Approximate structure learning for large Bayesian networks
Machine Learning(2018)
摘要
We present approximate structure learning algorithms for Bayesian networks. We discuss the two main phases of the task: the preparation of the cache of the scores and structure optimization, both with bounded and unbounded treewidth. We improve on state-of-the-art methods that rely on an ordering-based search by sampling more effectively the space of the orders. This allows for a remarkable improvement in learning Bayesian networks from thousands of variables. We also present a thorough study of the accuracy and the running time of inference, comparing bounded-treewidth and unbounded-treewidth models.
更多查看译文
关键词
Bayesian networks,Structural learning,Treewidth
AI 理解论文
溯源树
样例
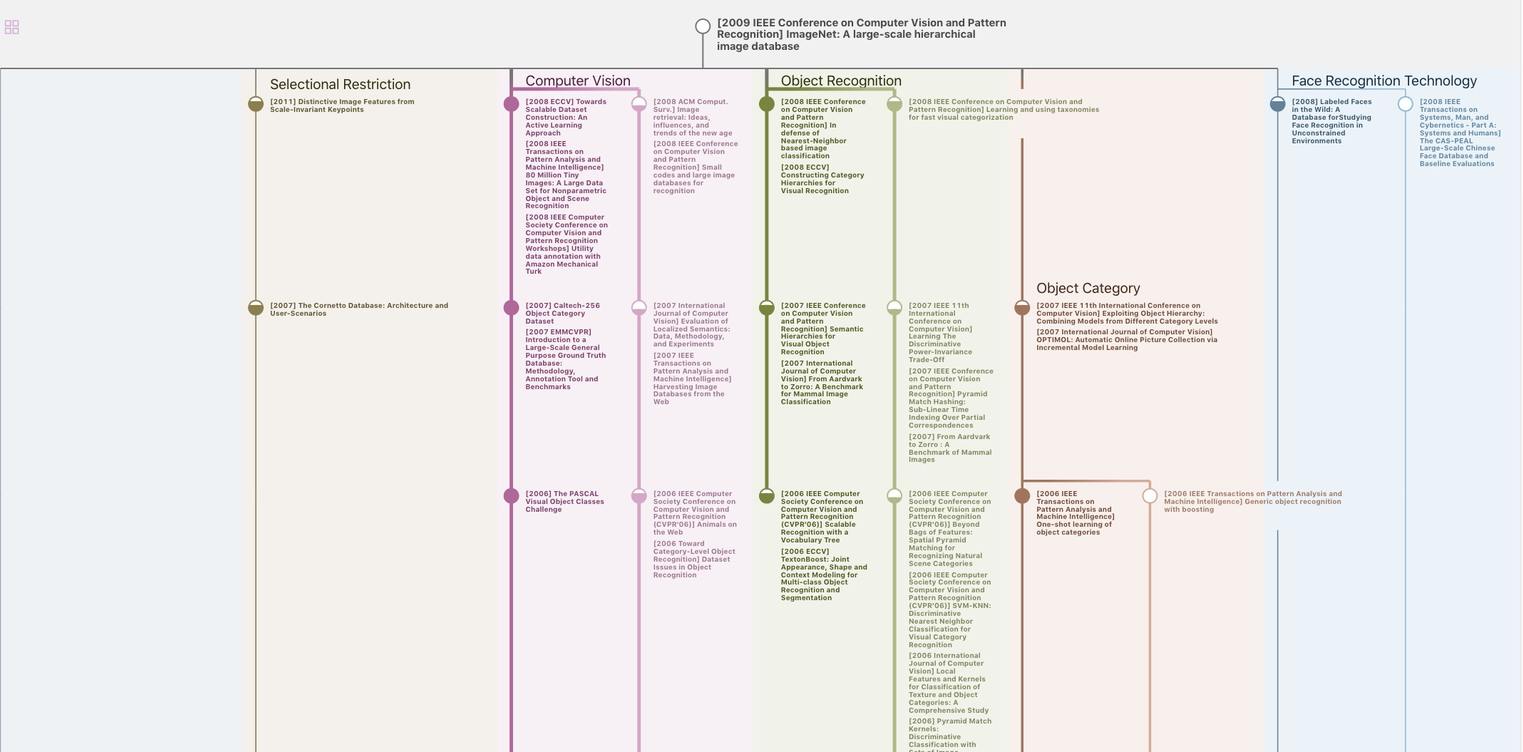
生成溯源树,研究论文发展脉络
Chat Paper
正在生成论文摘要