Inform: Efficient Information-Theoretic Analysis of Collective Behaviors.
FRONTIERS IN ROBOTICS AND AI(2018)
摘要
The study of collective behavior has traditionally relied on a variety of different methodological tools ranging from more theoretical methods such as population or game-theoretic models to empirical ones like Monte Carlo or multi-agent simulations. An approach that is increasingly being explored is the use of information theory as a methodological framework to study the flow of information and the statistical properties of collectives of interacting agents. While a few general purpose toolkits exist, most of the existing software for information theoretic analysis of collective systems is limited in scope. We introduce Inform, an open-source framework for efficient information theoretic analysis that exploits the computational power of a C library while simplifying its use through a variety of wrappers for common higher-level scripting languages. We focus on two such wrappers here: PyInform (Python) and inform (R). Inform and its wrappers are cross-platform and general-purpose. They include classical information-theoretic measures, measures of information dynamics and information-based methods to study the statistical behavior of collective systems, and expose a lower-level API that allow users to construct measures of their own. We describe the architecture of the Inform framework, study its computational efficiency and use it to analyze three different case studies of collective behavior: biochemical information storage in regenerating planaria, nest-site selection in the ant Temnothorax rugatulus, and collective decision making in multiagent simulations.
更多查看译文
关键词
information transfer,information storage,information dynamics,complex systems,collective behavior,information theory
AI 理解论文
溯源树
样例
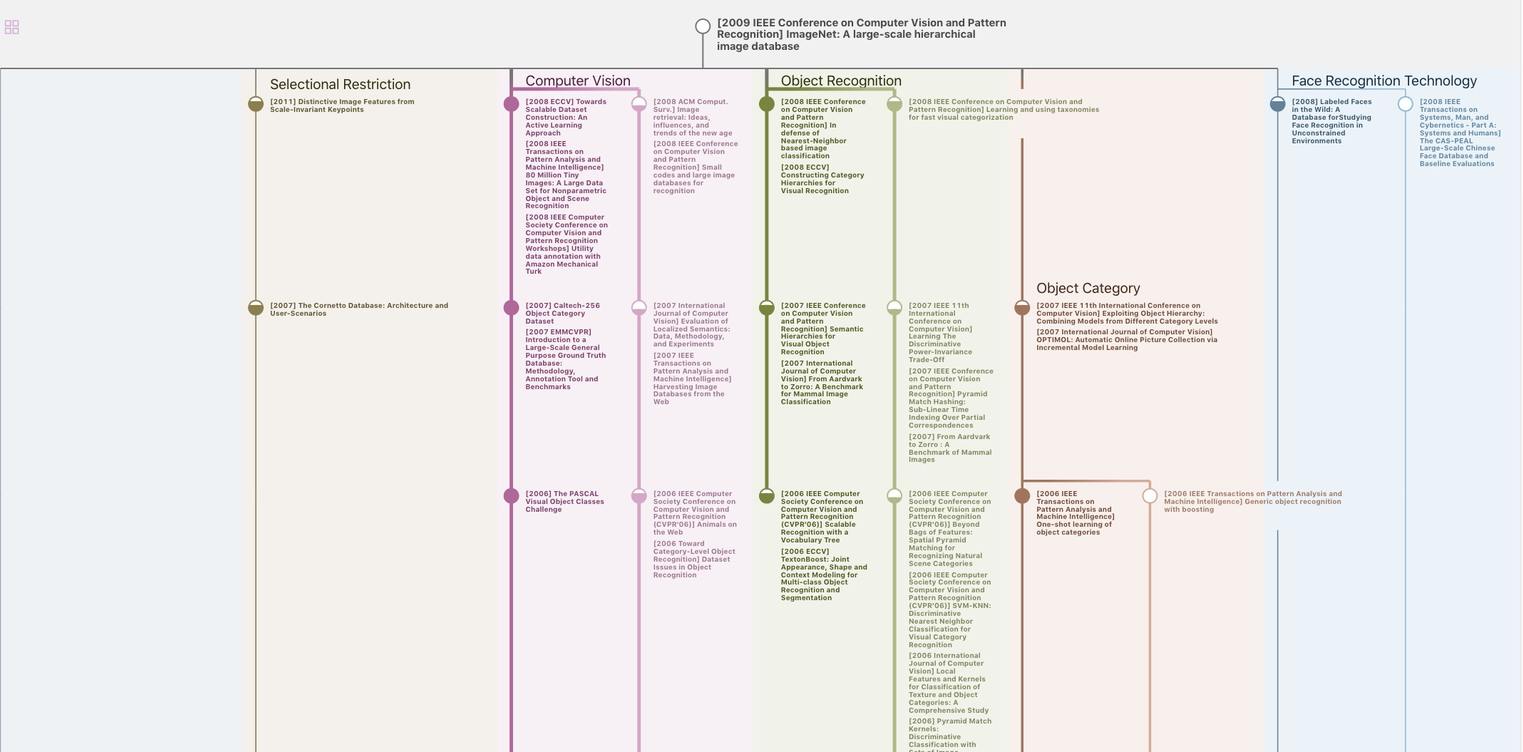
生成溯源树,研究论文发展脉络
Chat Paper
正在生成论文摘要