Goal-HSVI: Heuristic Search Value Iteration for Goal-POMDPs
PROCEEDINGS OF THE TWENTY-SEVENTH INTERNATIONAL JOINT CONFERENCE ON ARTIFICIAL INTELLIGENCE(2018)
Abstract
Partially observable Markov decision processes (POMDPs) are the standard models for planning under uncertainty with both finite and infinite horizon. Besides the well-known discounted-sum objective, indefinite-horizon objective (aka Goal-POMDPs) is another classical objective for POMDPs. In this case, given a set of target states and a positive cost for each transition, the optimization objective is to minimize the expected total cost until a target state is reached. In the literature, RTDP-Bel or heuristic search value iteration (HSVI) have been used for solving Goal-POMDPs. Neither of these algorithms has theoretical convergence guarantees, and HSVI may even fail to terminate its trials. We give the following contributions: (1) We discuss the challenges introduced in Goal-POMDPs and illustrate how they prevent the original HSVI from converging. (2) We present a novel algorithm inspired by HSVI, termed Goal-HSVI, and show that our algorithm has convergence guarantees. (3) We show that Goal-HSVI outperforms RTDP-Bel on a set of well-known examples.
MoreTranslated text
Key words
heuristic search value iteration,goal-hsvi
AI Read Science
Must-Reading Tree
Example
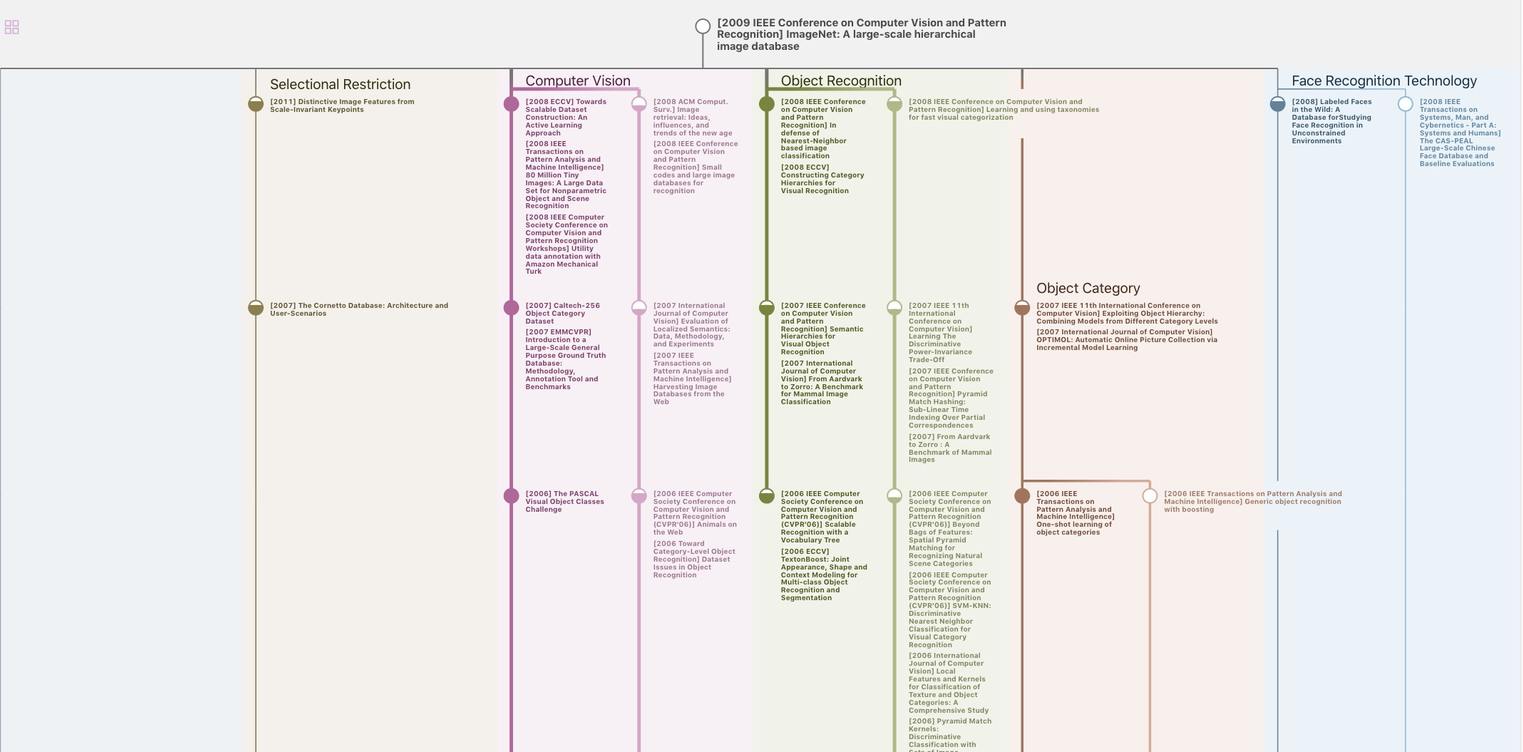
Generate MRT to find the research sequence of this paper
Chat Paper
Summary is being generated by the instructions you defined