Applying Subsequence Matching to Collaborative Filtering: Extended Abstract.
CERI(2018)
摘要
Neighbourhood-based approaches, although they are one of the most popular strategies in the recommender systems area, continue using classic similarities that leave aside the sequential information of the users interactions. In this extended abstract, we summarise the main contributions of our previous work where we proposed to use the Longest Common Subsequence algorithm as a similarity measure between users, by adapting it to the recommender systems context and proposing a mechanism to transform users interactions into sequences. Furthermore, we also introduced some modifications on the original LCS algorithm to allow non-exact matchings between users and to bound the similarities obtained in the [0,1] interval. Our reported results showed that our LCS-based similarity was able to outperform different state-of-the-art recommenders in two datasets in both ranking and novelty and diversity metrics.
更多查看译文
AI 理解论文
溯源树
样例
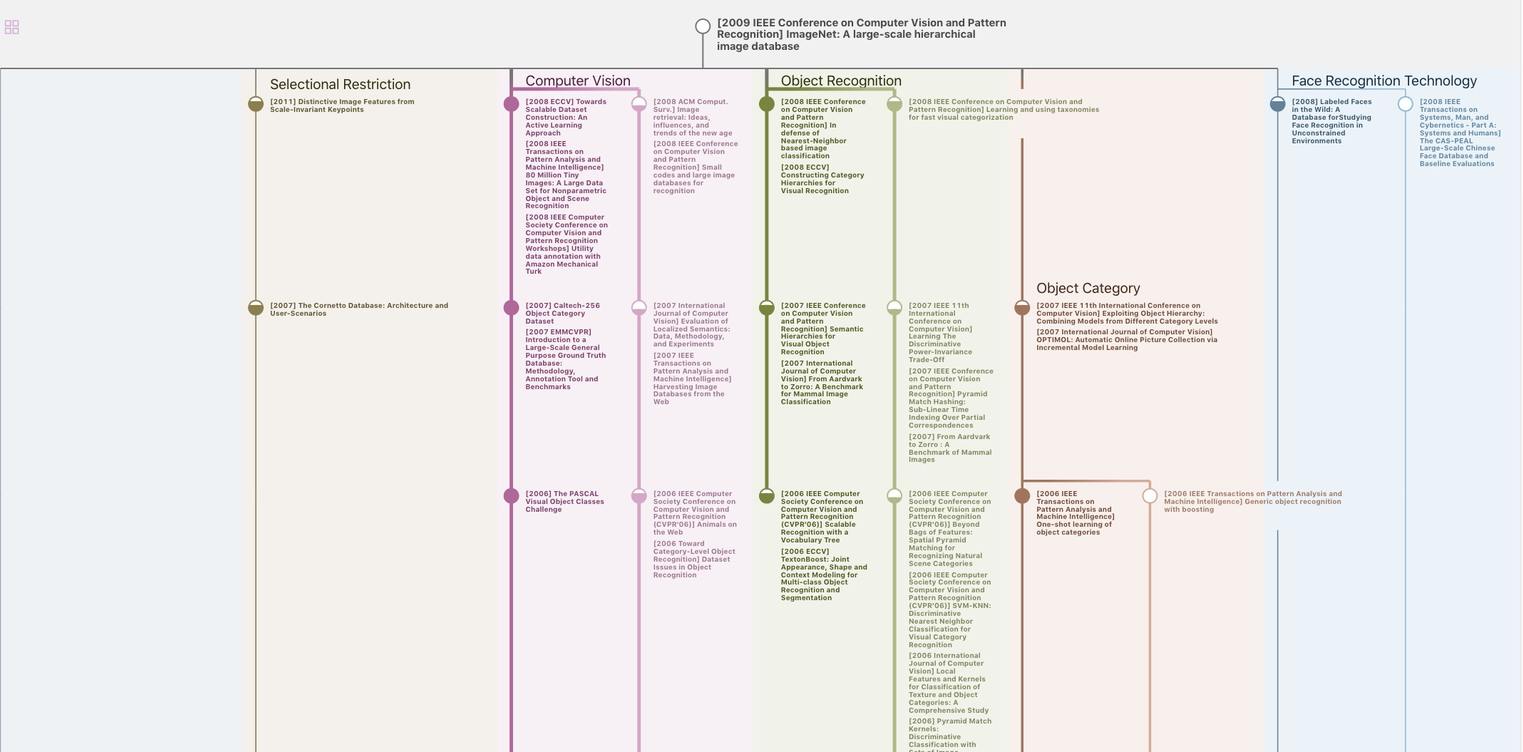
生成溯源树,研究论文发展脉络
Chat Paper
正在生成论文摘要