Structured Bayesian Gaussian process latent variable model
Journal of Computational Physics(2019)
摘要
We introduce a methodology for nonlinear inverse problems using a variational Bayesian approach where the unknown quantity is a spatial field. A structured Bayesian Gaussian process latent variable model is used both to construct a low-dimensional generative model of the sample-based stochastic prior as well as a surrogate for the forward evaluation. Its Bayesian formulation captures epistemic uncertainty introduced by the limited number of input and output examples, automatically selects an appropriate dimensionality for the learned latent representation of the data, and rigorously propagates the uncertainty of the data-driven dimensionality reduction of the stochastic space through the forward model surrogate. The structured Gaussian process model explicitly leverages spatial information for an informative generative prior to improve sample efficiency while achieving computational tractability through Kronecker product decompositions of the relevant kernel matrices. Importantly, the Bayesian inversion is carried out by solving a variational optimization problem, replacing traditional computationally-expensive Monte Carlo sampling. The methodology is demonstrated on an elliptic PDE and is shown to return well-calibrated posteriors and is tractable with latent spaces with over 100 dimensions. • Structured Bayesian GP-LVM introduced for data-driven dimensionality reduction and surrogate modeling of simulation codes, given limited data. • Variational Bayesian treatment of latent variables rigorously propagates uncertainty in the learned dimensionality reduction. • Bayesian inversion is accomplished by solving a variational optimization problem using gradient-based methods.
更多查看译文
关键词
Gaussian processes,Bayesian inference,Variational inference,Uncertainty quantification,Surrogate models,Stochastic partial differential equations
AI 理解论文
溯源树
样例
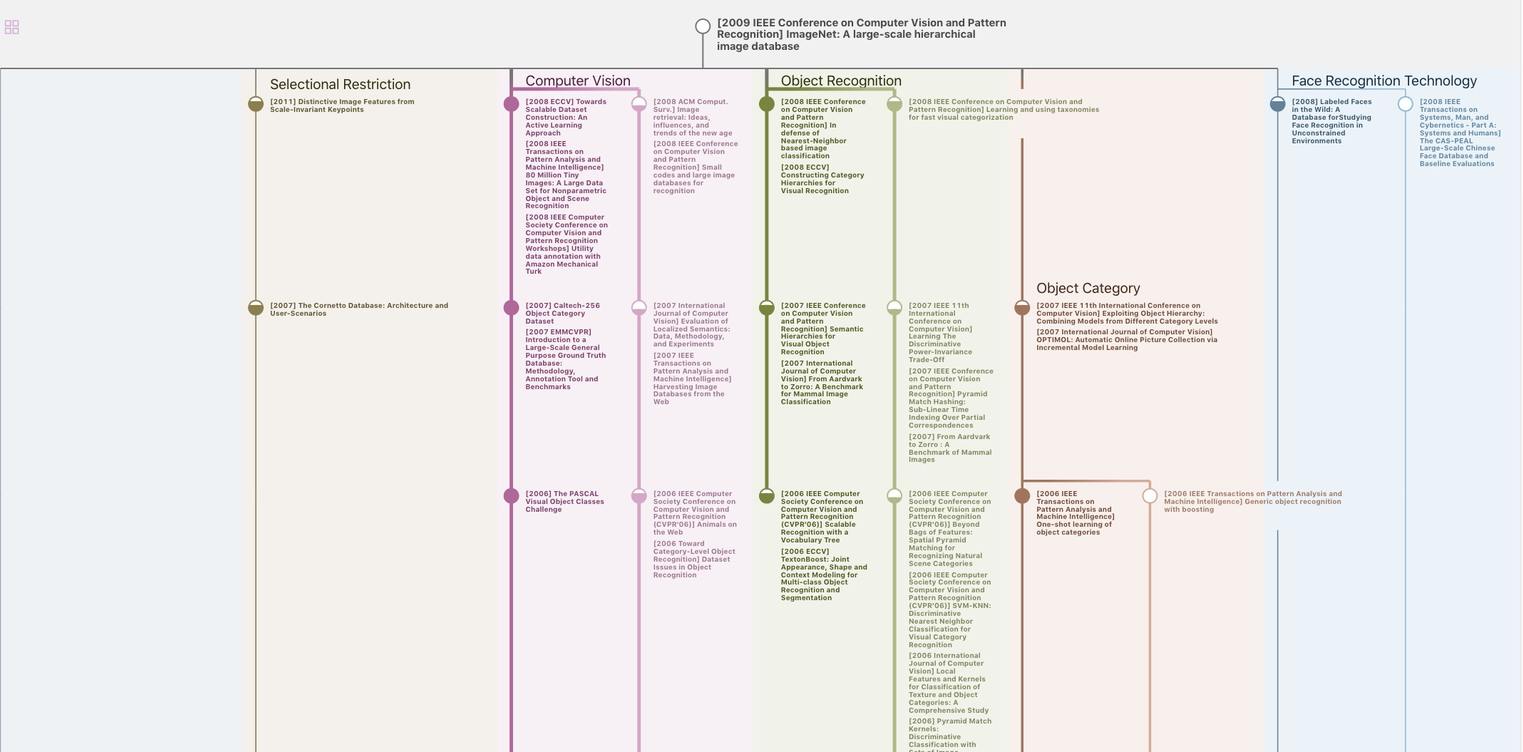
生成溯源树,研究论文发展脉络
Chat Paper
正在生成论文摘要