Algorithmic Regularization in Over-parameterized Matrix Sensing and Neural Networks with Quadratic Activations.
COLT(2018)
摘要
We show that the (stochastic) gradient descent algorithm provides an implicit regularization effect the learning of over-parameterized matrix factorization models and one-hidden-layer neural networks with quadratic activations. Concretely, we show that given (tilde{O}(dr^{2})) random linear measurements of a rank (r) positive semidefinite matrix (X^*), we can recover (X^*) by parameterizing it by (UU^top) with (U in mathbb{R}^{dtimes d}) and minimizing the squared loss, even if (r) is much less than (d). We prove that starting from a small initialization, gradient descent recovers (X^*) (tilde{O}(sqrt{r})) iterations approximately. The results solve the conjecture of Gunasekar et al. 17 under the restricted isometry property.The technique can be applied to analyzing neural networks with quadratic activations with some technical modifications.
更多查看译文
AI 理解论文
溯源树
样例
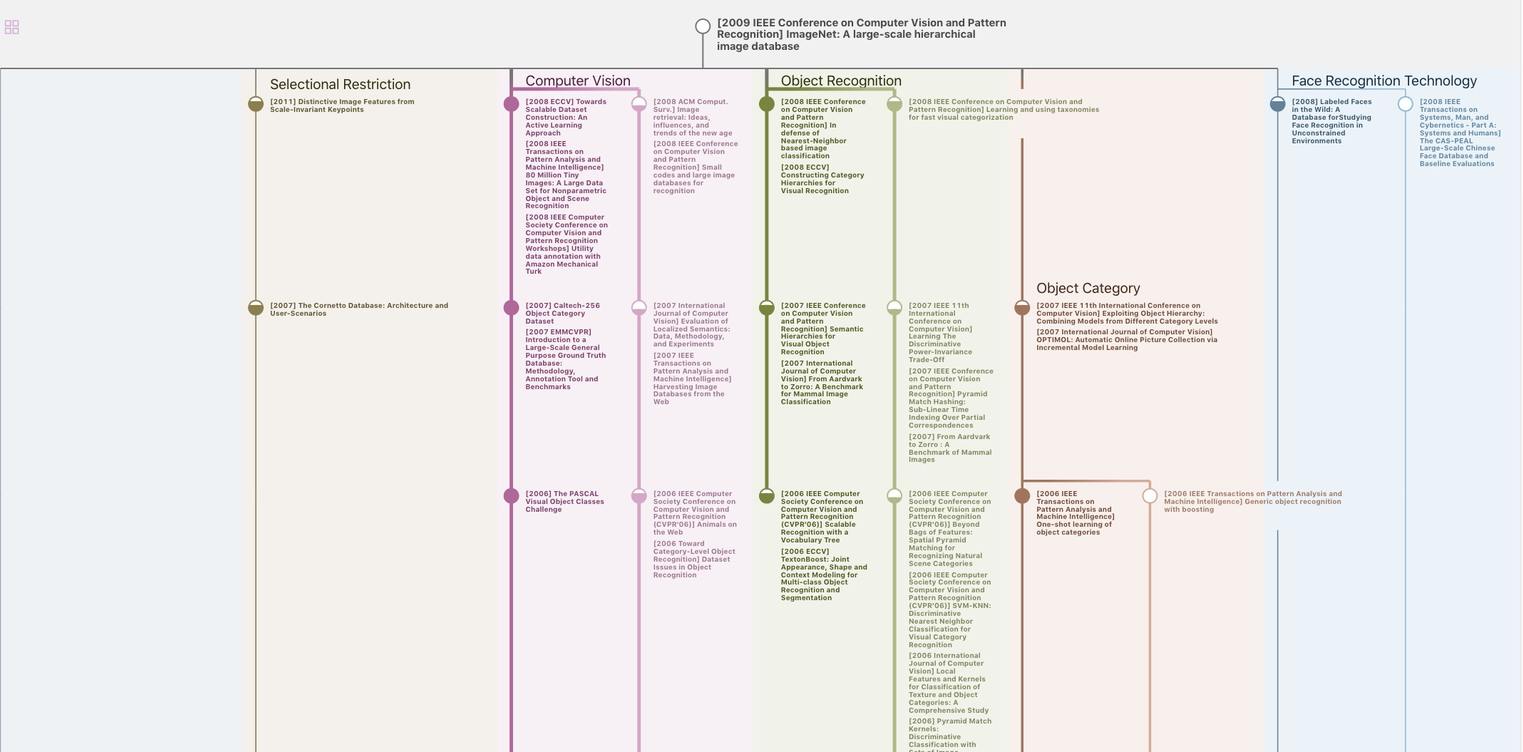
生成溯源树,研究论文发展脉络
Chat Paper
正在生成论文摘要