Near-Optimal Bounds for Phase Synchronization.
SIAM JOURNAL ON OPTIMIZATION(2018)
摘要
The problem of estimating the phases (angles) of a complex unit-modulus vector z from their noisy pairwise relative measurements C = zz* + sigma W, where W is a complex-valued Gaussian random matrix, is known as phase synchronization. The maximum likelihood estimator (MLE) is a solution to a unit{modulus-constrained quadratic programming problem, which is nonconvex. Existing works have proposed polynomial-time algorithms such as a semidefinite programming (SDP) relaxation or the generalized power method (GPM). Numerical experiments suggest that both of these methods succeed with high probability for sigma up to <(O)overtilde>(n(1/2)), yet existing analyses only confirm this observation for sigma up to <(O)overtilde>(n(1/2)). In this paper, we bridge the gap by proving that the SDP relaxation is tight for sigma = O(root n/log n), and GPM converges to the global optimum under the same regime. Moreover, we establish a linear convergence rate for GPM, and derive a tighter l(infinity) bound for the MLE. A novel technique we develop in this paper is to (theoretically) track n closely related sequences of iterates, in addition to the sequence of iterates GPM actually produces. As a by-product, we obtain an l(infinity) perturbation bound for leading eigenvectors. Our result also confirms predictions that use techniques from statistical mechanics.
更多查看译文
关键词
angular synchronization,nonconvex optimization,semidefinite programming relaxation,projected power method,quadratically constrained quadratic program,maximum likelihood estimator,eigenvector perturbation bound
AI 理解论文
溯源树
样例
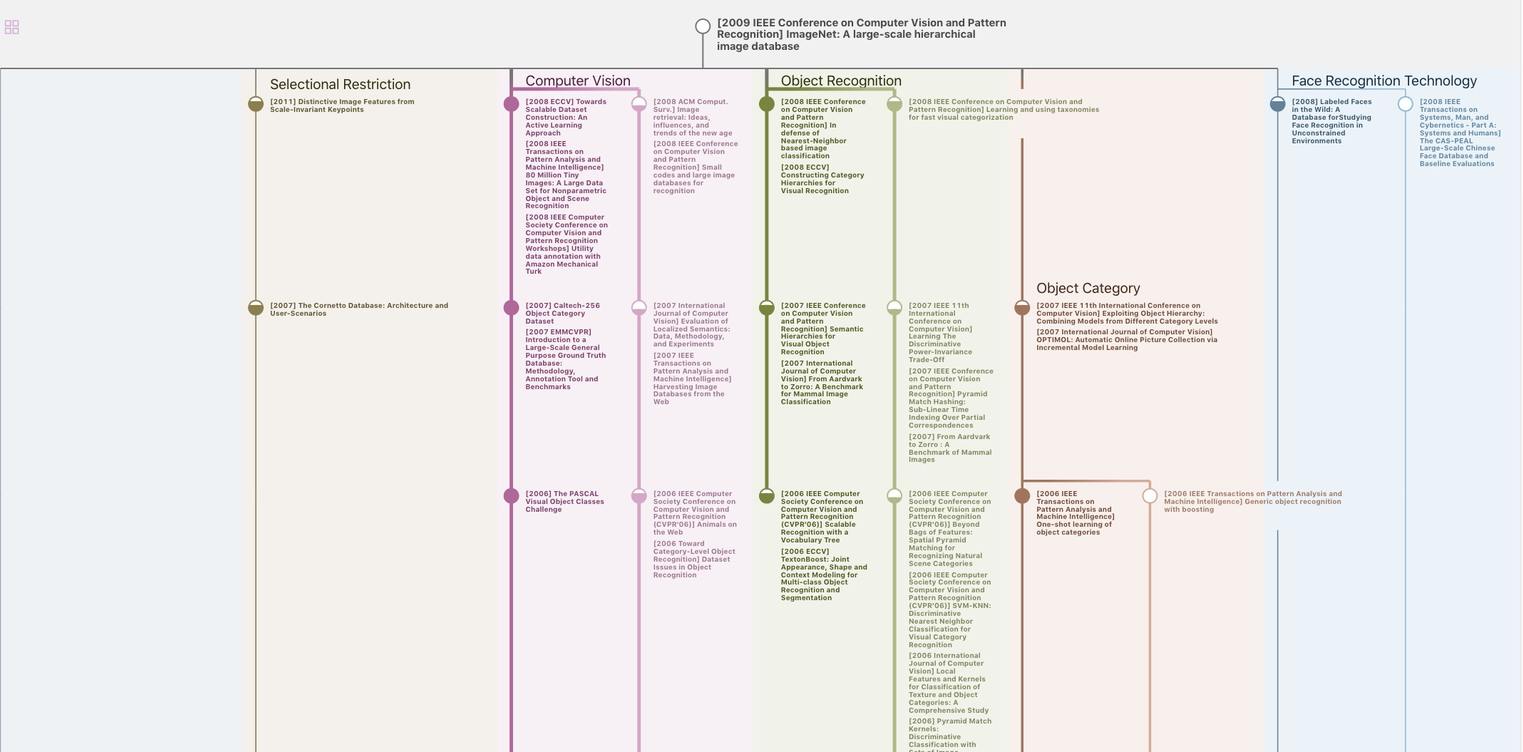
生成溯源树,研究论文发展脉络
Chat Paper
正在生成论文摘要