K8-scalar: a workbench to compare autoscalers for container-orchestrated database clusters.
SEAMS@ICSE(2018)
摘要
Although a considerable amount of research exists on auto-scaling of database clusters, the design of an effective auto-scaling strategy requires fine-grained tailoring towards the specific application scenario.
This paper presents an easy-to-use and extensible workbench exemplar, named K8-Scalar (Kube-Scalar), which allows researchers to implement and evaluate different self-adaptive approaches to auto-scaling container-orchestrated services. The workbench is based on Docker, a popular technology for easing the deployment of containerized software that also has been positioned as an enabler for reproducible research. The workbench also relies on a container orchestration framework: Kubernetes (K8s), the de-facto industry standard for orchestration and monitoring of elastically scalable container-based services. Finally, it integrates and extends Scalar, a generic testbed for evaluating the scalability of large-scale systems with support for evaluating the performance of autoscalers for database clusters.
The paper discusses (i) the architecture and implementation of K8-Scalar and how a particular autoscaler can be plugged in, (ii) sketches the design of a Riemann-based autoscaler for database clusters, (iii) illustrates how to design, setup and analyze a series of experiments to configure and evaluate the performance of this autoscaler for a particular database (i.e., Cassandra) and a particular workload type, and (iv) validates the effectiveness of K8-Scalar as a workbench for accurately comparing the performance of different auto-scaling strategies.
更多查看译文
关键词
Container orchestration, Autoscaling, Experimentation exemplar
AI 理解论文
溯源树
样例
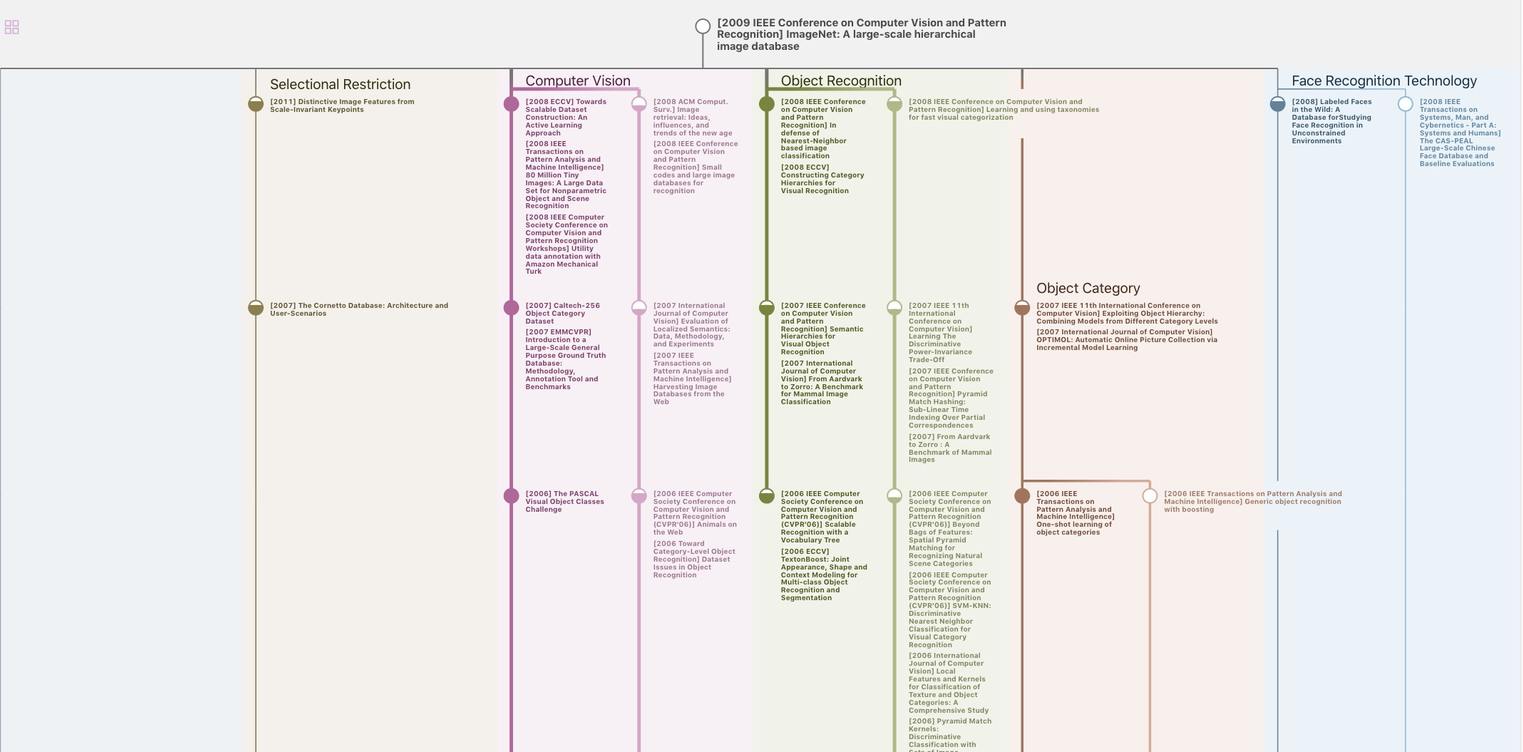
生成溯源树,研究论文发展脉络
Chat Paper
正在生成论文摘要