Deep Bayesian Nonparametric Tracking.
ICML(2018)
摘要
Time-series data often exhibit irregular behavior, making them hard to analyze and explain with a simple dynamic model. For example, information in social networks may show change-point-like bursts that then diffuse with smooth dynamics. Powerful models such as deep neural networks learn smooth functions from data, but are not as well-suited (in off-the-shelf form) for discovering and explaining sparse, discrete and bursty dynamic patterns. Bayesian models can do this well by encoding the appropriate probabilistic assumptions in the model prior. We propose an integration of Bayesian nonparametric methods within deep neural networks for modeling irregular patterns in time-series data. We use a Bayesian nonparametrics to model change-point behavior in time, and a deep neural network to model nonlinear latent space dynamics. We compare with a non-deep linear version of the model also proposed here. Empirical evaluations demonstrates improved performance and interpretable results when tracking stock prices and Twitter trends.
更多查看译文
关键词
deep bayesian nonparametric tracking
AI 理解论文
溯源树
样例
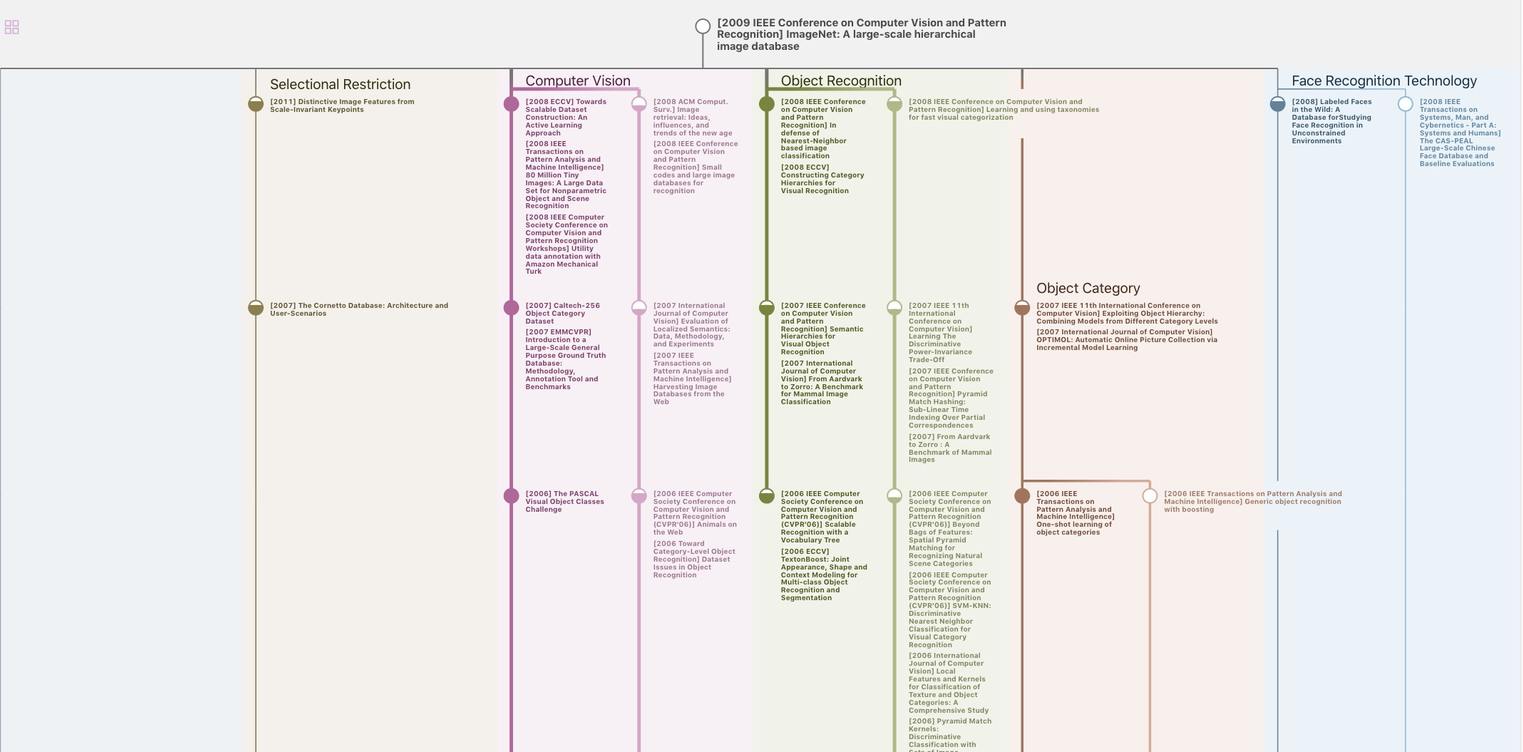
生成溯源树,研究论文发展脉络
Chat Paper
正在生成论文摘要