Diagonal Discriminant Analysis with Feature Selection for High Dimensional Data.
JOURNAL OF COMPUTATIONAL AND GRAPHICAL STATISTICS(2020)
摘要
We introduce a new method of performing high-dimensional discriminant analysis (DA), which we call multiDA. Starting from multiclass diagonal DA classifiers which avoid the problem of high-dimensional covariance estimation we construct a hybrid model that seamlessly integrates feature selection components. Our feature selection component naturally simplifies to weights which are simple functions of likelihood ratio test statistics allowing natural comparisons with traditional hypothesis testing methods. We provide heuristic arguments suggesting desirable asymptotic properties of our algorithm with regard to feature selection. We compare our method with several other approaches, showing marked improvements in regard to prediction accuracy, interpretability of chosen features, and fast run time. We demonstrate such strengths of our model by showing strong classification performance on publicly available high-dimensional datasets, as well as through multiple simulation studies. We make an R package available implementing our approach. for this article are available online.
更多查看译文
关键词
Asymptotic properties of hypothesis tests,Classification,Feature selection,Latent variables,Likelihood ratio tests,Multiple hypothesis testing
AI 理解论文
溯源树
样例
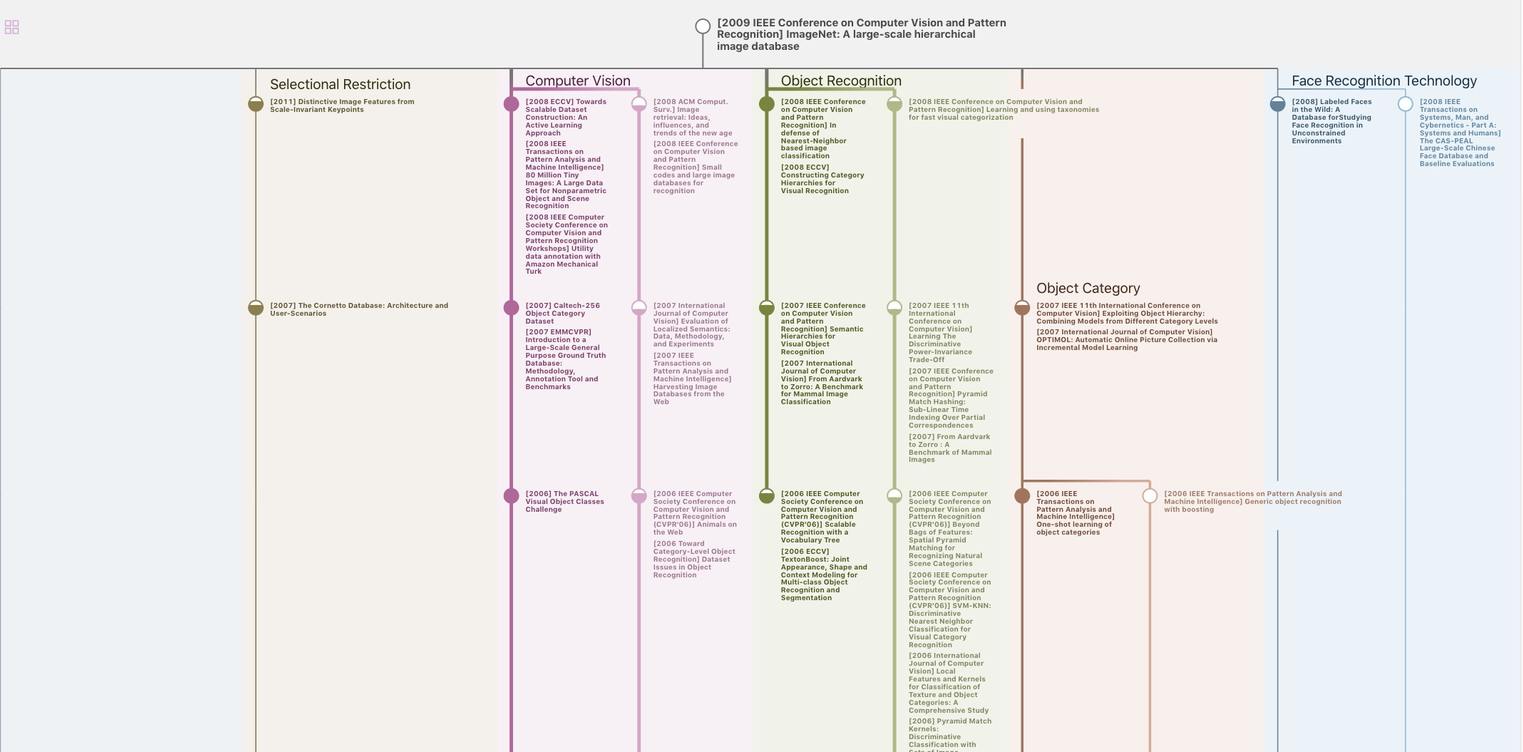
生成溯源树,研究论文发展脉络
Chat Paper
正在生成论文摘要