Information-Driven Rapidly-Exploring Random Tree for Efficient Environment Exploration
Journal of Intelligent and Robotic Systems(2017)
Abstract
Exploration of unknown environments using autonomous mobile robots is essential in various scenarios such as, for instance, search and rescue missions following natural disasters. The task consists essentially in transversing the environment to build a complete and accurate map of it, and different applications may demand different exploration strategies. In the literature, the most used strategy is a simple greedy approach which visits closest unknown sites first, without considering whether they will likely yield significant information gain about the environment. In this paper, we propose a navigation strategy for efficient exploration of unknown environments that, based on local structures present in the map built so far, uses Shannon entropy to estimate the expected information gain of exploring each candidate frontier. A key advantage of our method over the state of the art is that it allows for the robot to simultaneously (i) select a destination likely to be most informative among all candidate frontiers; and (ii) compute its own path to that destination. This unified approach balances priority among candidate frontiers with highly expected information gain and those closer to the current position of the robot. We thoroughly evaluate our methodology in several experiments in a simulated environment, showing that our approach provides faster information gain about the environment when compared to other exploration strategies.
MoreTranslated text
Key words
Autonomous exploration,Information theory,RRT,Mobile robots
AI Read Science
Must-Reading Tree
Example
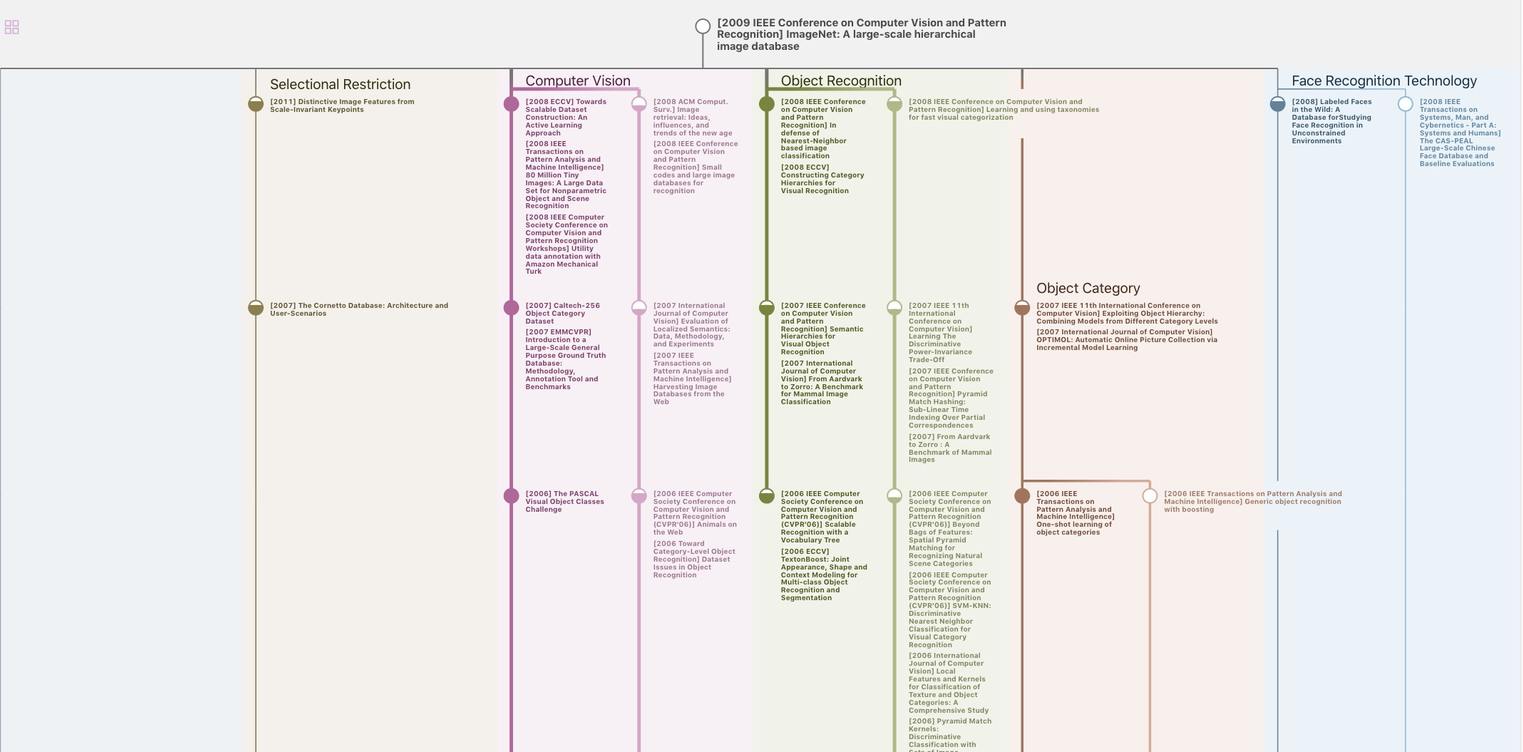
Generate MRT to find the research sequence of this paper
Chat Paper
Summary is being generated by the instructions you defined