Incomplete Multi-View Weak-Label Learning.
IJCAI(2018)
摘要
Learning from multi-view multi-label data has wide applications. Two main challenges characterize this learning task: incomplete views and missing (weak) labels. The former assumes that views may not include all data objects. The weak label setting implies that only a subset of relevant labels are provided for training objects while other labels are missing. Both incomplete views and weak labels can lead to significant performance degradation. In this paper, we propose a novel model (iMVWL) to jointly address the two challenges. iMVWL learns a shared subspace from incomplete views with weak labels, local label correlations, and a predictor in this subspace, simultaneously. The latter can capture not only cross-view relationships but also weak-label information of training samples. We further develop an alternative solution to optimize our model; this solution can avoid suboptimal results and reinforce their reciprocal effects, and thus further improve the performance. Extensive experimental results on real-world datasets validate the effectiveness of our model against other competitive algorithms.
更多查看译文
AI 理解论文
溯源树
样例
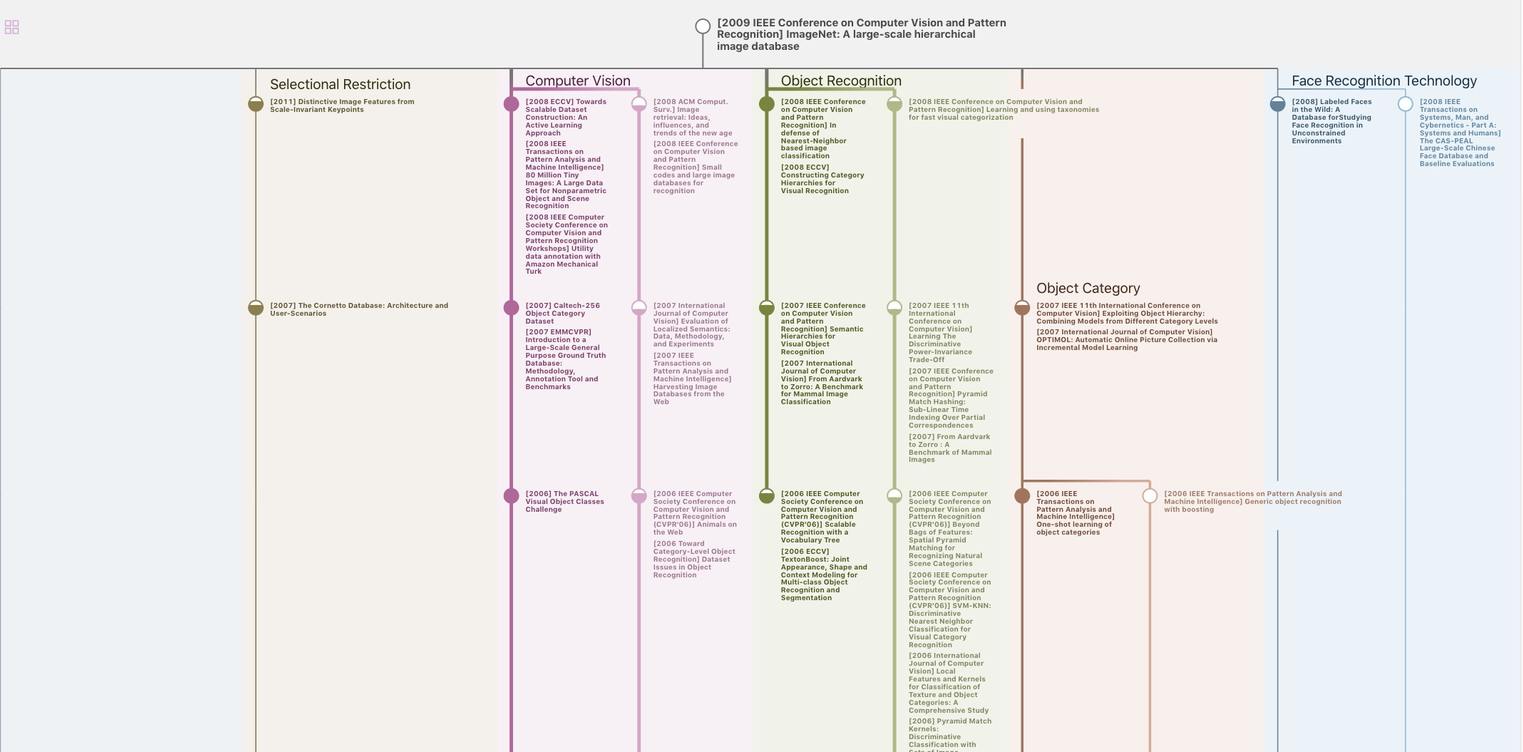
生成溯源树,研究论文发展脉络
Chat Paper
正在生成论文摘要