosome position,nucleosome positioning,occupancy coverage profile,occupancy level
AI 理解论文
溯源树
样例
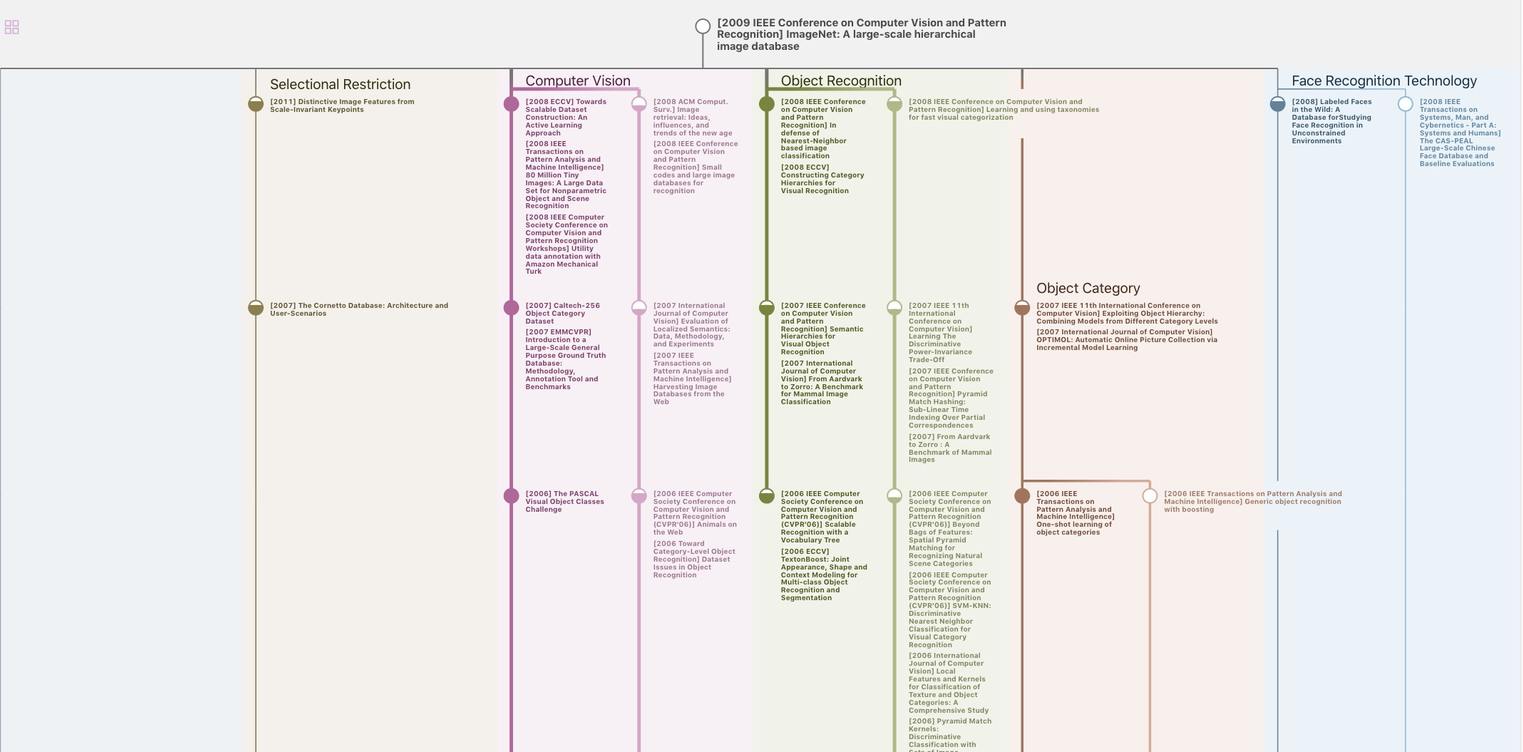
生成溯源树,研究论文发展脉络
Chat Paper
正在生成论文摘要