Forecasting market states.
QUANTITATIVE FINANCE(2019)
摘要
We propose a novel methodology to define, analyze and forecast market states. In our approach, market states are identified by a reference sparse precision matrix and a vector of expectation values. In our procedure, each multivariate observation is associated to a given market state accordingly to a minimization of a penalized Mahalanobis distance. The procedure is made computationally very efficient and can be used with a large number of assets. We demonstrate that this procedure is successful at clustering different states of the markets in an unsupervised manner. In particular, we describe an experiment with one hundred log-returns and two states in which the methodology automatically associates states prevalently to pre- and post-crisis periods with one state gathering periods with average positive returns and the other state periods with average negative returns, therefore discovering spontaneously the common classification of 'bull' and 'bear' markets. In another experiment, with again one hundred log-returns and two states, we demonstrate that this procedure can be efficiently used to forecast off-sample future market states with significant prediction accuracy. This methodology opens the way to a range of applications in risk management and trading strategies in the context where the correlation structure plays a central role.
更多查看译文
关键词
Financial market states,Temporal clustering,Information filtering networks,TMFG,LoGo,Sparse inverse covariance,Correlation structure
AI 理解论文
溯源树
样例
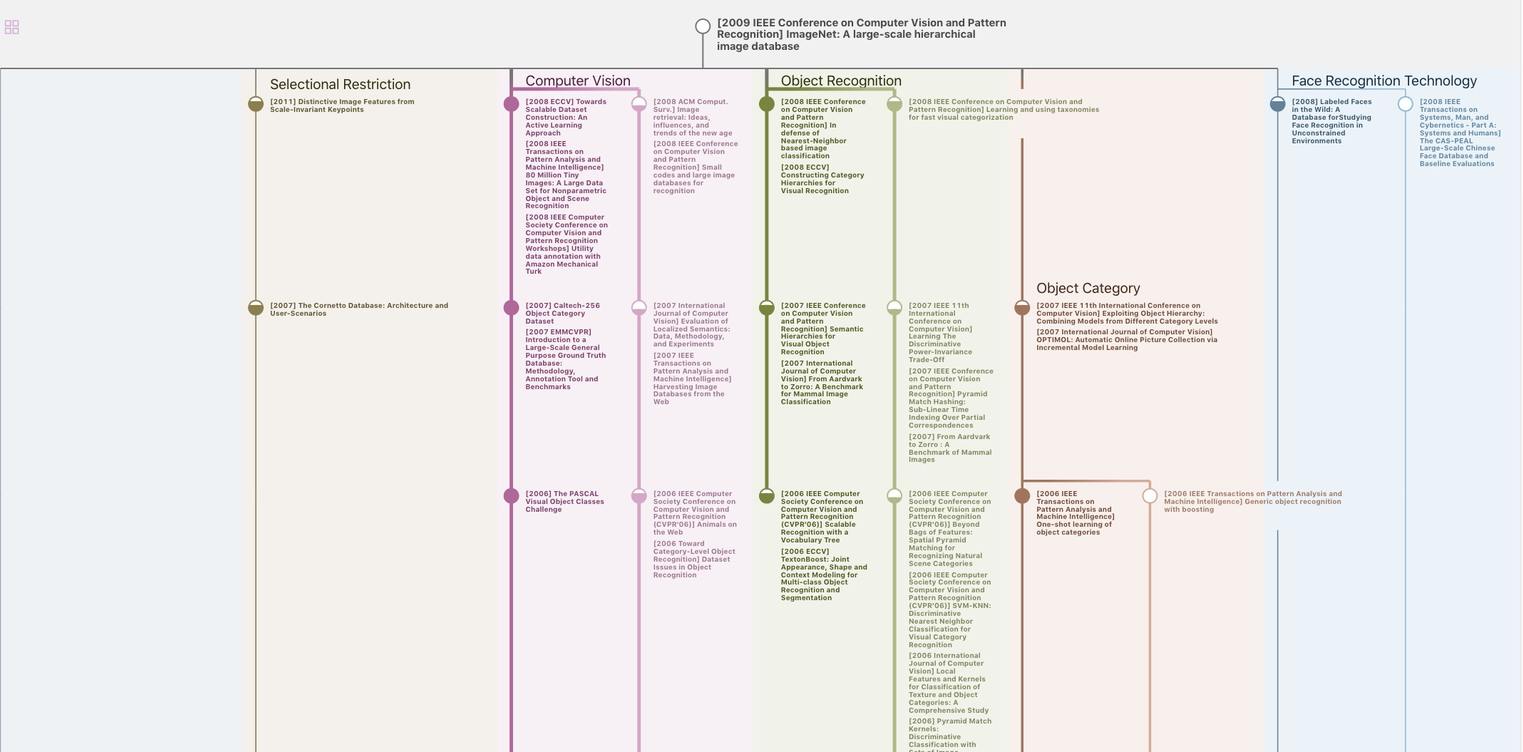
生成溯源树,研究论文发展脉络
Chat Paper
正在生成论文摘要