A Comparative Study on Unsupervised Domain Adaptation for Coffee Crop Mapping.
arXiv: Computer Vision and Pattern Recognition(2018)
摘要
In this work, we investigate the application of existing unsupervised domain adaptation (UDA) approaches to the task of transferring knowledge between crop regions having different coffee patterns. Given a geographical region with fully mapped coffee plantations, we observe that this knowledge can be used to train a classifier and to map a new county with no need of samples indicated in the target region. Experimental results show that transferring knowledge via UDA strategies performs better than just applying a classifier trained in a region to predict coffee crops in a new one. However, UDA methods may lead to negative transfer, which may indicate that domains are excessively dissimilar, rendering transfer strategies ineffective. We observe a meaningful complementary contribution between coffee crop data; and a visual behavior suggests the existence of clusters of samples that are more likely to be drawn from a specific data.
更多查看译文
关键词
Unsupervised domain adaptation, Remote sensing, Transfer knowledge, Coffee crops
AI 理解论文
溯源树
样例
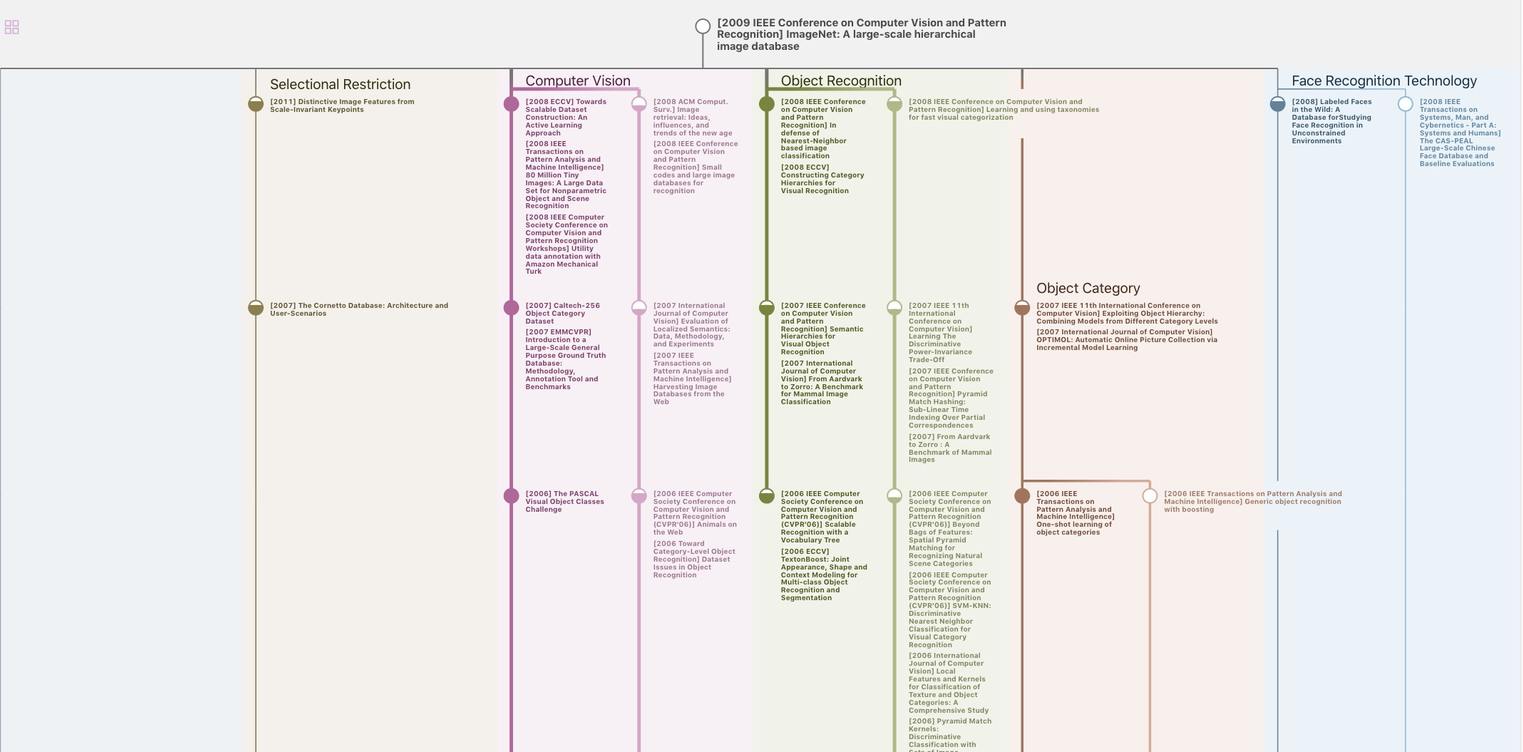
生成溯源树,研究论文发展脉络
Chat Paper
正在生成论文摘要