A Boo(n) for Evaluating Architecture Performance.
international conference on machine learning(2018)
摘要
We point out several important problems with the common practice of using the best single model performance for comparing Deep Learning architectures, and we propose a method that corrects these flaws. Each time a model is trained, one gets a different result due to random factors in the training process, which include random parameter initialization and random data shuffling. Reporting the best single model performance does not appropriately deal with this stochasticity. Furthermore, the expected best result increases with the number of experiments run, among other problems. We propose a normalized expected best-out-of-n performance (Boo_n) as a way to correct these problems.
更多查看译文
关键词
evaluating architecture performance
AI 理解论文
溯源树
样例
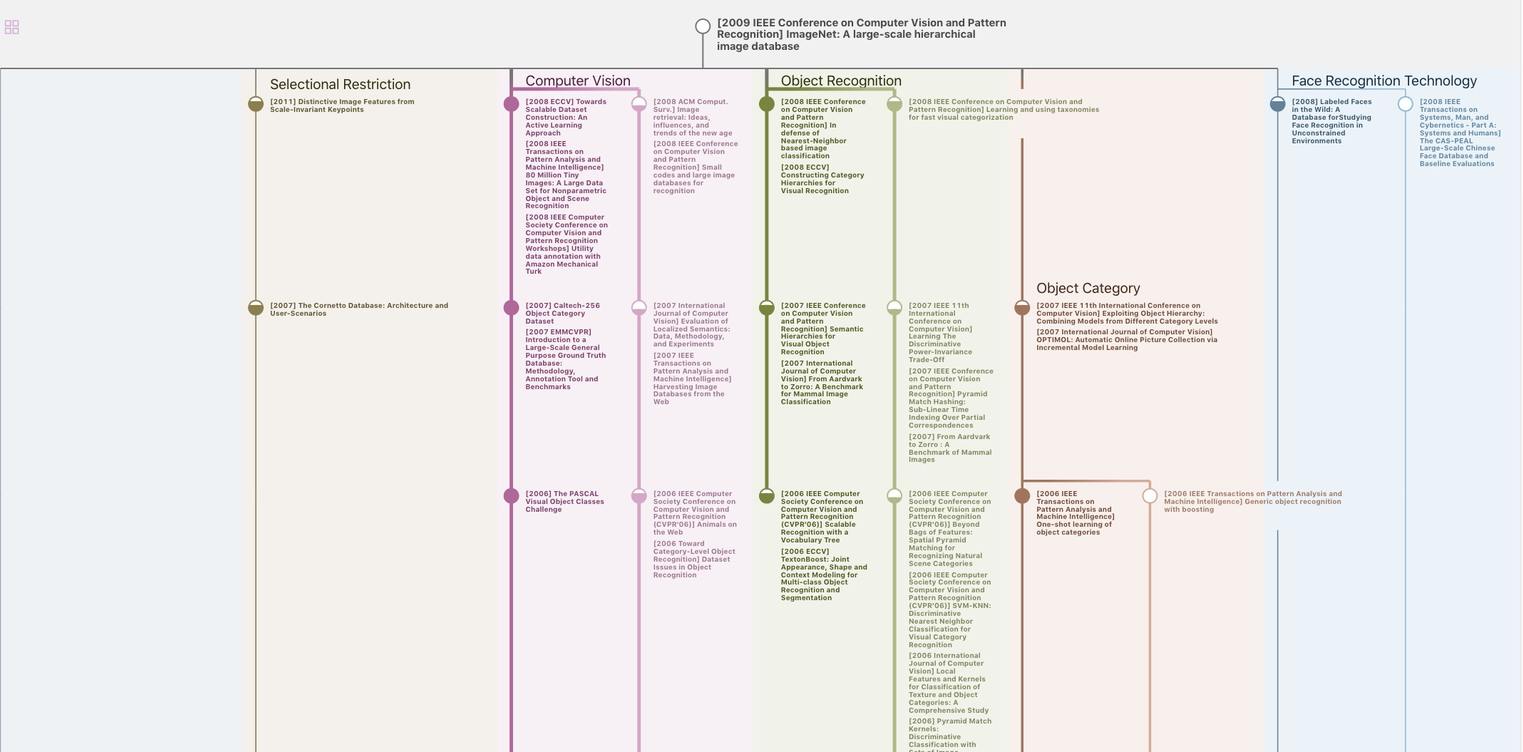
生成溯源树,研究论文发展脉络
Chat Paper
正在生成论文摘要