Understanding Behaviors in Different Domains: The Role of Machine Learning Techniques and Network Science.
HCI(2018)
摘要
Recent developments in the Internet of Things (IoT), social media, and the data sciences have resulted in larger volumes of data than ever before, offering more opportunity for observing and understanding behaviors. Advances in data analytic and machine learning techniques have also enabled assessments to be more multi-faceted, incorporating data from more sources. Machine learning algorithms such as Decision Trees and Random Forests, K-nearest neighbors, and Artificial Neural Networks have been used to uncover hidden patterns in data and derive predictions and recommendations from a wide range of data types and sources. However, these do not necessarily yield insights into behaviors in complex systems/domains. Methods from mathematics such as Set Theory, Graph Theory, and Network Science may be useful in shedding light on the interactions and relationships within and across domains. This paper provides a description of the applications, strengths, and limitations of some of these techniques and methods.
更多查看译文
关键词
Machine learning techniques, Decision tree, Random forest, K-nearest neighbor, Artificial Neural Network, Network science
AI 理解论文
溯源树
样例
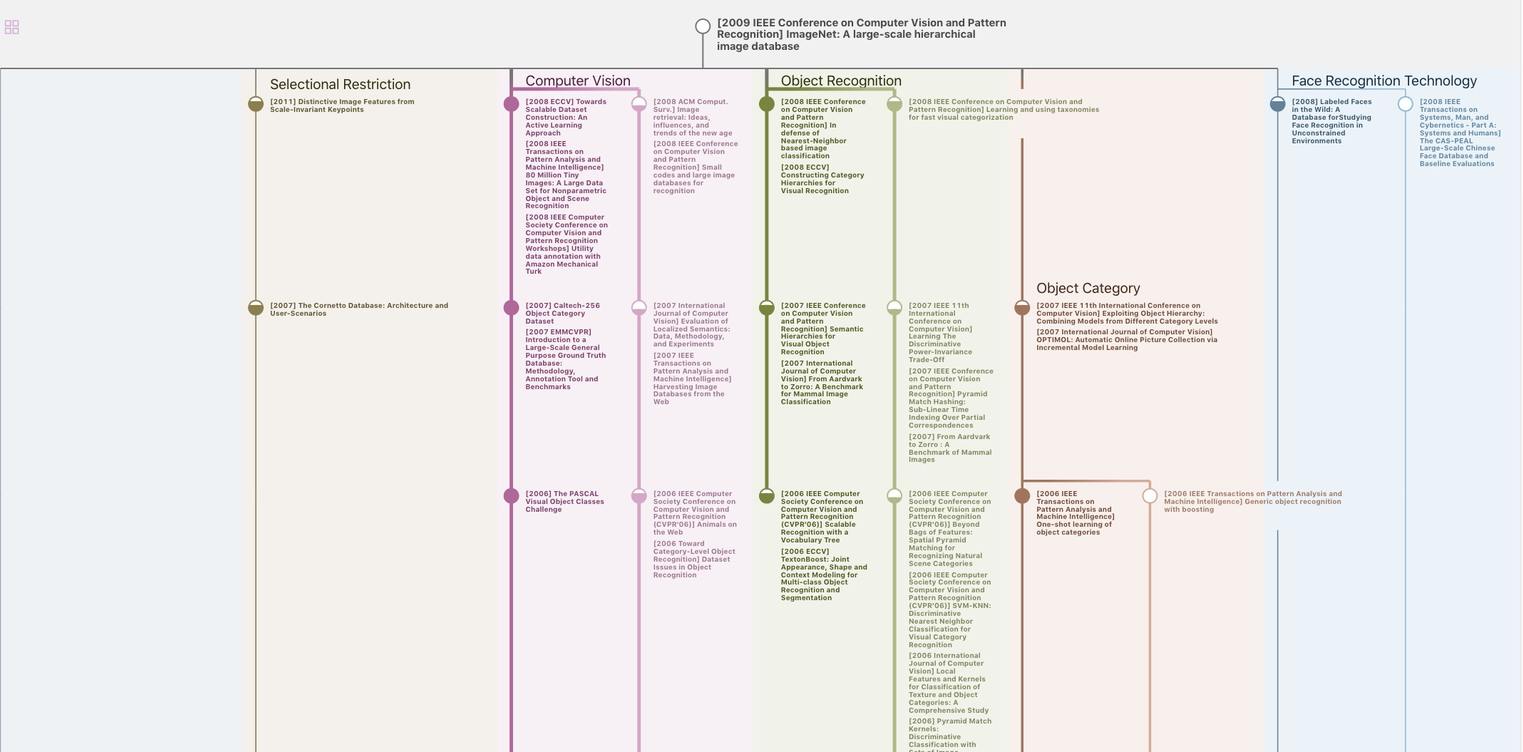
生成溯源树,研究论文发展脉络
Chat Paper
正在生成论文摘要