Bayesian Semiparametric Model for Pathway-Based Analysis with Zero-Inflated Clinical Outcomes
Journal of Agricultural, Biological and Environmental Statistics(2016)
摘要
In this paper, we propose a semiparametric regression approach for identifying pathways related to zero-inflated clinical outcomes, where a pathway is a gene set derived from prior biological knowledge. Our approach is developed by using a Bayesian hierarchical framework. We model the pathway effect nonparametrically into a zero-inflated Poisson hierarchical regression model with an unknown link function. Nonparametric pathway effect was estimated via a kernel machine, and the unknown link function was estimated by transforming a mixture of the beta cumulative density function. Our approach provides flexible nonparametric settings to describe the complicated association between gene expressions and zero-inflated clinical outcomes. The Metropolis-within-Gibbs sampling algorithm and Bayes factor were adopted to make statistical inferences. Our simulation results support that our semiparametric approach is more accurate and flexible than zero-inflated Poisson regression with the canonical link function, which is especially true when the number of genes is large. The usefulness of our approach is demonstrated through its applications to the Canine data set from Enerson et al. (Toxicol Pathol 34:27–32, 2006 ). Our approach can also be applied to other settings where a large number of highly correlated predictors are present. Supplementary materials accompanying this paper appear on-line.
更多查看译文
关键词
Gaussian process,Marginal likelihood,Mixed model,Unknown link,Pathway based analysis,Zero-inflated poisson
AI 理解论文
溯源树
样例
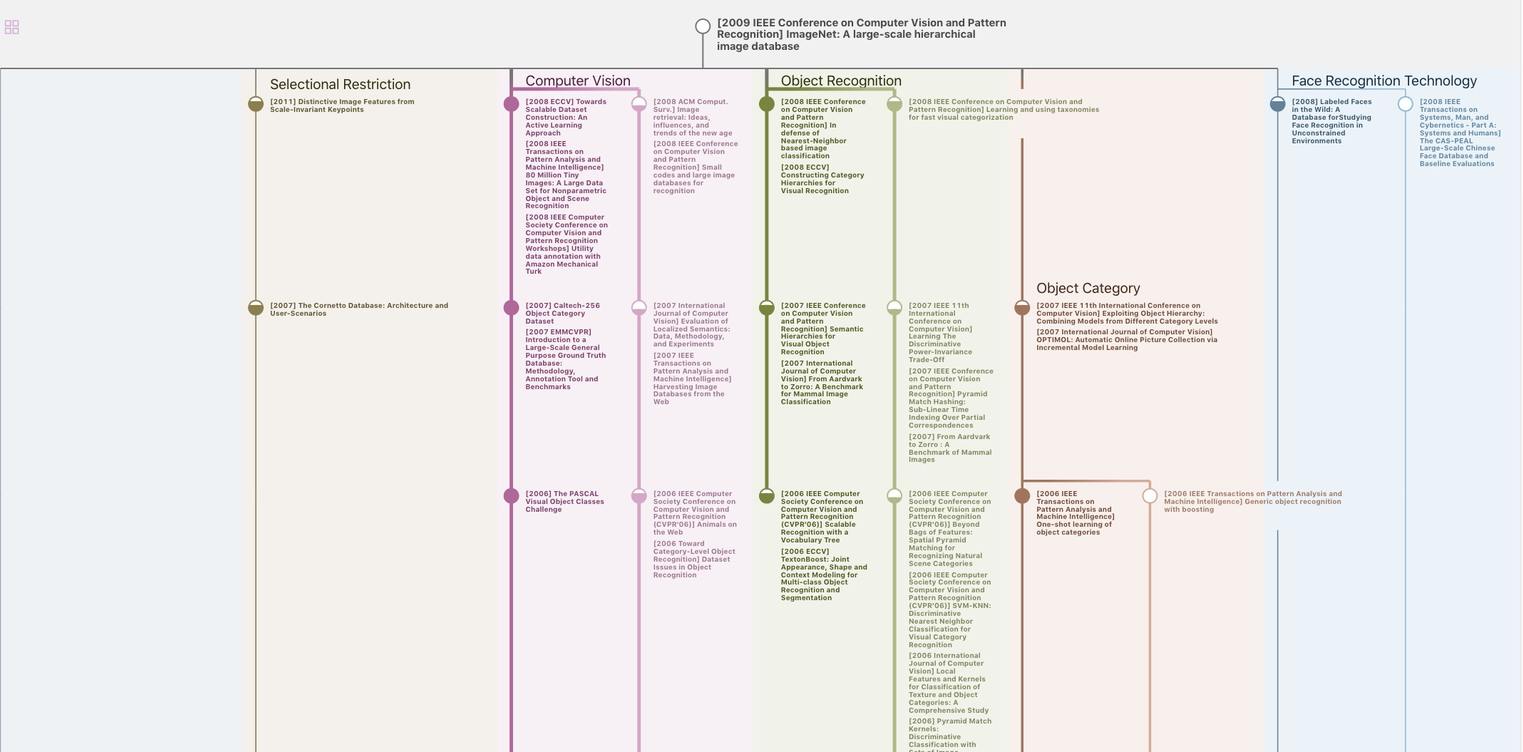
生成溯源树,研究论文发展脉络
Chat Paper
正在生成论文摘要