The Unusual Effectiveness of Averaging in GAN Training.
international conference on learning representations(2019)
摘要
We show empirically that the optimal strategy of parameter averaging in a minmax convex-concave game setting is also strikingly effective in the non convex-concave GAN setting, specifically alleviating the convergence issues associated with cycling behavior observed in GANs. We show that averaging over generator parameters outside of the trainig loop consistently improves inception and FID scores on different architectures and for different GAN objectives. We provide comprehensive experimental results across a range of datasets, bilinear games, mixture of Gaussians, CIFAR-10, STL-10, CelebA and ImageNet, to demonstrate its effectiveness. We achieve state-of-the-art results on CIFAR-10 and produce clean CelebA face images, demonstrating that averaging is one of the most effective techniques for training highly performant GANs.
更多查看译文
AI 理解论文
溯源树
样例
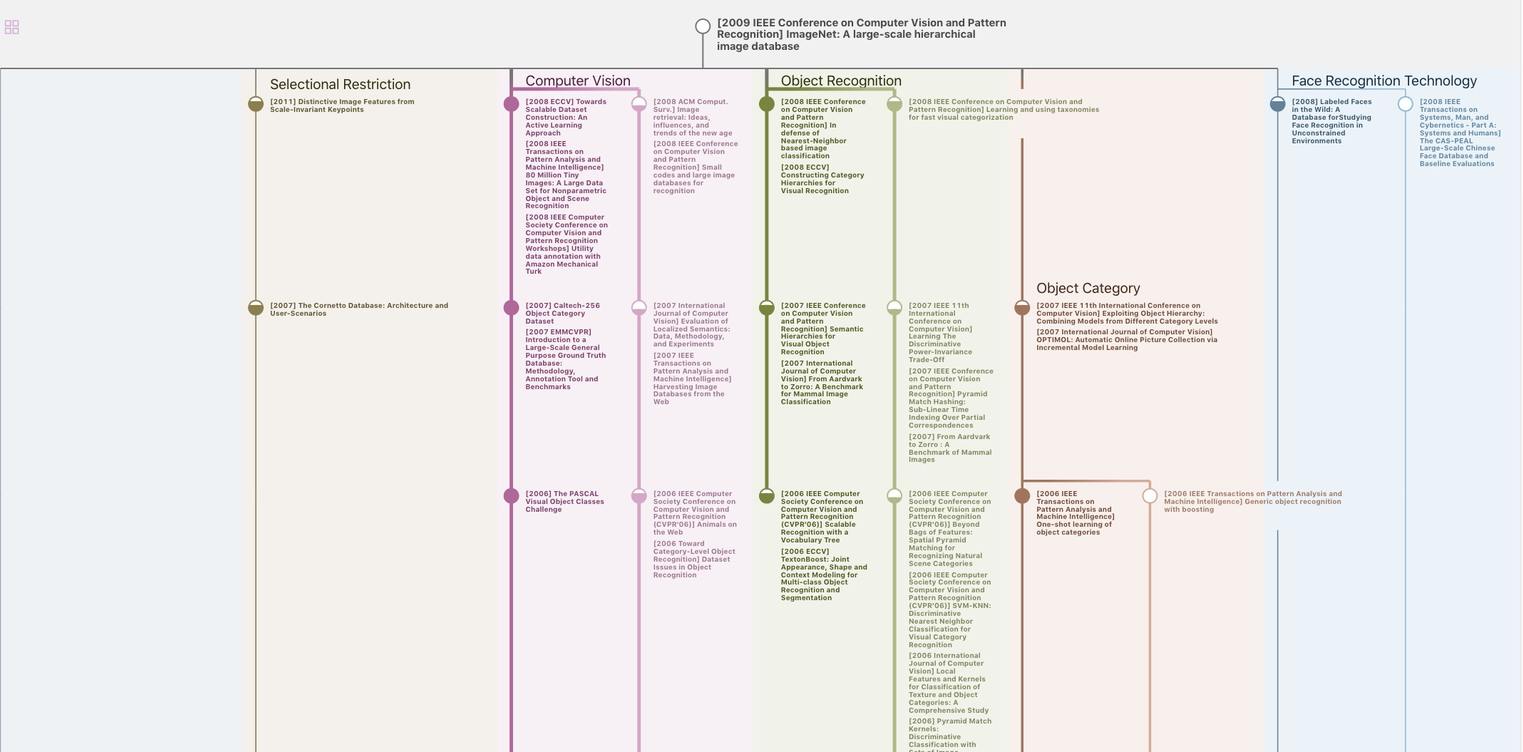
生成溯源树,研究论文发展脉络
Chat Paper
正在生成论文摘要