Noisy Low-Tubal-Rank Tensor Completion Through Iterative Singular Tube Thresholding.
IEEE ACCESS(2018)
摘要
In many applications, data organized in tensor form contains noise and missing entries. In this paper, the goal is to complete a tensor from its partial noisy observations. Specifically, we consider low-tubal-rank tensors sampled by element-wise Bernoulli sampling with additive sub-exponential noise. Algorithmically, a soft-impute-like algorithm, namely iterative singular tube thresholding (ISTT), is proposed. Statistically, bound on the estimation error of ISTT is explored. First, the estimation error is upper bounded non-asymptotically. Then, the minimax optimal lower bound of the estimation error for tensors with limited tubal-rank and bounded l infinity-norm is established, indicating that the proposed upper bound is order optimal up to a logarithm factor. Numerical simulations show that the proposed upper bound can precisely predict the scaling behavior of the estimation error of ISTT. Compared with several state-of-the-art convex algorithms, the effectiveness of ISTT is demonstrated through experiments on real-world data sets.
更多查看译文
关键词
Tensor completion,tensor SVD,soft-impute,multidimensional signal processing
AI 理解论文
溯源树
样例
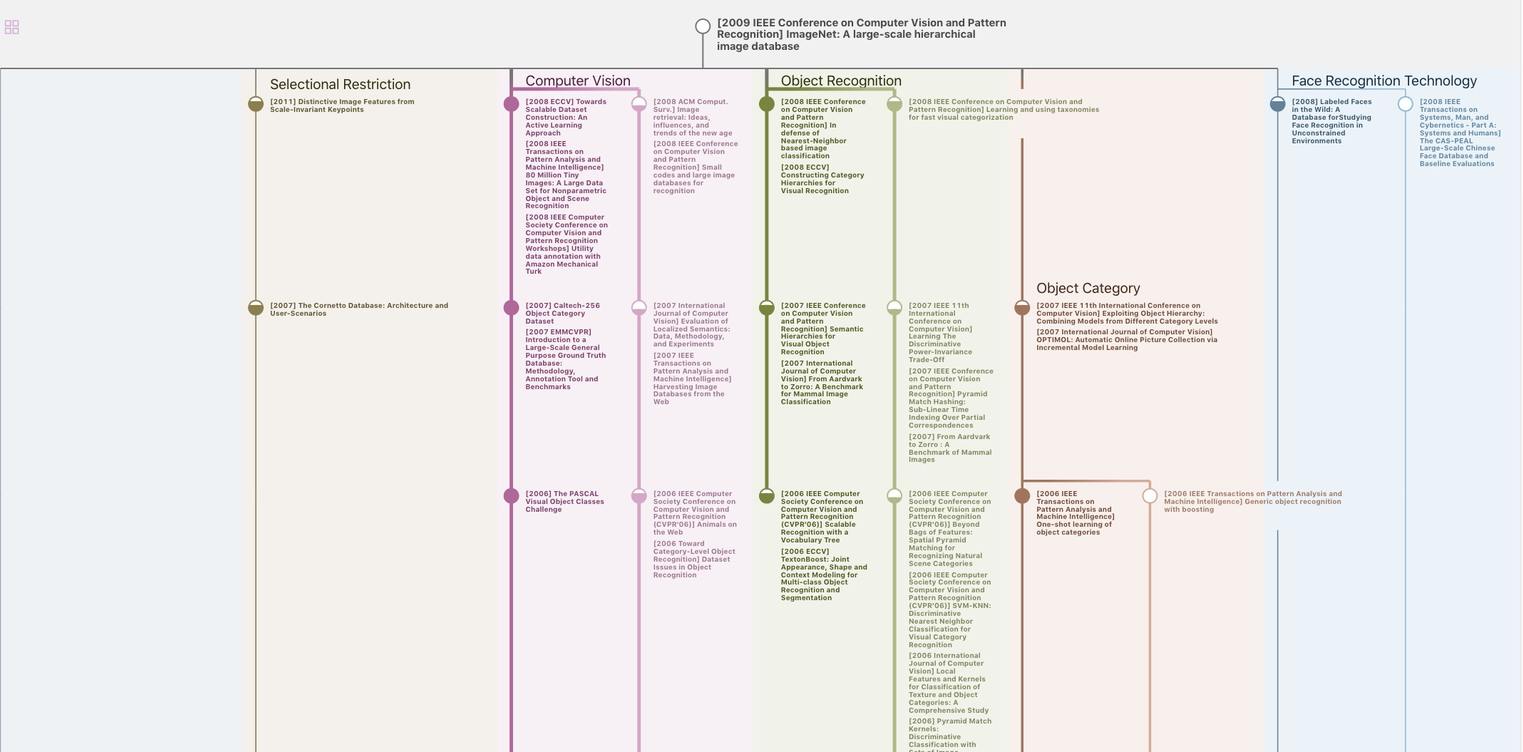
生成溯源树,研究论文发展脉络
Chat Paper
正在生成论文摘要