Improved Localisation Using Spatio-Temporal Data from Cellular Network
2018 19th IEEE International Conference on Mobile Data Management (MDM)(2018)
Abstract
Localisation of mobile devices has been a topic of academic research and industry practice for solving various application problems, examples can be footfall counting and profiling used for location based digital or physical advertising, crowd monitoring for public security, emergency handling, transport measurement and management, etc. Often the solutions for localisation require large scale networks hardware and/or software upgrades, which can be very costly. However, we note the fact that many commercial use cases actually do not require very high resolution of localisation and satisfactory level of accuracy may be sufficient for attaining business decision quality. A reasonable trade-off between achieving business value and minimising additional costs on network equipment purchase and maintenance is to build solutions that rely only on telco-network data and utilize data mining methods to improve the localisation accuracy of mobile devices that carried by subscribers. In this work, we aim to achieve acceptable accuracy for localisation at the resolution of region of interest (ROI), the exact shape of which is defined according to business requirements. One example is the geographical division of planning sub-zone in Singapore. We make use of the Global Positioning System (GPS) locations extracted from mobile broadband log that contain the longitude and latitude of the subscriber to annotate the telco-network data. We experimented with three learning models: maximum likelihood estimation, dominant serving ROI, and random forest, along with the baseline of localisation based on cellular tower locations. The experiment results demonstrate the effectiveness of the proposed models and demonstrate accuracy improvement from baseline of 37.8% (naive cellular tower localisation) to 78.4% (random forest classification).
MoreTranslated text
Key words
cellular networks,localisation,spatio temporal data
AI Read Science
Must-Reading Tree
Example
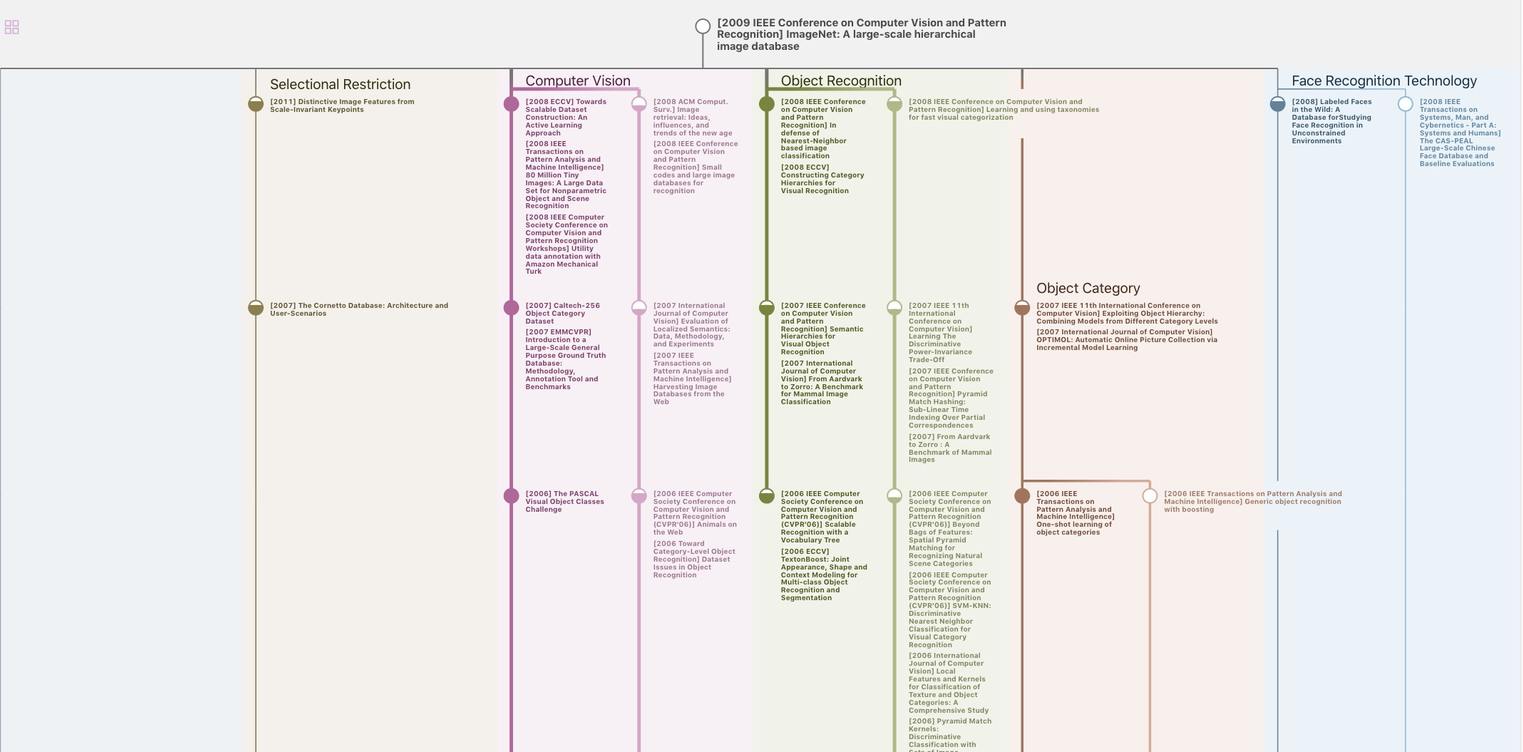
Generate MRT to find the research sequence of this paper
Chat Paper
Summary is being generated by the instructions you defined