Ensemble Pruning Based on Objection Maximization With a General Distributed Framework
IEEE Transactions on Neural Networks and Learning Systems(2020)
Abstract
Ensemble pruning, selecting a subset of individual learners from an original ensemble, alleviates the deficiencies of ensemble learning on the cost of time and space. Accuracy and diversity serve as two crucial factors, while they usually conflict with each other. To balance both of them, we formalize the ensemble pruning problem as an objection maximization problem based on information entropy. Then we propose an ensemble pruning method, including a centralized version and a distributed version, in which the latter is to speed up the former. Finally, we extract a general distributed framework for ensemble pruning, which can be widely suitable for most of the existing ensemble pruning methods and achieve less time-consuming without much accuracy degradation. Experimental results validate the efficiency of our framework and methods, particularly concerning a remarkable improvement of the execution speed, accompanied by gratifying accuracy performance.
MoreTranslated text
Key words
Composable core-sets,diversity,ensemble learning,ensemble pruning,information entropy
AI Read Science
Must-Reading Tree
Example
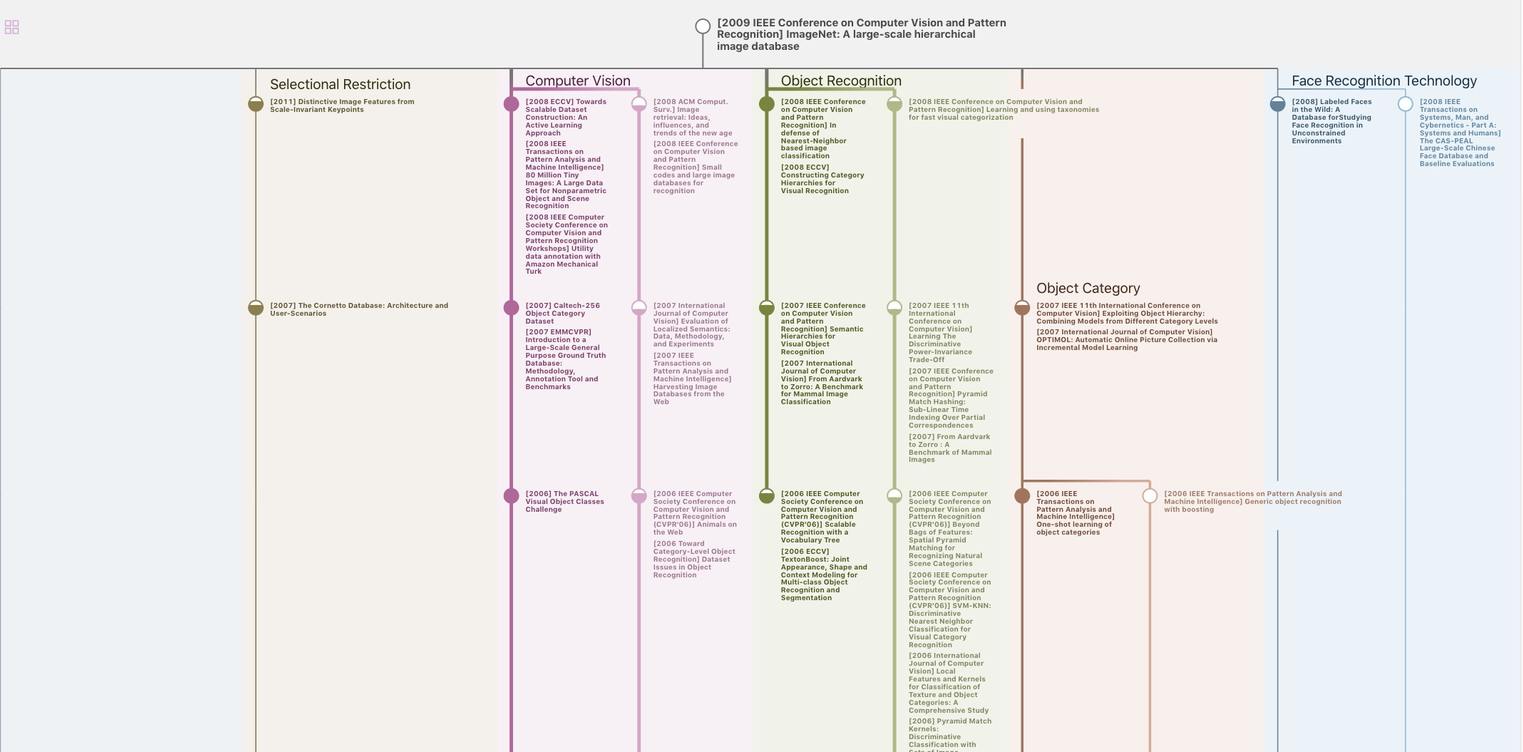
Generate MRT to find the research sequence of this paper
Chat Paper
Summary is being generated by the instructions you defined