Drug Target Interaction Predictions Using Pu-Learning Under Different Experimental Setting For Four Formulations Namely Known Drug Target Pair Prediction, Drug Prediction, Target Prediction And Unknown Drug Target Pair Prediction
2018 IEEE CONFERENCE ON COMPUTATIONAL INTELLIGENCE IN BIOINFORMATICS AND COMPUTATIONAL BIOLOGY (CIBCB)(2018)
摘要
Predicting new drug target interactions experimentally through wet lab experiments is time as well as resource intensive. In general, drug-target interaction prediction problem leads to drug discovery, drug repositioning and uncovers interesting patterns in chemogenomics research. Drug and target represent heterogeneous nodes within a network of interactions. Presence of an edge between the nodes indicates a positive interaction whereas an absence suggests an unknown interaction. Classification based machine learning algorithms are heavily applied in this area of research. Classification algorithms need positive as well as negative data to yield optimized results. The major problem in this field is lack of negative data because the data that are found in the public databases are positive interaction samples. Considering unknown drug target pairs as negative data may cause severe consequences for the prediction performance. Thereby, we propose a positive un-labelled (PU) learning-based approach that uses one class support vector machine technique as the learning algorithm. The algorithm learns the positive distribution from the unified feature vector space of drugs and targets and regards unknown pairs as unlabeled instead of labelling them as negative pairs. Additionally, we use 4860 Klekota Roth fingerprint + 881 PubChem fingerprint as a high dimensional and highly discriminative feature vector representation for drugs. To represent protein features, we create a protein-motif matrix based on the sliding window score that records the probability of a motif pattern occurring within a given protein sequence. Also, we separately evaluate the prediction performance using 5-fold nested cross-validation under different experimental setting for each of the four formulations: 1) Known drug-target pair, 2) Drug prediction, 3) Target prediction and 4) Unknown drug target pair. We show that our approach yields the best AUC score over previous benchmark techniques and outperforms most of the recent works based on one class classifiers and PU-based learning.
更多查看译文
关键词
Drug target prediction,Support vector machine,Positive un-labelled learning,One class classifier,Chemical fingerprint,Protein motif
AI 理解论文
溯源树
样例
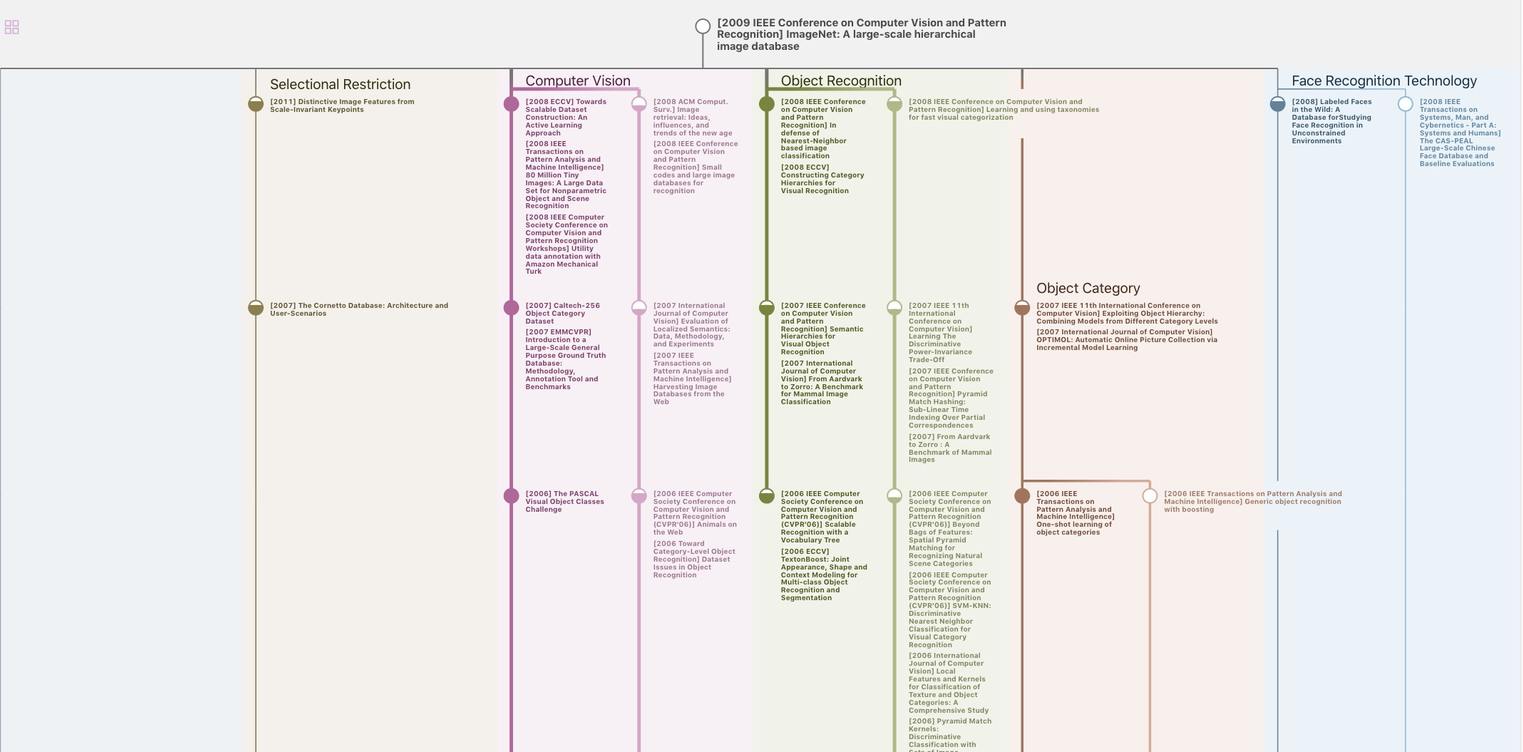
生成溯源树,研究论文发展脉络
Chat Paper
正在生成论文摘要